Knowledge-Assisted Dual-Stage Evolutionary Optimization of Large-Scale Crude Oil Scheduling
IEEE TRANSACTIONS ON EMERGING TOPICS IN COMPUTATIONAL INTELLIGENCE(2024)
摘要
With the scaling up of crude oil scheduling in modern refineries, large-scale crude oil scheduling problems (LSCOSPs) emerge with thousands of binary variables and non-linear constraints, which are challenging to be optimized by traditional optimization methods. To solve LSCOSPs, we take the practical crude oil scheduling from a marine-access refinery as an example and start with modeling LSCOSPs from crude unloading, transportation, crude distillation unit processing, and inventory management of intermediate products. On the basis of the proposed model, a dual-stage evolutionary algorithm driven by heuristic rules (denoted by DSEA/HR) is developed, where the dual-stage search mechanism consists of global search and local refinement. In the global search stage, we devise several heuristic rules based on the empirical operating knowledge to generate a well-performing initial population and accelerate convergence in the mixed variables space. In the local refinement stage, a repair strategy is proposed to move the infeasible solutions towards feasible regions by further optimizing the local continuous variables. During the whole evolutionary process, the proposed dual-stage framework plays a crucial role in balancing exploration and exploitation. Experimental results have shown that DSEA/HR outperforms the state-of-the-art and widely-used mathematical programming methods and metaheuristic algorithms on LSCOSP instances within a reasonable time.
更多查看译文
关键词
Evolutionary optimization,heuristic rules,large-scale crude oil scheduling,local refinement
AI 理解论文
溯源树
样例
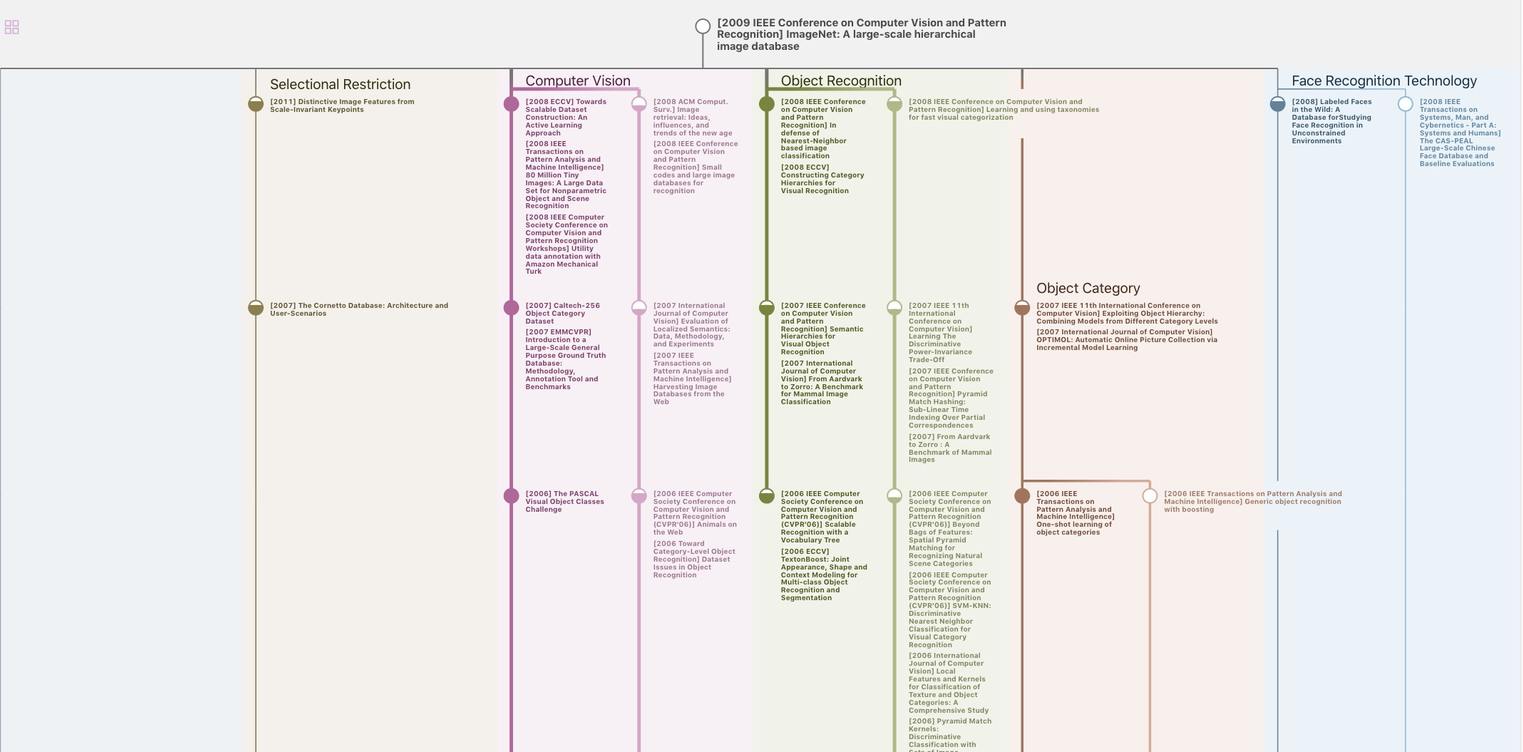
生成溯源树,研究论文发展脉络
Chat Paper
正在生成论文摘要