On-Policy Data-Driven Linear Quadratic Regulator via Model Reference Adaptive Reinforcement Learning
2023 62ND IEEE CONFERENCE ON DECISION AND CONTROL, CDC(2023)
摘要
In this paper, we address a data-driven linear quadratic optimal control problem in which the regulator design is performed on-policy by resorting to approaches from reinforcement learning and model reference adaptive control. In particular, a continuous-time identifier of the value function is used to generate online a reference model for the adaptive stabilizer. By introducing a suitably selected dithering signal, the resulting policy is shown to achieve asymptotic convergence to the optimal gain while the controlled plant reaches asymptotically the behavior of the optimal closed-loop system.
更多查看译文
AI 理解论文
溯源树
样例
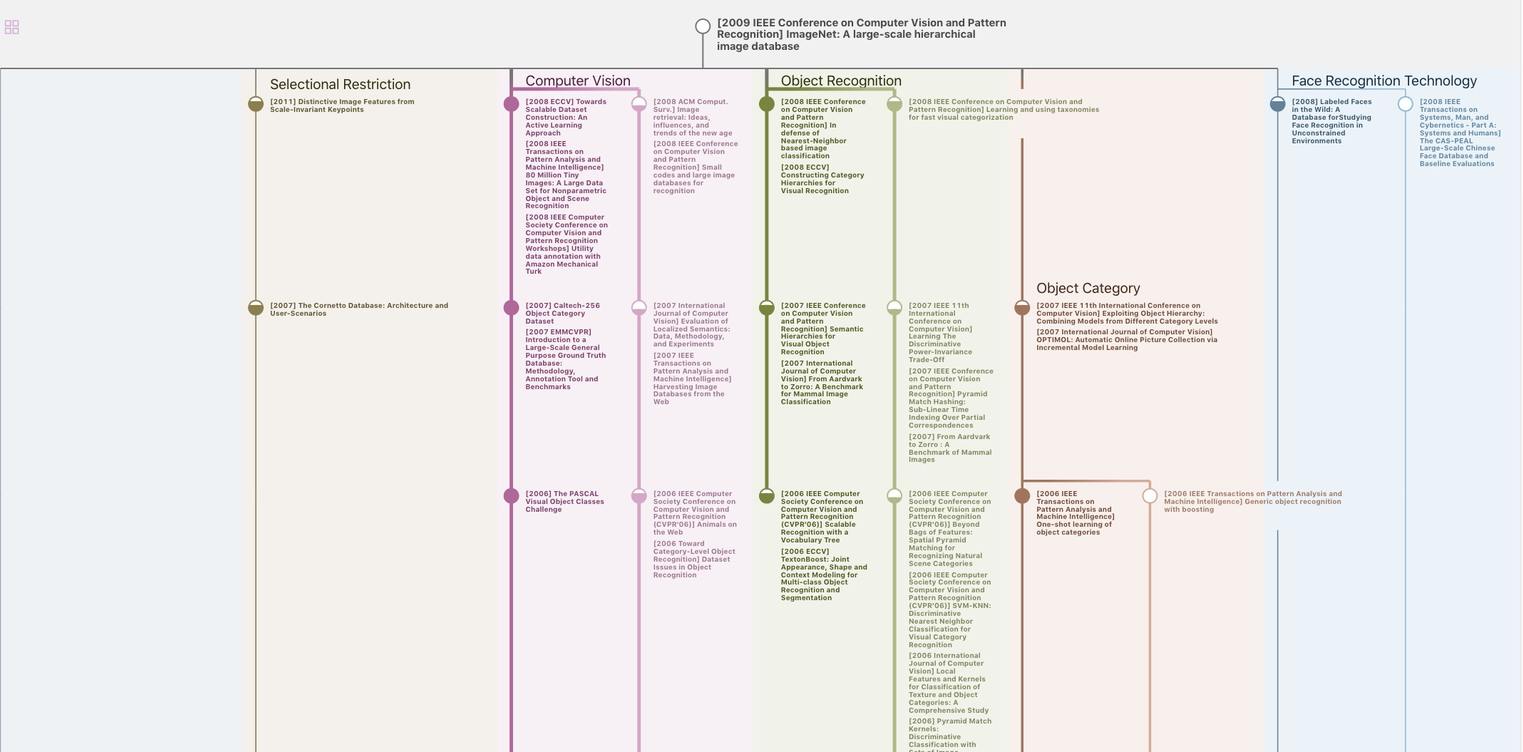
生成溯源树,研究论文发展脉络
Chat Paper
正在生成论文摘要