Causal Disentanglement Domain Generalization for time-series signal fault diagnosis
NEURAL NETWORKS(2024)
摘要
Domain generalization -based fault diagnosis (DGFD) presents significant prospects for recognizing faults without the accessibility of the target domain. Previous DGFD methods have achieved significant progress; however, there are some limitations. First, most DGFG methods statistically model the dependence between time -series data and labels, and they are superficial descriptions to the actual data -generating process. Second, most of the existing DGFD methods are only verified on vibrational time -series datasets, which is insufficient to show the potential of domain generalization in the fault diagnosis area. In response to the above issues, this paper first proposes a DGFD method named Causal Disentanglement Domain Generalization (CDDG), which can reestablish the data -generating process by disentangling time -series data into the causal factors (fault -related representation) and no -casual factors (domain -related representation) with a structural causal model. Specifically, in CDDG, causal aggregation loss is designed to separate the unobservable causal and non -causal factors. Meanwhile, the reconstruction loss is proposed to ensure the information completeness of the disentangled factors. We also introduce a redundancy reduction loss to learn efficient features. The proposed CDDG is verified on five cross -machine vibrational fault diagnosis cases and three cross -environment acoustical anomaly detection cases by comparing it with eight state-of-the-art (SOTA) DGFD methods. We publicize the open -source time -series DGFD Benchmark containing CDDG and the eight SOTA methods. The code repository will be available at https://github.com/ShaneSpace/DGFDBenchmark.
更多查看译文
关键词
Causal disentanglement,Domain generalization,Time-series fault diagnosis,DGFDBenchmark
AI 理解论文
溯源树
样例
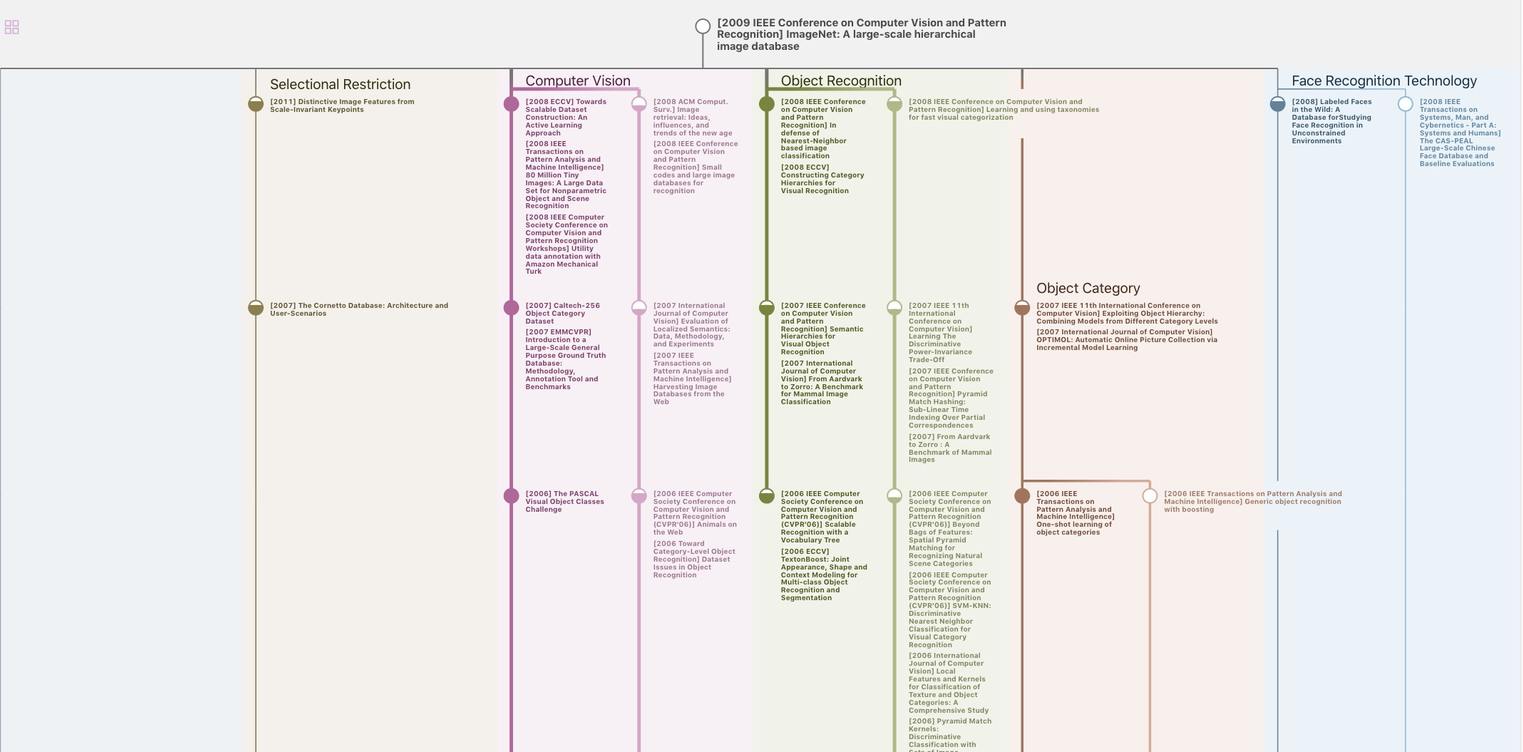
生成溯源树,研究论文发展脉络
Chat Paper
正在生成论文摘要