PEST: A General-Purpose Protein Embedding Model for Homology Search.
2023 IEEE International Conference on Bioinformatics and Biomedicine (BIBM)(2023)
摘要
Finding known homologs of newly predicted proteins is essential for understanding their functions and mechanisms. It is a highly complex task because proteins undergo various changes during evolution. Traditional methods based on sequence or structure alignment either have low accuracy or take a long time. Recent deep learning-based methods primarily focus on structural information, yet they can’t fully exploiting protein information. To solve this problem, in this paper, we propose a novel general-purpose protein embedding model that can be used for homology search. It first employs a protein language pre-trained model to extract protein sequence embeddings, capturing intricate biological patterns. Subsequently, a Transformer integrating protein structural information generates the high-level representations. By combining protein sequence and structural features, the model can effectively exploit the rich contextual and spatial information inherent in proteins. We applied the model to the SCOP dataset for protein superfamily classification, achieving a classification accuracy of 86.97%, outperforming state-of-the-art method by 7.91%. The source code has been published on GitHub (https://github.com/CMACH508/PEST).
更多查看译文
关键词
protein embedding,alignment-free homology search,superfamily classification,deep representation learning
AI 理解论文
溯源树
样例
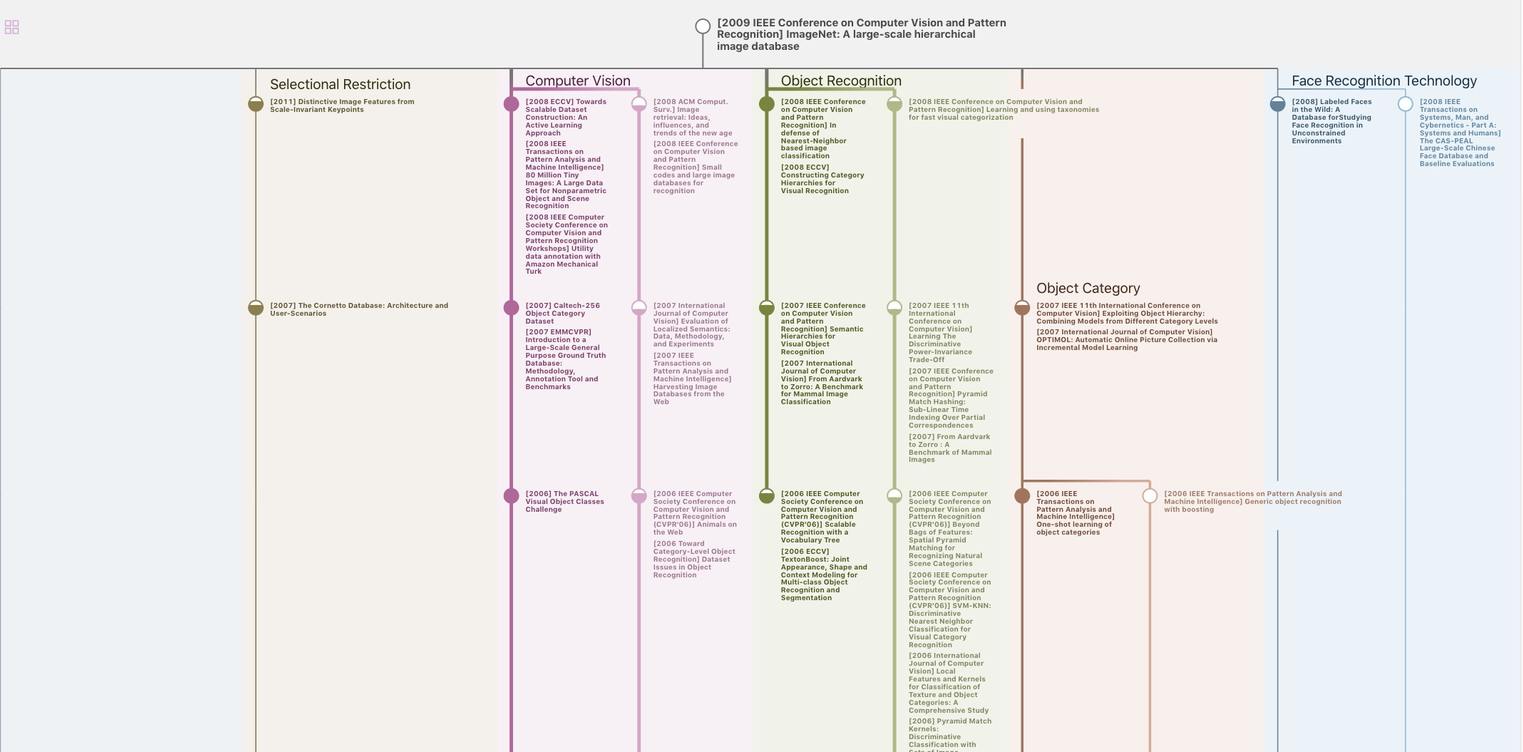
生成溯源树,研究论文发展脉络
Chat Paper
正在生成论文摘要