Uncertainty Quantification for Learning-based MPC using Weighted Conformal Prediction
2023 62ND IEEE CONFERENCE ON DECISION AND CONTROL, CDC(2023)
摘要
Nonlinear model predictive control (MPC) is an established control framework that not only provides a systematic way to handle state and input constraints, but also offers the flexibility to incorporate data-driven models. With the proliferation of machine learning techniques, there is an uptrend in the development of learning-based MPC, with neural networks (NN) being an important cornerstone. Although it has been shown that NNs are expressive enough to model the dynamics of complex systems and produce accurate state predictions, these predictions often do not include uncertainty estimates or have practical finite sample guarantees. In contrast to existing work that either requires the data samples to be exchangeable or relies on properties of the underlying data distribution, we propose an approach that utilizes weighted conformal prediction to alleviate these assumptions and to synthesize provably valid, finite-sample uncertainty estimates for data-driven dynamics models, in a distribution-free manner. These uncertainty estimates are generated online and incorporated into a novel uncertainty-aware learning-based MPC framework. Through a case study with a cartpole system controlled by a state-of-the-art learning-based MPC framework, we demonstrate that our approach not only provides wellcalibrated uncertainty estimates, but also enhances the closedloop performance of the system.
更多查看译文
AI 理解论文
溯源树
样例
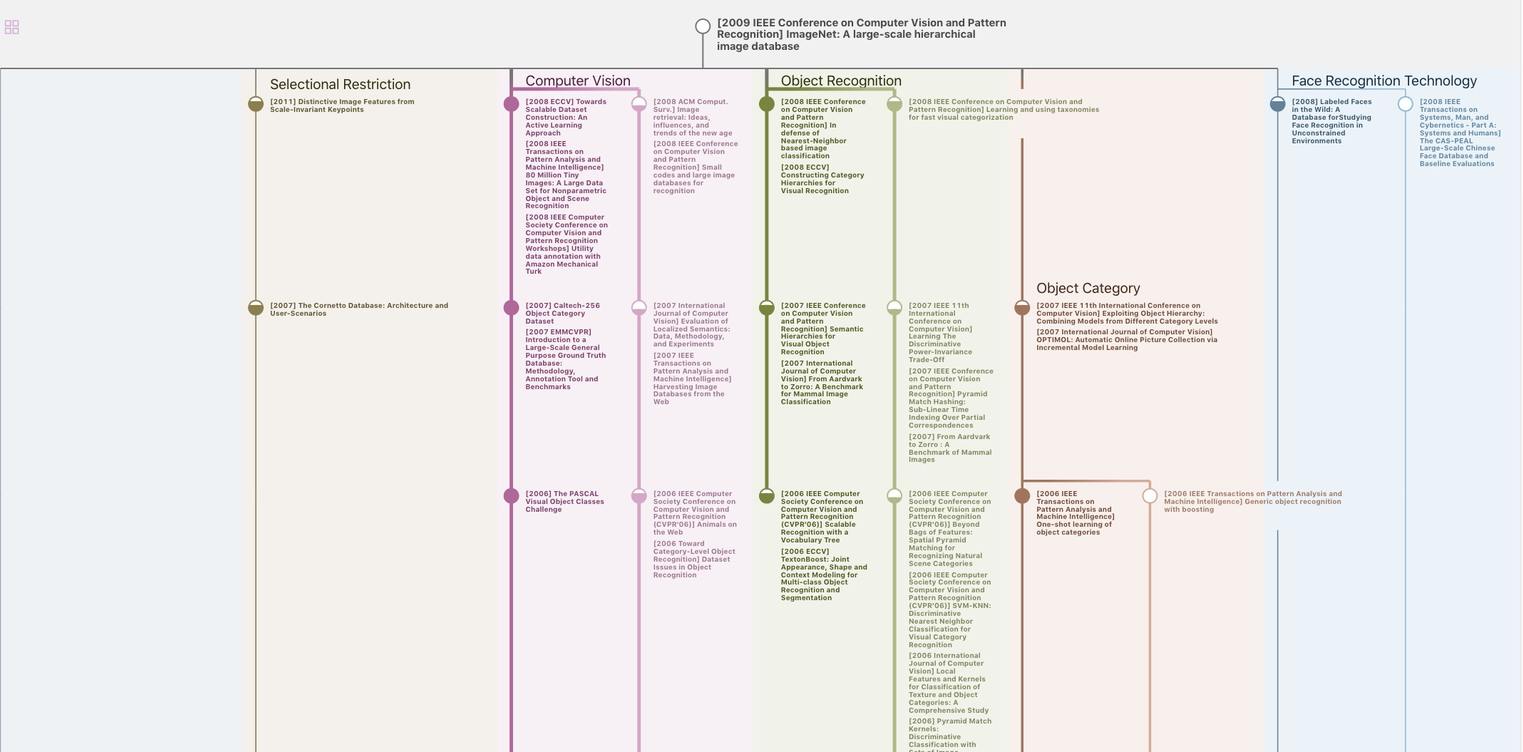
生成溯源树,研究论文发展脉络
Chat Paper
正在生成论文摘要