Communication-efficient clustered federated learning via model distance
Machine Learning(2024)
摘要
Clustered Federated Learning (CFL) leverages the differences among data distributions on clients to partition all clients into several clusters for personalized federated training. Compared with the conventional federated algorithms such as FedAvg, existing methods for CFL require either more communication costs or multi-stage computation overheads. In this paper, we propose an iterative CFL framework with almost the same communication cost as FedAvg in each round based on a novel model distance. Specifically, the model distance measures the discrepancy between the client model and the cluster model so that we can estimate the cluster identities for clients on the server side. The proposed model distance considers class-wise model dissimilarity, which enables us to apply it to multi-class classification even when the labels are non-iid across clients. To calculate the proposed model distance, we introduce two sampling methods which generate samples from feature distributions approximately without accessing the raw dataset. Experimental results show that our method can achieve superior and comparable performance on non-iid and iid data respectively with less communication cost compared with the baselines.
更多查看译文
关键词
Federated learning,Client clustering,Data heterogeneity,Model distance
AI 理解论文
溯源树
样例
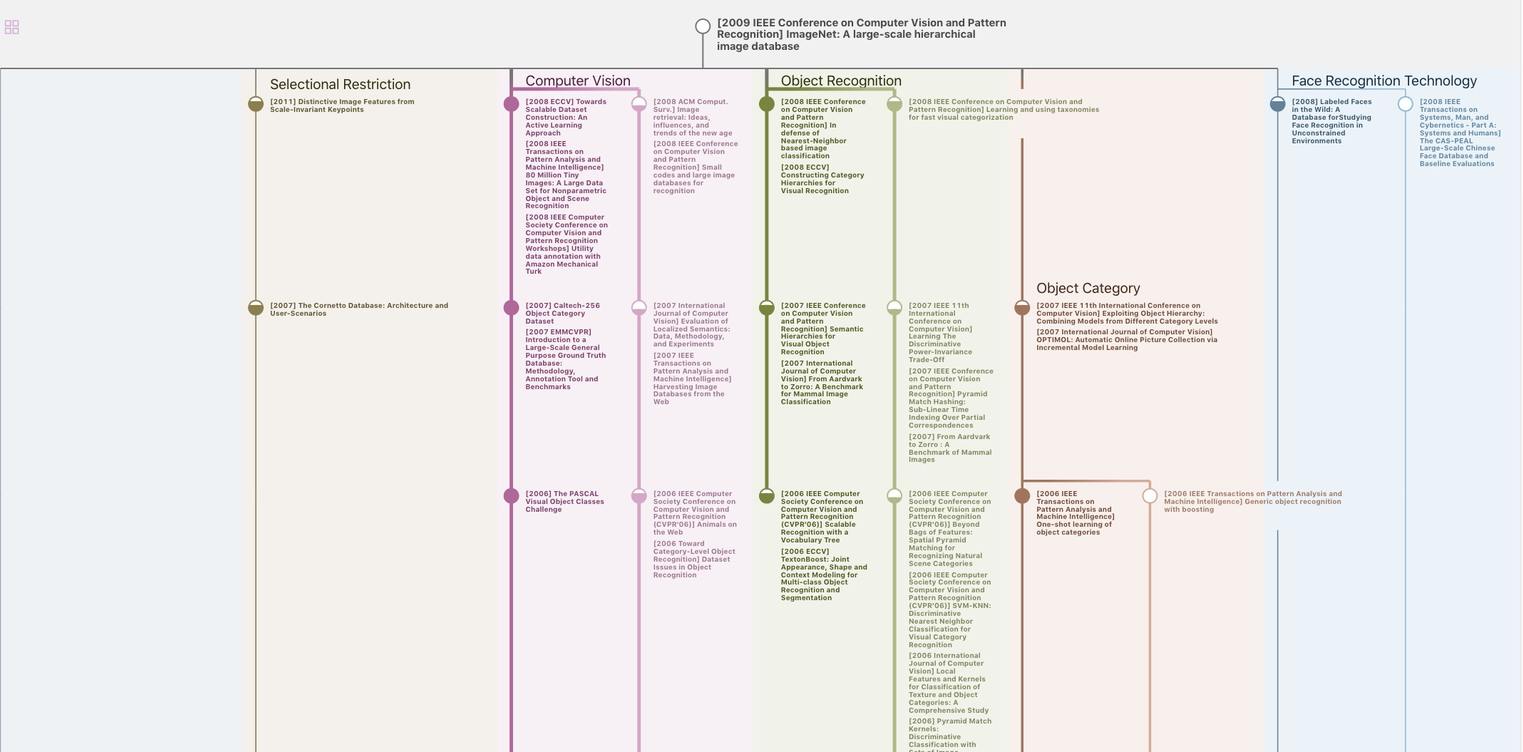
生成溯源树,研究论文发展脉络
Chat Paper
正在生成论文摘要