On-the-fly image-level oversampling for imbalanced datasets of manufacturing defects
Machine Learning(2024)
摘要
Visual defect recognition and its manufacturing applications have been an upcoming topic in recent AI research. Defect datasets are often severely imbalanced and can be additionally burdened with separating classes of high visual similarity. Although various methods of data augmentation have been proposed to mitigate the class imbalance, they often fail to cope with tinier minority classes or have fidelity issues with smaller defects while, at the same time, needing significant computational resources to train. Also, augmentation based on vector-based oversampling struggles to produce high-fidelity inputs and is hard to apply on custom CNN architectures, which often perform better for this type of problem. Our work presents an image-level oversampling method based on an instance-based image generator that can be applied to any CNN directly during the training process without increasing the order of training time required. It is based on identifying a small number of the most uncertain base samples close to the estimated class boundaries and using them as seeds for augmentation. The resulting images are of high visual quality preserving small class differences, and they also improve the classifier boundary leading to higher recall scores than other state-of-the-art approaches.
更多查看译文
关键词
Imbalanced learning,Defect recognition,Data augmentation,Oversampling,Deep learning,Generative adversarial networks
AI 理解论文
溯源树
样例
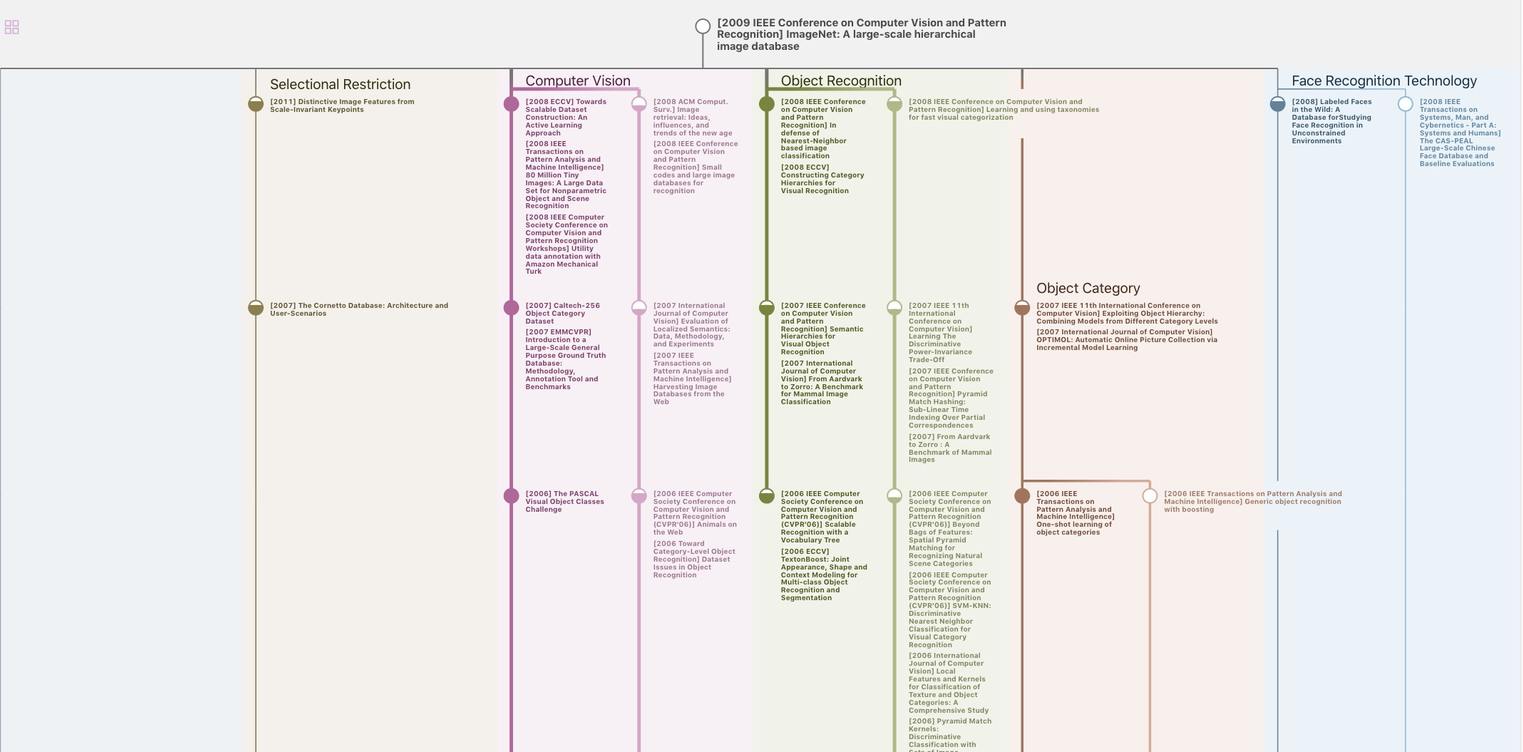
生成溯源树,研究论文发展脉络
Chat Paper
正在生成论文摘要