An Iterative Semi-supervised Approach with Pixel-wise Contrastive Loss for Road Extraction in Aerial Images
ACM TRANSACTIONS ON MULTIMEDIA COMPUTING COMMUNICATIONS AND APPLICATIONS(2024)
摘要
Extracting roads in aerial images has numerous applications in artificial intelligence and multimedia computing, including traffic pattern analysis and parking space planning. Learning deep neural networks, though very successful, demand vast amounts of high-quality annotations, of which acquisition is time-consuming and expensive. In this work, we propose a semi-supervised approach for image-based road extraction in which only a small set of labeled images are available for training to address this challenge. We design a pixel-wise contrastive loss to self-supervise the network training to utilize the large corpus of unlabeled images. The key idea is to identify pairs of overlapping image regions (positive) or non-overlapping image regions (negative) and encourage the network to make similar outputs for positive pairs or dissimilar outputs for negative pairs. We also develop a negative sampling strategy to filter false-negative samples during the process. An iterative procedure is introduced to apply the network over raw images to generate pseudo-labels, filter and select high-quality labels with the proposed contrastive loss, and retrain the network with the enlarged training dataset. We repeat these iterative steps until convergence. We validate the effectiveness of the proposed methods by performing extensive experiments on the public SpaceNet3 and DeepGlobe Road datasets. Results show that our proposed method achieves state-of-the-art results on public image segmentation benchmarks and significantly outperforms other semi-supervised methods.
更多查看译文
关键词
Deep learning,semi-supervised learning,contrastive loss,iterative labeling,road extraction
AI 理解论文
溯源树
样例
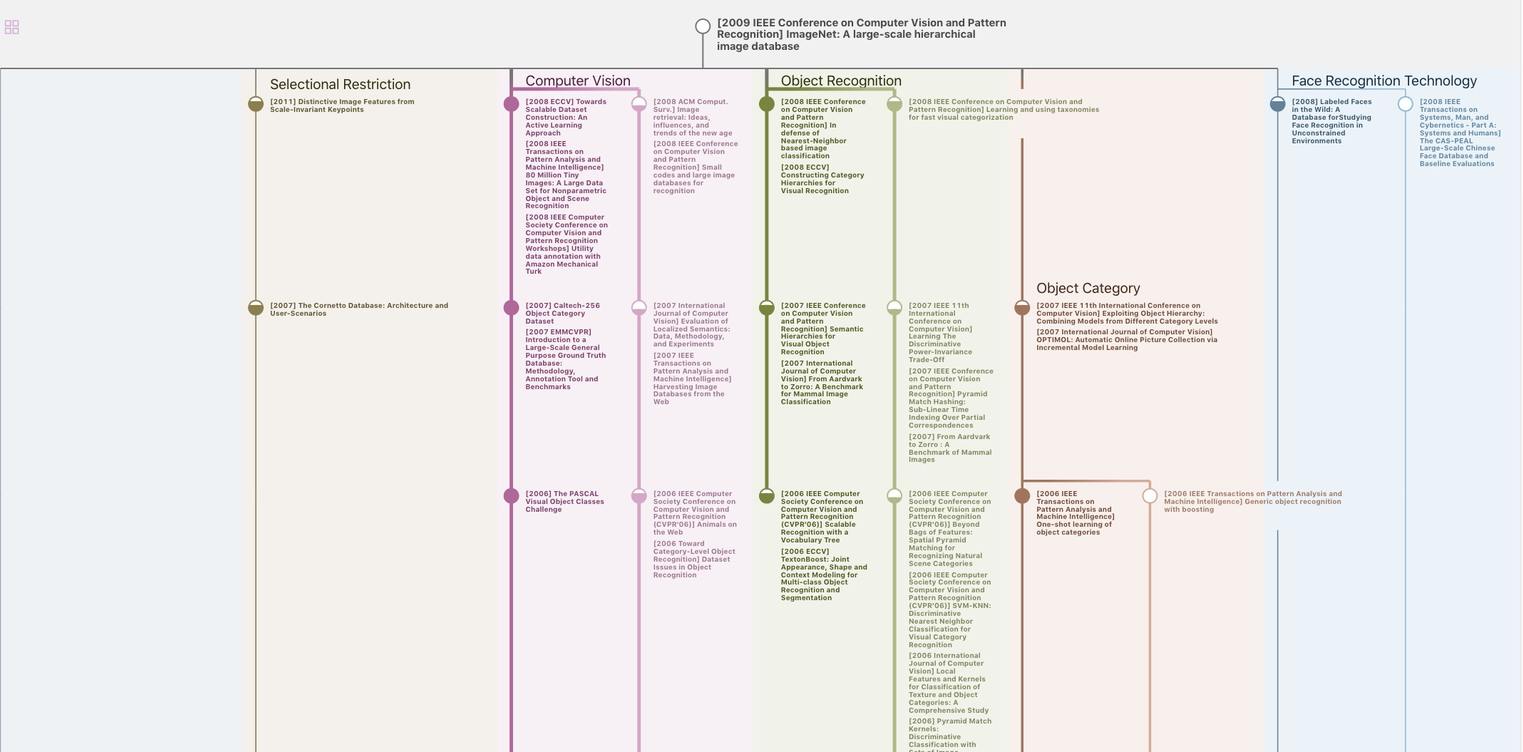
生成溯源树,研究论文发展脉络
Chat Paper
正在生成论文摘要