GPU Database Systems Characterization and Optimization
PROCEEDINGS OF THE VLDB ENDOWMENT(2023)
摘要
GPUs offer massive parallelism and high-bandwidth memory access, making them an attractive option for accelerating data analytics in database systems. However, while modern GPUs possess more resources than ever before (e.g., higher DRAM bandwidth), efficient system implementations and judicious resource allocations for query processing are still necessary for optimal performance. Database systems can save GPU runtime costs through just-enough resource allocation or improve query throughput with concurrent query processing by leveraging new GPU resource-allocation capabilities, such as Multi-Instance GPU (MIG). In this paper, we do a cross-stack performance and resource-utilization analysis of four GPU database systems, including Crystal (the state-of-the-art GPU database, performance-wise) and TQP (the latest entry in the GPU database space). We evaluate the bottlenecks of each system through an in-depth microarchitectural study and identify resource underutilization by leveraging the classic roofline model. Based on the insights gained from our investigation, we propose optimizations for both system implementation and resource allocation, using which we are able to achieve 1.9x lower latency for single-query execution and up to 6.5x throughput improvement for concurrent query execution.
更多查看译文
AI 理解论文
溯源树
样例
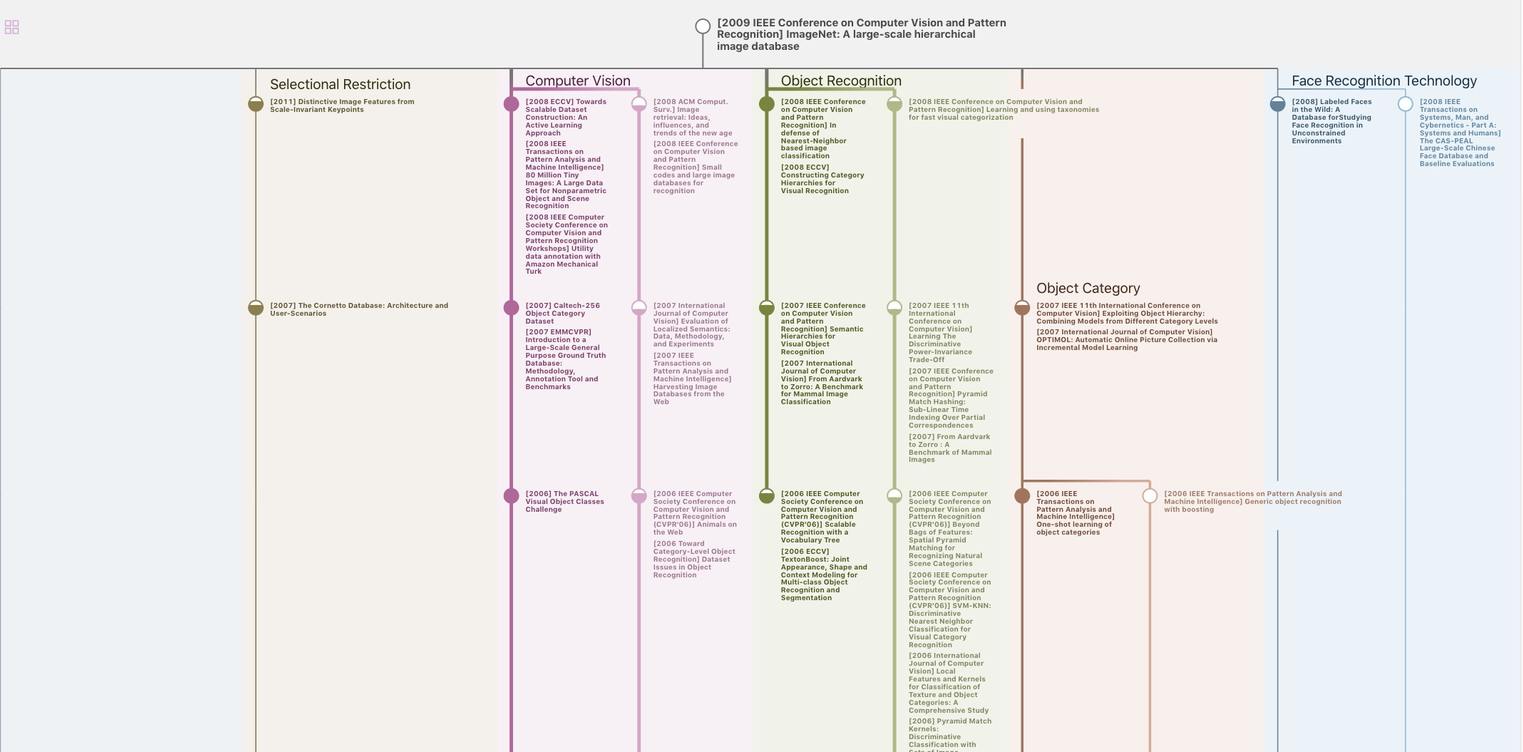
生成溯源树,研究论文发展脉络
Chat Paper
正在生成论文摘要