Deep Learning for Automatic Volumetric Segmentation of Left Ventricular Myocardium and Ischemic Scar from Multi-Slice LGE-CMR.
European heart journal. Cardiovascular Imaging(2024)
摘要
PURPOSE:This study details application of deep learning for automatic volumetric segmentation of left ventricular myocardium and scar and automated quantification of myocardial ischemic scar burden from late-gadolinium enhancement cardiovascular magnetic resonance (LGE-CMR).
MATERIALS AND METHODS:We included 501 images and manual segmentations of short-axis LGE-CMR from over 20 multinational sites, from which 377 studies were used for training and 124 studies from unique participants for internal validation. A third test set of 52 images was used for external evaluation. Three models, U-Net, Cascaded U-Net, and U-Net++, were trained with a novel adaptive weighted categorical cross entropy loss function. Model performance was evaluated using concordance correlation coefficients (CCC) for left ventricular (LV) mass and percent myocardial scar burden.
RESULTS:Cascaded U-Net was found to be the best model for quantification of LV mass and scar percentage. The model exhibited a mean difference of -5 ± 23 g for LV mass, -0.4 ± 11.2 g for scar mass, and -0.8 ± 7% for percent scar. CCC were 0.87, 0.77, and 0.78 for LV mass, scar mass, and percent scar burden, respectively, in the internal validation set and 0.75, 0.71, and 0.69, respectively, in the external test set. For segmental scar mass, CCC was 0.74 for apical scar, 0.91 for midventricular scar, and 0.73 for basal scar, demonstrating moderate to strong agreement.
CONCLUSION:We successfully trained a convolutional neural network for volumetric segmentation and analysis of left ventricular scar burden from LGE-CMR images in a large, multinational cohort of participants with ischemic scar.
更多查看译文
AI 理解论文
溯源树
样例
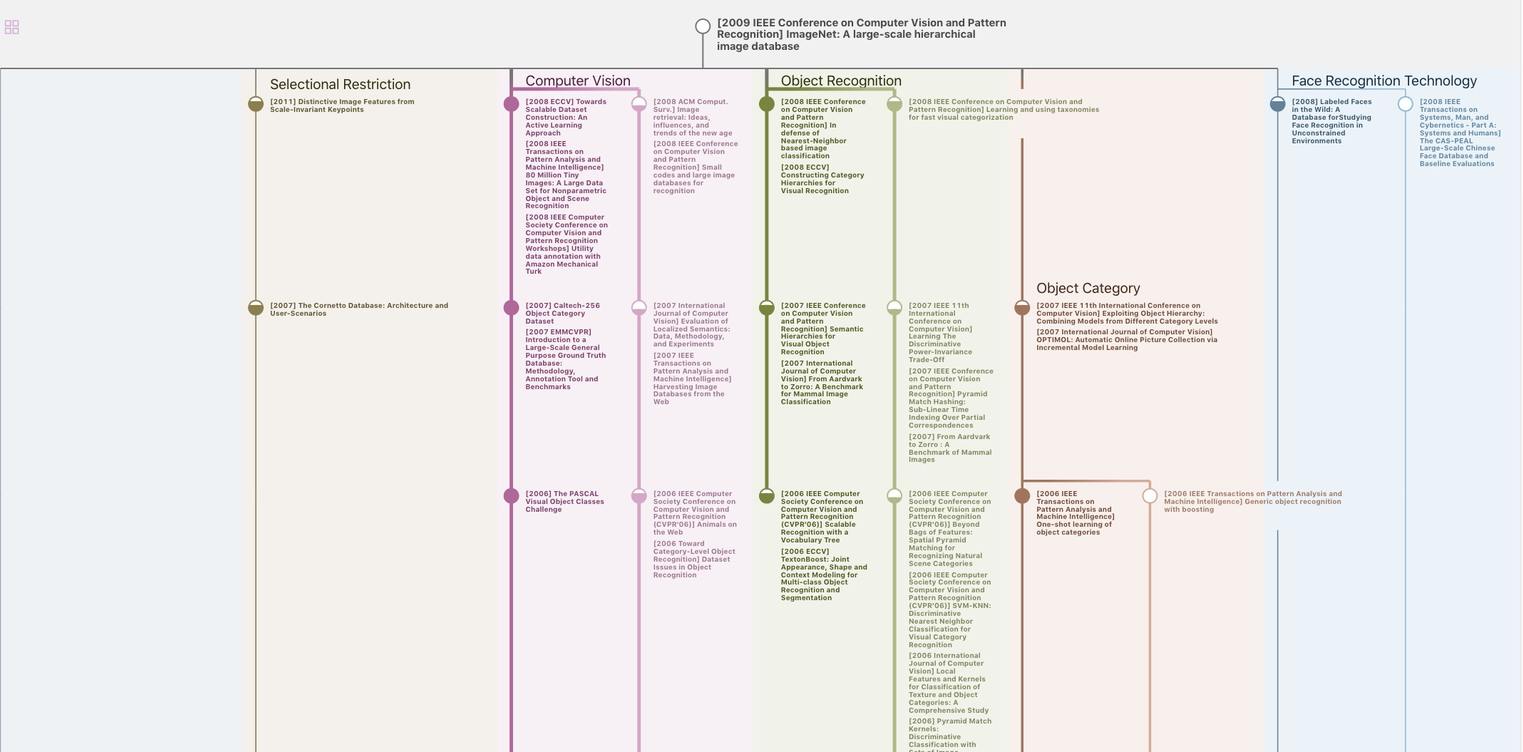
生成溯源树,研究论文发展脉络
Chat Paper
正在生成论文摘要