FedAGA: A federated learning framework for enhanced inter-client relationship learning
KNOWLEDGE-BASED SYSTEMS(2024)
摘要
Exploring relationships is important for effective model aggregation in Personalized Federated Learning (PFL). However, state-of-the-art (SOTA) PFL methods primarily focus on simple model aggregation through interclient relationships within Euclidean space, overlooking the intricate nature of unstructured relationships. To remedy this, we propose a novel framework named Federated Learning with Adaptive Graph -based Aggregation (FedAGA) tailored for non-Euclidean space. FedAGA captures inter -client relationships through similarities based on accumulated gradients of various client models, constructing a dynamic graph topology. To enhance the reliability and accuracy of graph topology, we introduce two criteria from the perspectives of model convergence and model divergence. Our proposed approach is extensively evaluated on two PFL benchmark datasets and four real medical datasets. The results demonstrate that FedAGA accurately captures inter -client relationships across diverse models, leading to superior performance compared to the SOTA baselines. In particular, we observe notable accuracy improvements of 6.8% on CIFAR10 and 4.0% on PathMNIST compared to the existing methods.
更多查看译文
关键词
Personalized federated learning,Relationship exploration,Graph-based method,Internet of Things,Distributed learning
AI 理解论文
溯源树
样例
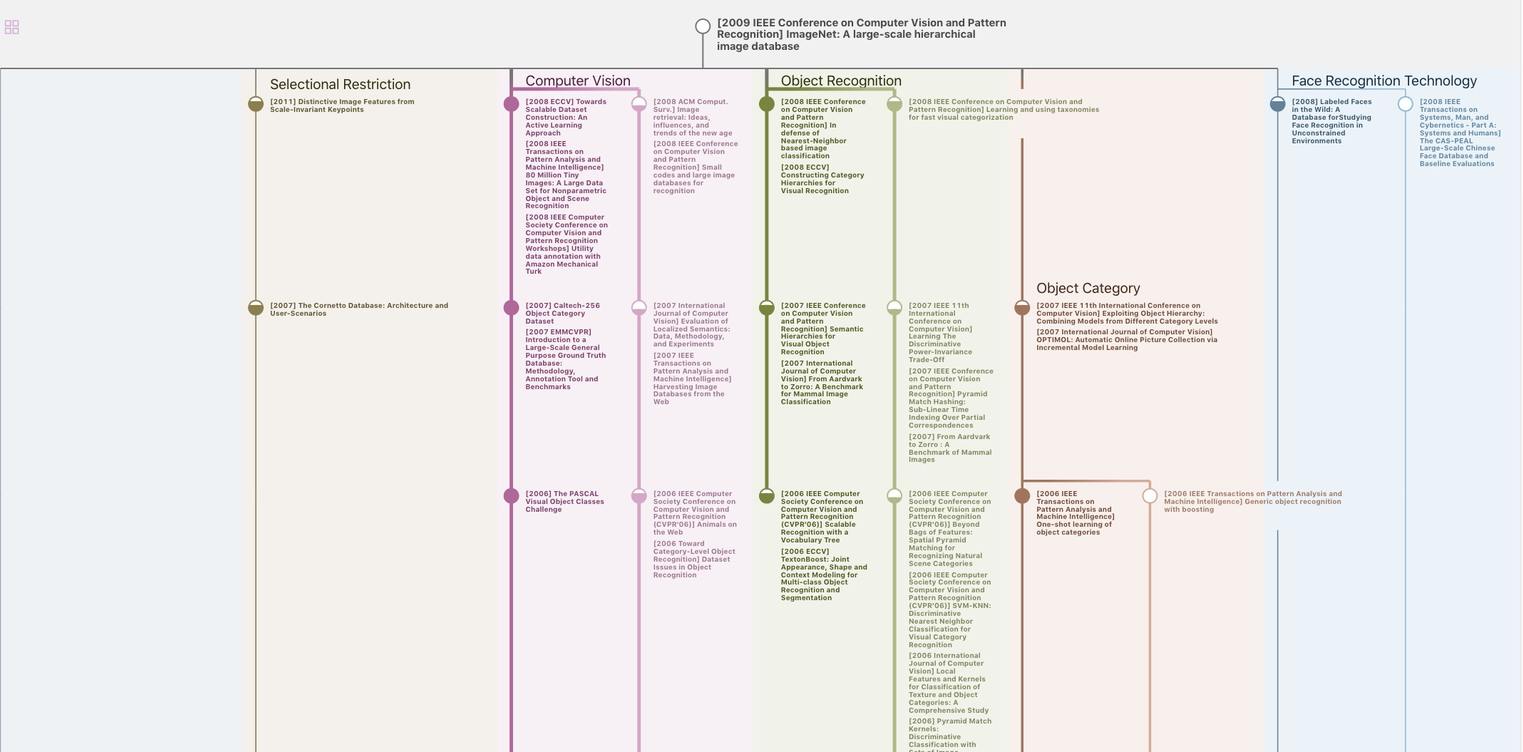
生成溯源树,研究论文发展脉络
Chat Paper
正在生成论文摘要