Dual-branch Feature Interaction Network with Structure Information Learning for Retinopathy of Prematurity Classification.
2023 IEEE International Conference on Bioinformatics and Biomedicine (BIBM)(2023)
摘要
Diagnosing retinopathy of prematurity (ROP) is a time-consuming and complex task, even for experienced clinicians, as it is challenging to determine its specific stages accurately. In this study, we propose an advanced dual-branch feature interaction network for predicting the stages of ROP using color fundus photographs. Specifically, the proposed network includes a Vision Transformer (ViT) branch and a convolutional neural network (CNN) branch, which are used to capture global contextual information and express local detail features, respectively. To improve the efficiency of ViT, we introduce a cascaded group attention (CGA) module feeding attention heads with different splits of the full feature, which not only saves computation cost but also improves attention diversity. The semantic features extracted from both the Transformer and CNN branches are fused through the branch feature interaction (BFI) module, allowing us to leverage the unique characteristics of both branches to optimize ROP feature representations comprehensively. Afterwards, we further design a Transformer block with structure information learning (SIL) to gather ROP-related semantic information from high-level features, gradually constructing ROP feature information structure to highlight important regions and improve the model’s discriminative ability for different lesion feature structures. Our extensive experiments on both clinical and public datasets produce promising results, showcasing the outstanding performance of our method.
更多查看译文
关键词
ROP diagnosis,dual-branch,feature interaction,structure information learning
AI 理解论文
溯源树
样例
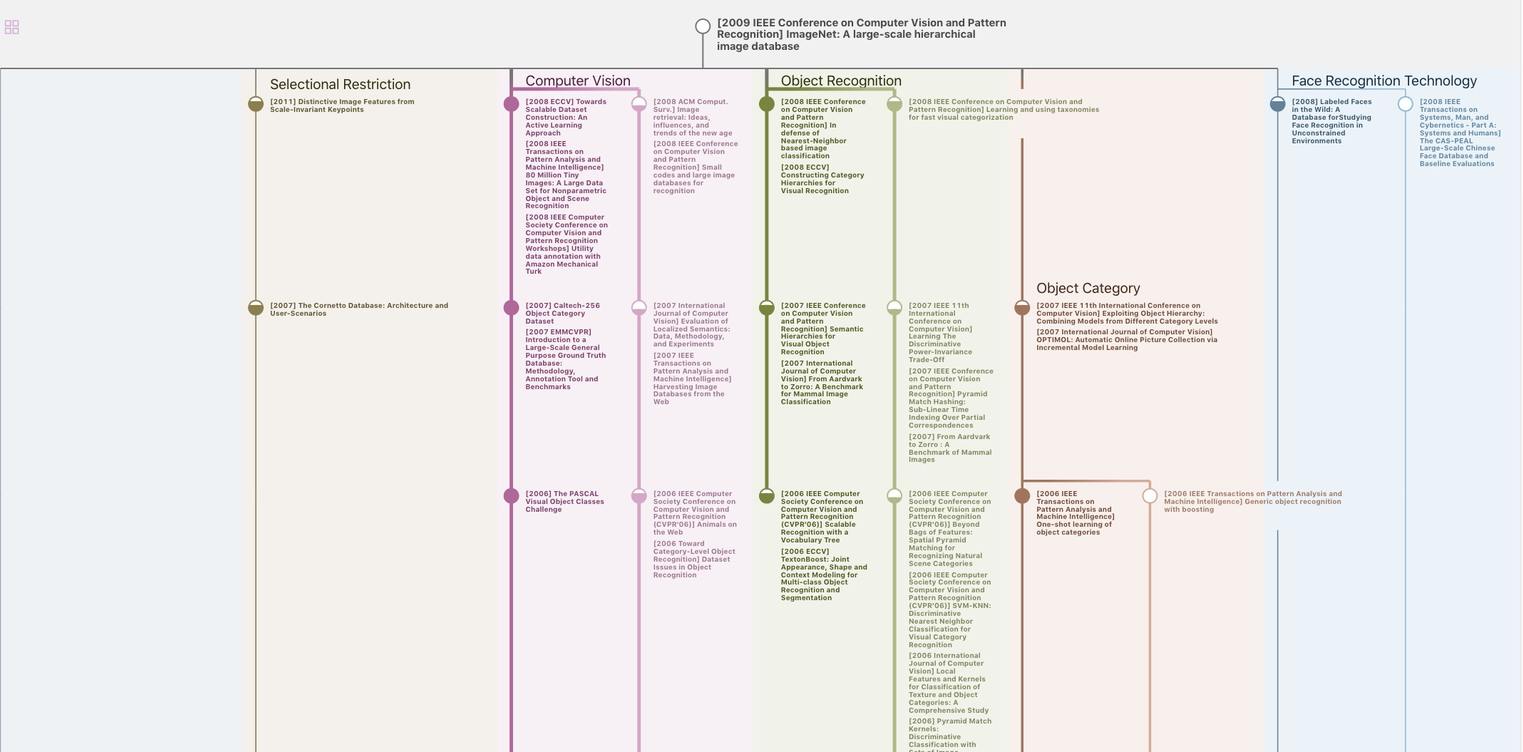
生成溯源树,研究论文发展脉络
Chat Paper
正在生成论文摘要