Automated Data Transformation and Feature Extraction for Oxygenation-Sensitive Cardiovascular Magnetic Resonance Images
Journal of Cardiovascular Translational Research(2024)
摘要
Oxygenation-sensitive cardiovascular magnetic resonance (OS-CMR) is a novel, powerful tool for assessing coronary function in vivo. The data extraction and analysis however are labor-intensive. The objective of this study was to provide an automated approach for the extraction, visualization, and biomarker selection of OS-CMR images. We created a Python-based tool to automate extraction and export of raw patient data, featuring 3336 attributes per participant, into a template compatible with common data analytics frameworks, including the functionality to select predictive features for the given disease state. Each analysis was completed in about 2 min. The features selected by both ANOVA and MIC significantly outperformed ( p < 0.001) the null set and complete set of features in two datasets, with mean AUROC scores of 0.89eatures f 0.94lete set of features in two datasets, with mean AUROC scores that our tool is suitable for automated data extraction and analysis of OS-CMR images. Graphical Abstract
更多查看译文
关键词
Oxygenation-sensitive cardiovascular magnetic resonance,Machine learning for medical imaging,Magnetic resonance imaging,Coronary vascular function
AI 理解论文
溯源树
样例
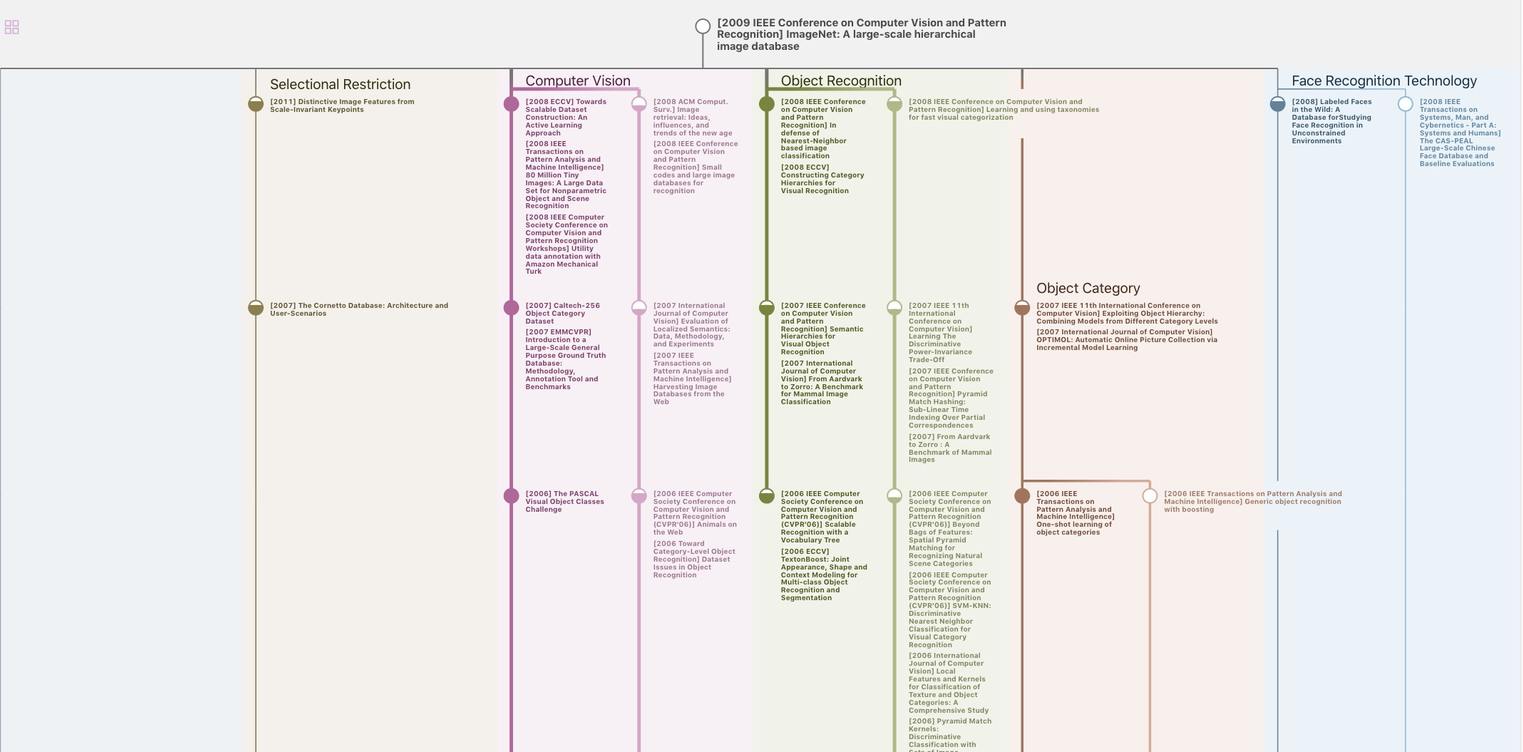
生成溯源树,研究论文发展脉络
Chat Paper
正在生成论文摘要