Mitigating distribution shift in machine learning-augmented hybrid simulation
CoRR(2024)
摘要
We study the problem of distribution shift generally arising in
machine-learning augmented hybrid simulation, where parts of simulation
algorithms are replaced by data-driven surrogates. We first establish a
mathematical framework to understand the structure of machine-learning
augmented hybrid simulation problems, and the cause and effect of the
associated distribution shift. We show correlations between distribution shift
and simulation error both numerically and theoretically. Then, we propose a
simple methodology based on tangent-space regularized estimator to control the
distribution shift, thereby improving the long-term accuracy of the simulation
results. In the linear dynamics case, we provide a thorough theoretical
analysis to quantify the effectiveness of the proposed method. Moreover, we
conduct several numerical experiments, including simulating a partially known
reaction-diffusion equation and solving Navier-Stokes equations using the
projection method with a data-driven pressure solver. In all cases, we observe
marked improvements in simulation accuracy under the proposed method,
especially for systems with high degrees of distribution shift, such as those
with relatively strong non-linear reaction mechanisms, or flows at large
Reynolds numbers.
更多查看译文
AI 理解论文
溯源树
样例
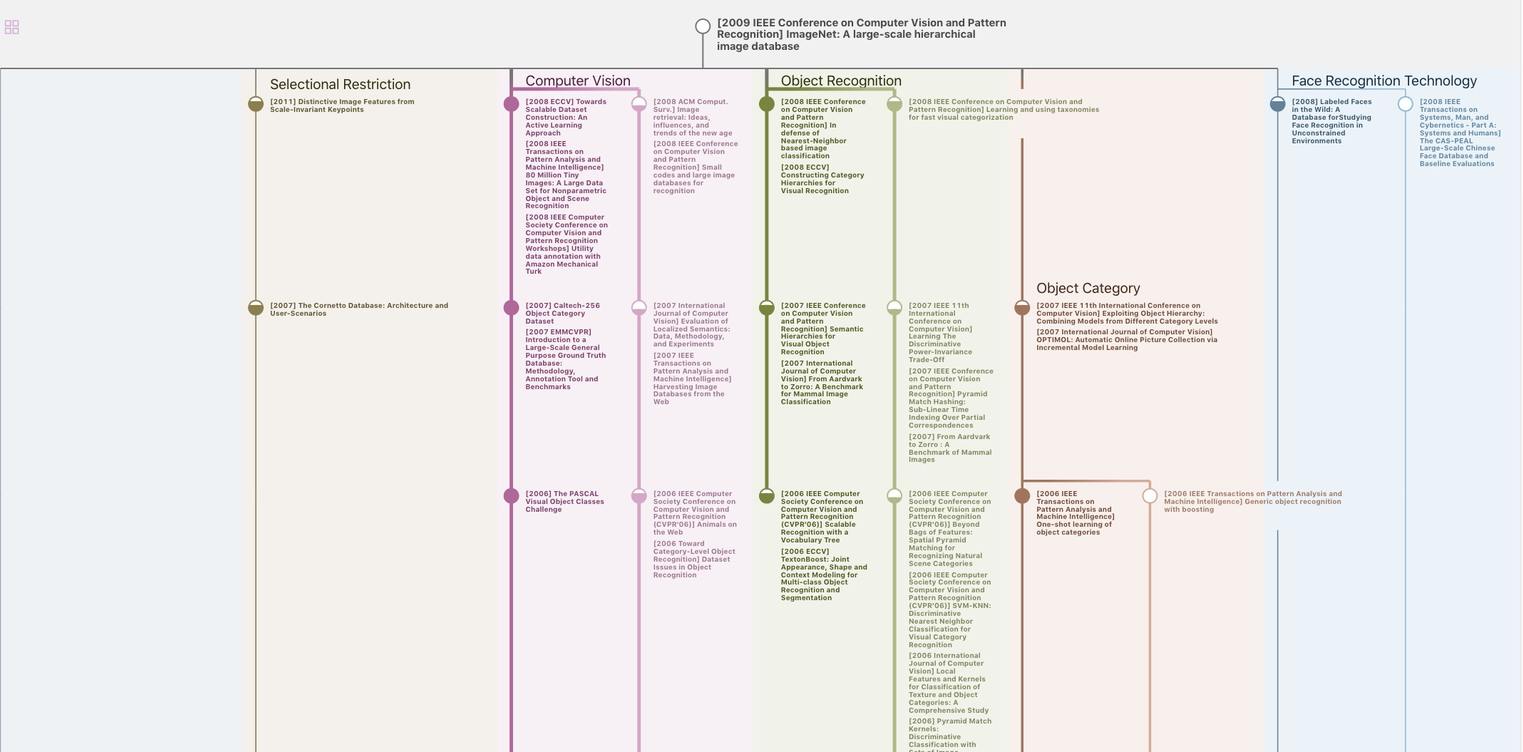
生成溯源树,研究论文发展脉络
Chat Paper
正在生成论文摘要