sEMG-Based Wearable HMI System For Real-Time Robotic Arm Control With Edge AI.
2023 IEEE Biomedical Circuits and Systems Conference (BioCAS)(2023)
摘要
Gesture and motion recognition based on surface electromyographic (sEMG) signals brings intelligent and natural interaction experience for human-machine interaction (HMI). Deep learning (DL) based recognition methods are currently widely used. However, performing deep learning in real-time on resource-constrained edge portable devices is still in its infancy. Therefore, there is a great demand for efficient, accurate, cost-effective solutions for edge devices. To address this challenge, we built a low-power and wearable HMI system with edge AI. Specifically, we proposed a multi-channel sEMG signal-based gesture and action recognition algorithm, developed a portable 8-channel sEMG acquisition circuit, and designed a dedicated algorithm acceleration processor to implement the proposed algorithm. The data of 10 categories in 6 healthy subjects were tested with an average recognition accuracy of 95.73%. In addition, we built a robotic arm online test system for testing, and the experimental results showed an average success rate of 92.5%, a single-frame signal processing and recognition time of 44.36 s, and an overall system power consumption of 0.493W. This system meets the needs of real-time applications and can be integrated or extended to other application scenarios.
更多查看译文
关键词
HMI,sEMG,Robotic Arm,Hardware accelerator,Deep learning,Embedded Platforms,Wearable device
AI 理解论文
溯源树
样例
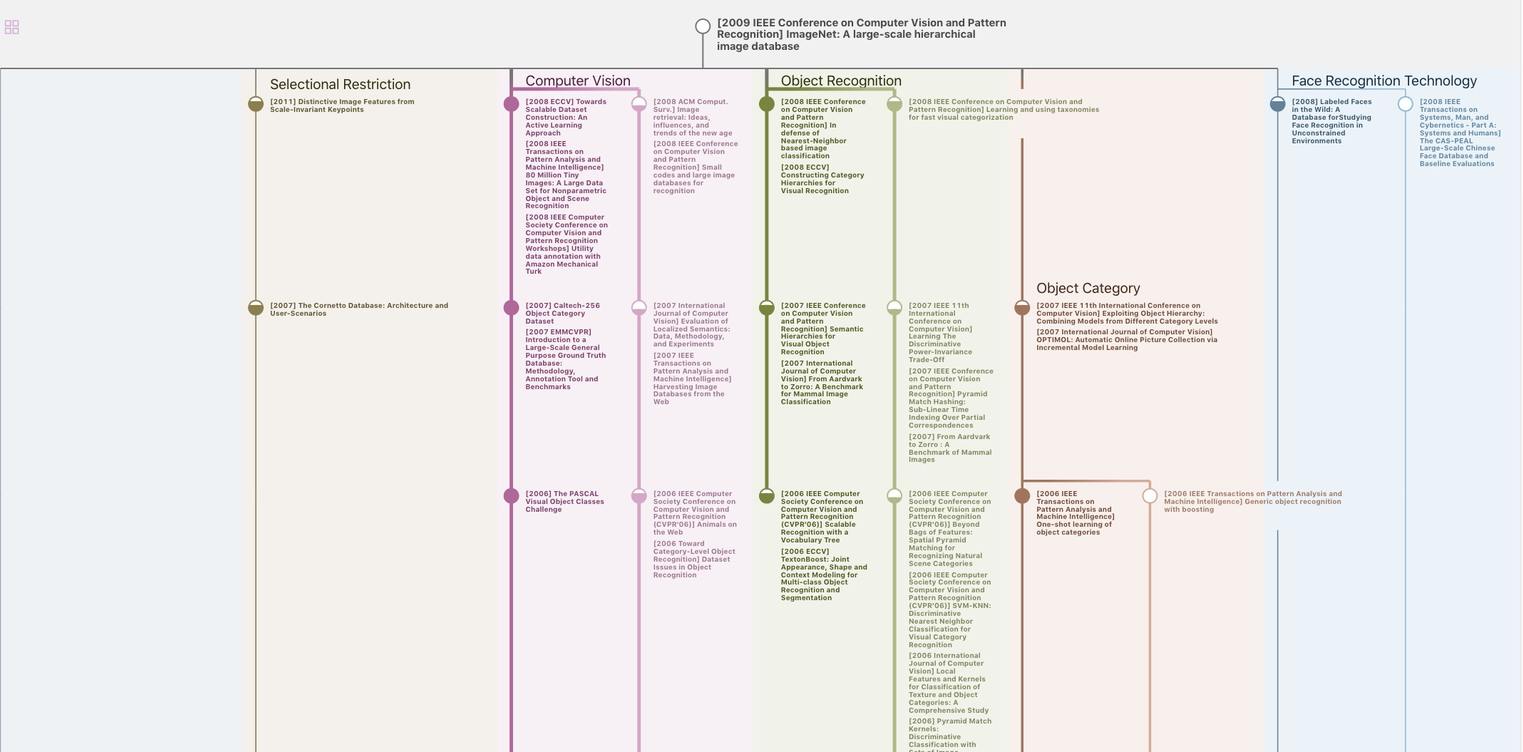
生成溯源树,研究论文发展脉络
Chat Paper
正在生成论文摘要