SICNet: Learning selective inter-slice context via Mask-Guided Self-knowledge distillation for NPC segmentation
JOURNAL OF VISUAL COMMUNICATION AND IMAGE REPRESENTATION(2024)
摘要
Accurate segmentation of nasopharyngeal carcinoma (NPC) in magnetic resonance (MR) images is crucial for radiotherapy planning. However, vanilla 2D/3D deep convolutional networks fail to gain satisfying NPC segmentation results, since 3D methods suffer from inter-slice discontinuities and 2D methods lack inter-slice context learning. To address this problem, this paper proposes a 2.5D learning Selective Inter-slice Context Network (SICNet) for NPC segmentation. The proposed SICNet comprises a main encoder that extracts target features from the middle slice and an auxiliary encoder that learns inter-slice context from adjacent slices. The features from two encoders are fused by the Inter-slice Context Fusion (ICF) module and then sent to the decoder for predictions. Furthermore, a knowledge distillation module named Mask-Guided Self-knowledge Distillation (MGSD) is proposed to help the auxiliary encoder learn context selectively. Specifically, with the guidance of mask labels, MGSD tells the auxiliary encoder where to look and how to learn, thus achieving selective inter-slice context learning and boosting segmentation accuracy. The proposed SICNet achieves a DSC of 74.38 +/- 11.99, an HD95 of 9.31 +/- 2.68, and an ASSD of 1.46 +/- 0.76 on an in-house NPC dataset, outperforming other state-of-theart segmentation methods.
更多查看译文
关键词
Nasopharyngeal carcinoma,Segmentation,Convolutional neural networks,Self-knowledge distillation,Selective inter-slice context
AI 理解论文
溯源树
样例
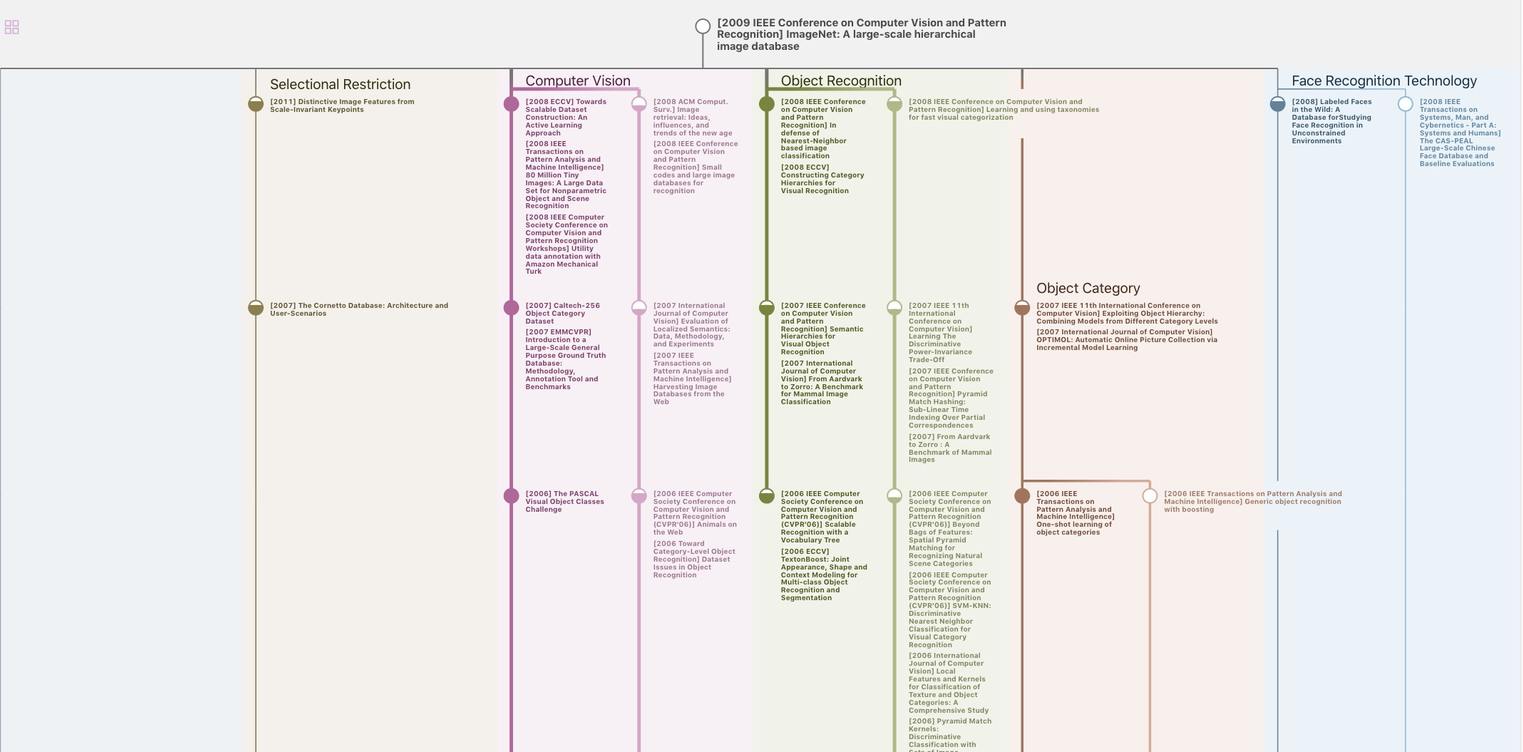
生成溯源树,研究论文发展脉络
Chat Paper
正在生成论文摘要