Divide and Conquer: Improving Multi-Camera 3D Perception With 2D Semantic-Depth Priors and Input-Dependent Queries
IEEE TRANSACTIONS ON IMAGE PROCESSING(2024)
摘要
3D perception tasks, such as 3D object detection and Bird's-Eye-View (BEV) segmentation using multi-camera images, have drawn significant attention recently. Despite the fact that accurately estimating both semantic and 3D scene layouts are crucial for this task, existing techniques often neglect the synergistic effects of semantic and depth cues, leading to the occurrence of classification and position estimation errors. Additionally, the input-independent nature of initial queries also limits the learning capacity of Transformer-based models. To tackle these challenges, we propose an input-aware Transformer framework that leverages Semantics and Depth as priors (named SDTR). Our approach involves the use of an S-D Encoder that explicitly models semantic and depth priors, thereby disentangling the learning process of object categorization and position estimation. Moreover, we introduce a Prior-guided Query Builder that incorporates the semantic prior into the initial queries of the Transformer, resulting in more effective input-aware queries. Extensive experiments on the nuScenes and Lyft benchmarks demonstrate the state-of-the-art performance of our method in both 3D object detection and BEV segmentation tasks.
更多查看译文
关键词
Three-dimensional displays,Semantics,Task analysis,Transformers,Object detection,Image segmentation,Feature extraction,3D object detection,bird's-eye-view (BEV) segmentation,multi-camera,3D perception
AI 理解论文
溯源树
样例
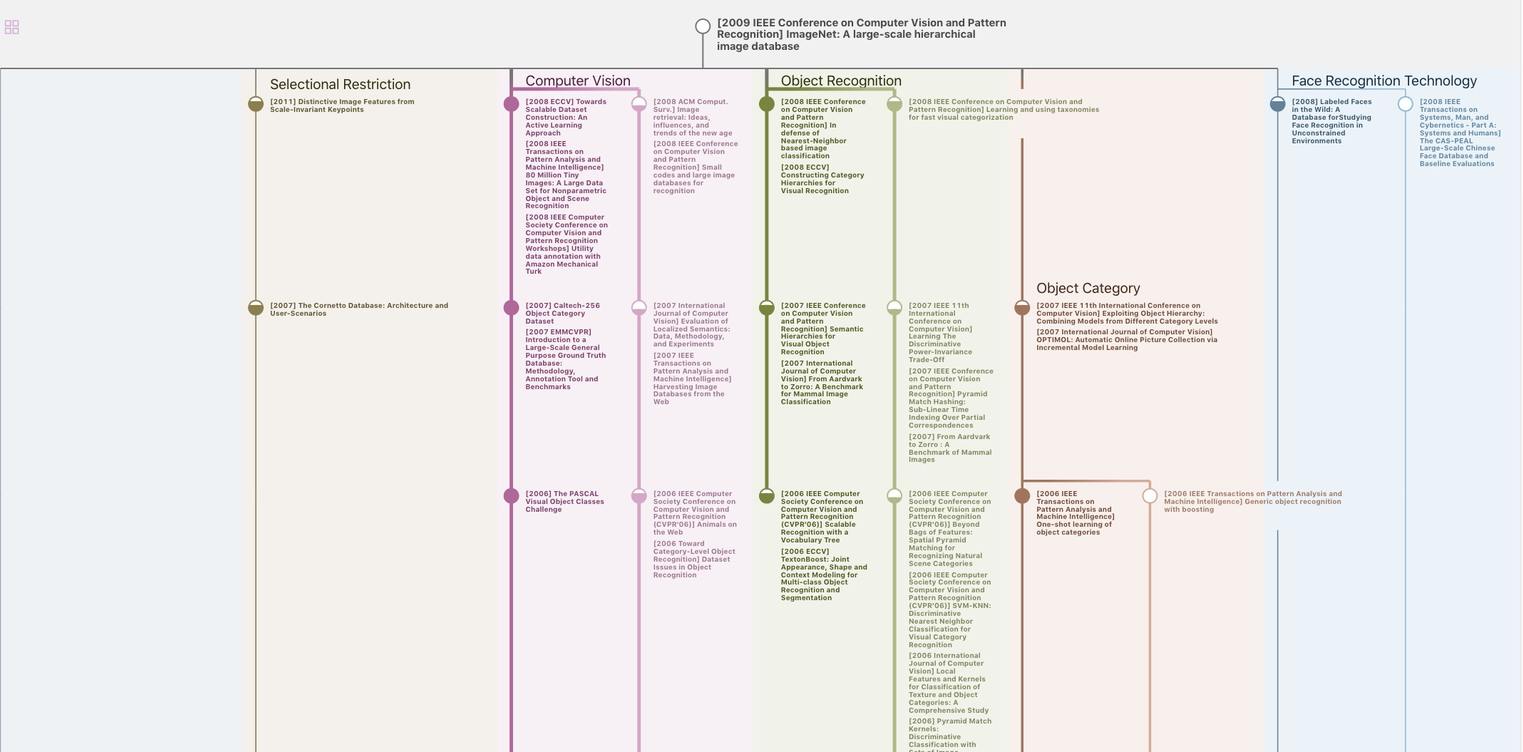
生成溯源树,研究论文发展脉络
Chat Paper
正在生成论文摘要