CrossU-Net: Dual-modality cross-attention U-Net for segmentation of precancerous lesions in gastric cancer
COMPUTERIZED MEDICAL IMAGING AND GRAPHICS(2024)
摘要
Gastric precancerous lesions (GPL) significantly elevate the risk of gastric cancer, and precise diagnosis and timely intervention are critical for patient survival. Due to the elusive pathological features of precancerous lesions, the early detection rate is less than 10%, which hinders lesion localization and diagnosis. In this paper, we provide a GPL pathological dataset and propose a novel method for improving the segmentation accuracy on a limited-scale dataset, namely RGB and Hyperspectral dual-modal pathological image Cross-attention U-Net (CrossU-Net). Specifically, we present a self-supervised pre-training model for hyperspectral images to serve downstream segmentation tasks. Secondly, we design a dual-stream U-Net-based network to extract features from different modal images. To promote information exchange between spatial information in RGB images and spectral information in hyperspectral images, we customize the cross-attention mechanism between the two networks. Furthermore, we use an intermediate agent in this mechanism to improve computational efficiency. Finally, we add a distillation loss to align predicted results for both branches, improving network generalization. Experimental results show that our CrossU-Net achieves accuracy and Dice of 96.53% and 91.62%, respectively, for GPL lesion segmentation, providing a promising spectral research approach for the localization and subsequent quantitative analysis of pathological features in early diagnosis.
更多查看译文
关键词
Dual -modality,Hyperspectral imaging,Cross attention,Image segmentation
AI 理解论文
溯源树
样例
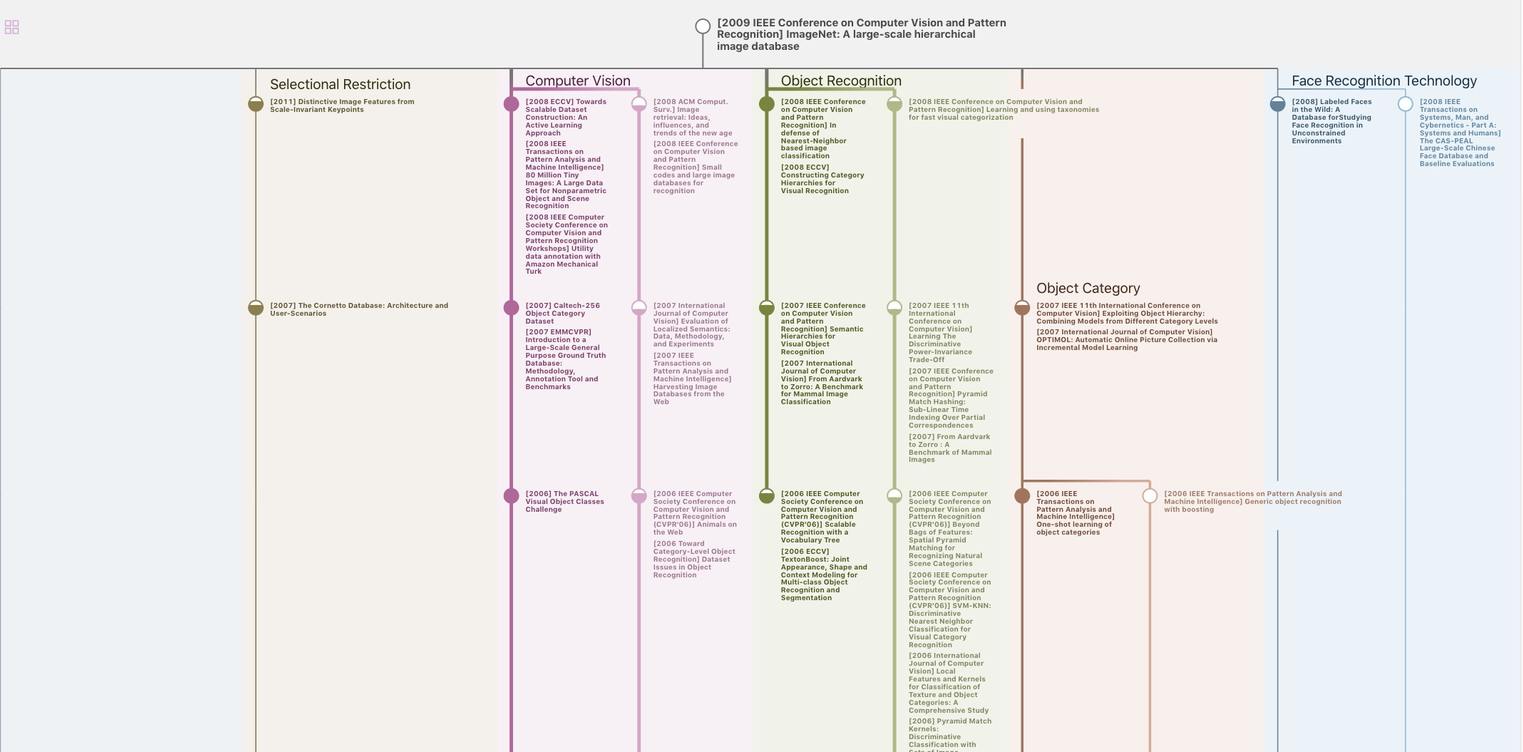
生成溯源树,研究论文发展脉络
Chat Paper
正在生成论文摘要