HiCD: Change Detection in Quality-Varied Images via Hierarchical Correlation Distillation
IEEE Transactions on Geoscience and Remote Sensing(2024)
摘要
Advanced change detection techniques primarily target image pairs of equal and high quality. However, variations in imaging conditions and platforms frequently lead to image pairs with distinct qualities: one image being high-quality, while the other being low-quality. These disparities in image quality present significant challenges for understanding image pairs semantically and extracting change features, ultimately resulting in a notable decline in performance. To tackle this challenge, we introduce an innovative training strategy grounded in knowledge distillation. The core idea revolves around leveraging task knowledge acquired from high-quality image pairs to guide the model's learning process when dealing with image pairs that exhibit differences in quality. Additionally, we develop a hierarchical correlation distillation approach (involving self-correlation, cross-correlation, and global correlation). This approach compels the student model to replicate the correlations inherent in the teacher model, rather than focusing solely on individual features. This ensures effective knowledge transfer while maintaining the student model's training flexibility.
更多查看译文
关键词
Change detection,quality difference,hierarchical correlation distillation
AI 理解论文
溯源树
样例
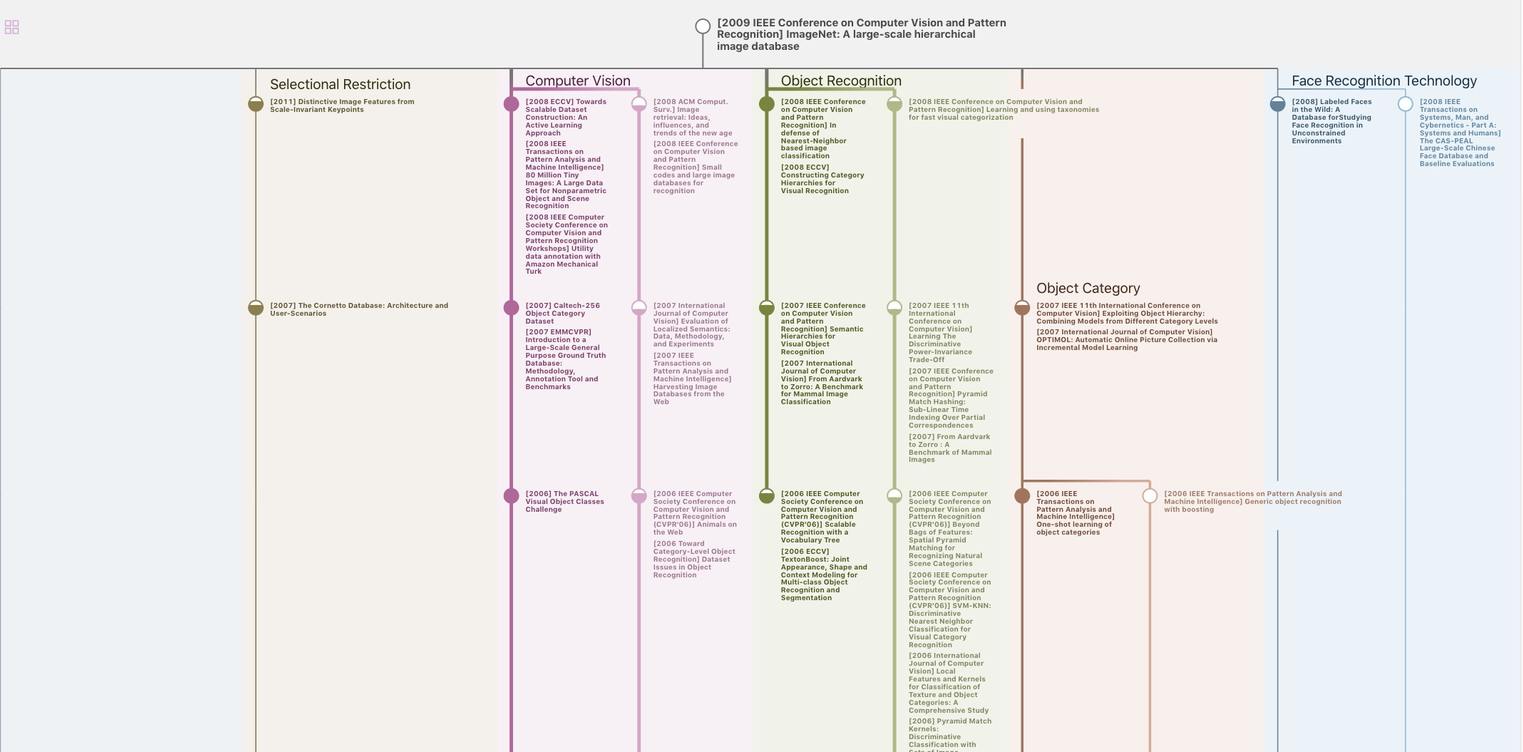
生成溯源树,研究论文发展脉络
Chat Paper
正在生成论文摘要