Shooting condition insensitive unmanned aerial vehicle object detection
Expert Systems with Applications(2024)
摘要
The increasing use of unmanned aerial vehicle (UAV) devices in diverse fields such as agriculture, surveillance, and aerial photography has led to a significant demand for intelligent object detection. The key is in dealing with unconstrained shooting condition variations (e.g., weather, view, altitude). Previous data augmentation or adversarial learning based methods try to extract shooting condition invariant features, but they are constrained by the large number of combinations of different shooting conditions. To address this limitation, in this work we introduce a novel Language Guided UAV Detection Network Training Method (LGNet), capable of leveraging pre-trained multi-modal representations (e.g., CLIP) as learning structure reference, and as a model-agnostic strategy that can be applied in various detection models. The key idea is to remove language-described domain-specific features from the visual-language feature space, enhancing tolerance to variations in shooting conditions. Concretely, we fine-tune text prompt embedding about shooting condition and feed the fine-tuned text prompt embedding into CLIP-text encoder to obtain more accurate domain-specific features. By aligning the features from the detector backbone with those of the CLIP image encoder, we situate features within a visual-language space, while staying away from language-encoded domain-specific features to be domain-invariant. Extensive experiments demonstrate that LGNet, as a generic training plug-in, boosts the state-of-the-art performance on various base detectors. Specifically, it achieves an increase in the range of 0.9–1.7% in Average Precision (AP) on the UAVDT dataset and 1.0–2.4% on the VisDrone dataset, respectively.
更多查看译文
关键词
UAV object detection,Visual-language model,Text prompt embedding
AI 理解论文
溯源树
样例
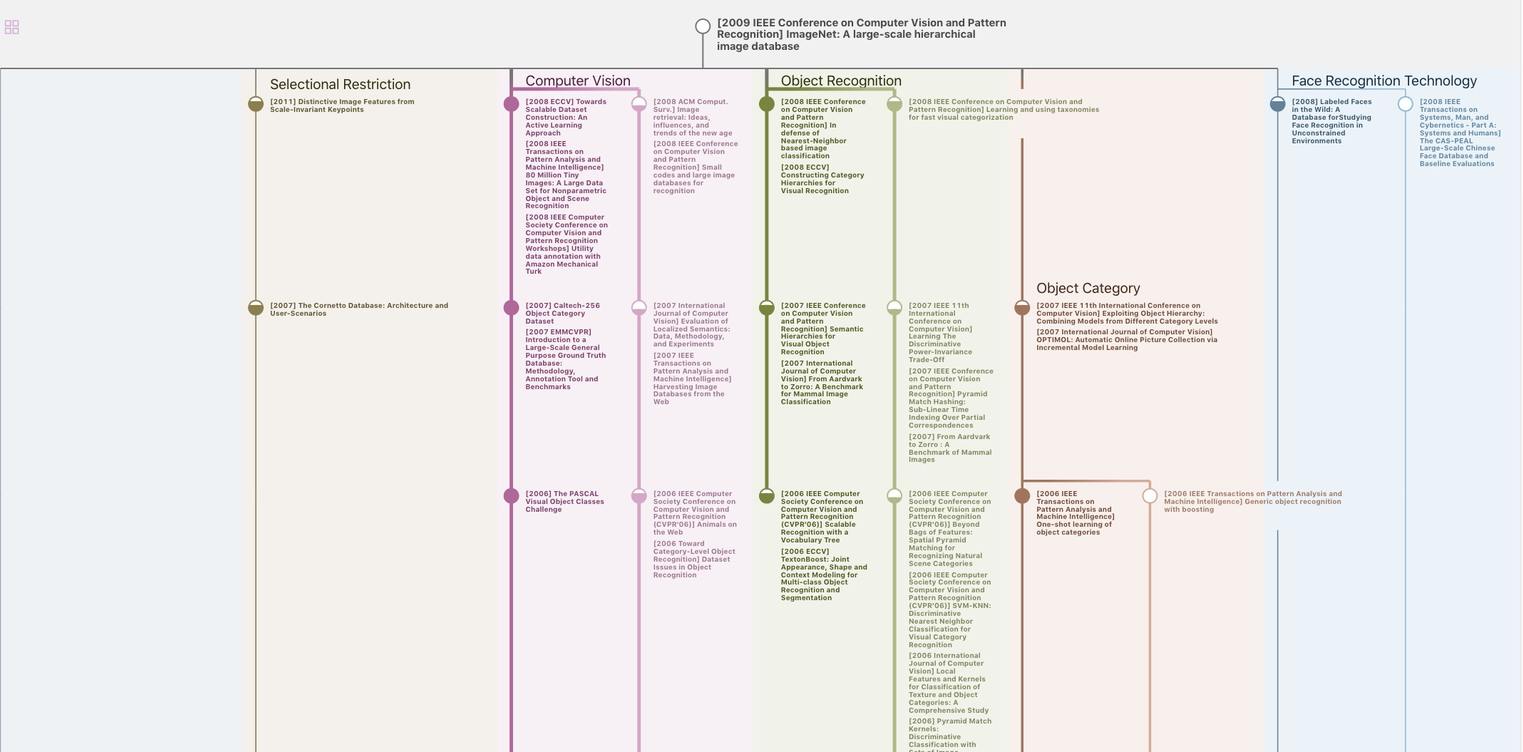
生成溯源树,研究论文发展脉络
Chat Paper
正在生成论文摘要