Using Machine-Learning to Decode Postoperative Hip Mortality Trends: Actionable Insights from an Extensive Clinical Dataset
Injury(2024)
摘要
BACKGROUND:Hip fractures are one of the most common injuries experienced by the general population. Despite advances in surgical techniques, postoperative mortality rates remain high. identifying relevant clinical factors associated with mortality is essential to preoperative risk stratification and tailored post-surgical interventions to improve patient outcomes. The purpose of this study aimed to identify preoperative risk factors and develop predictive models for increased hip fracture-related mortality within 30 days post-surgery, using one of the largest patient cohorts to date.METHODS:Data from the American College of Surgeons National Surgical Quality Improvement Program database, comprising 107,660 hip fracture patients treated with surgical fixation was used. A penalized regression approach, least absolute shrinkage and selection operator was employed to develop two predictive models: one using preoperative factors and the second incorporating both preoperative and postoperative factors.RESULTS:The analysis identified 68 preoperative factor outcomes associated with 30-day mortality. The combined model revealed 84 relevant factors, showing strong predictive power for determining postoperative mortality, with an AUC of 0.83.CONCLUSIONS:The study's comprehensive methodology provides risk assessment tools for clinicians to identify high-risk patients and optimize patient-specific care.
更多查看译文
关键词
Hip Fracture,Clinical Outcomes Research,Mortality,Statistical Methods,Surgery
AI 理解论文
溯源树
样例
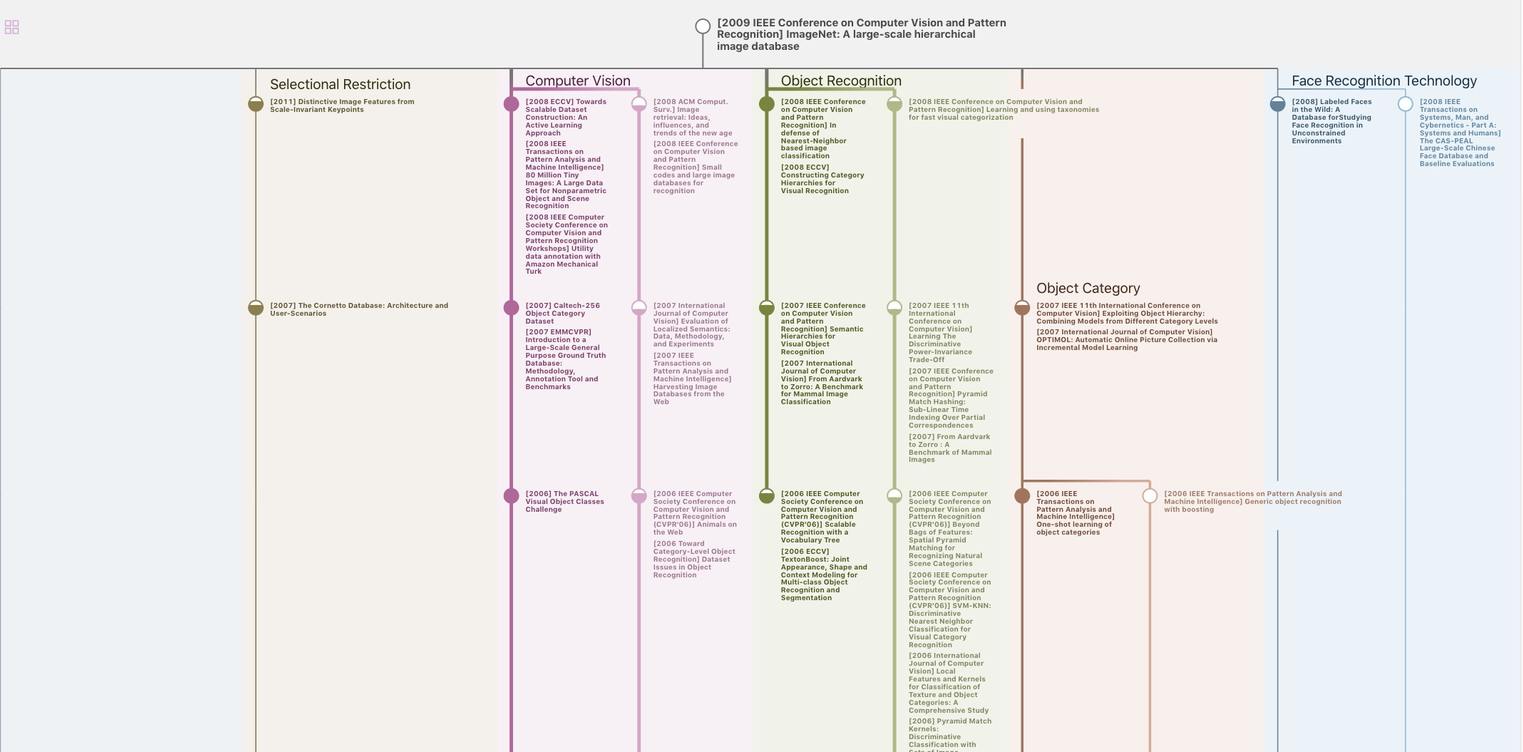
生成溯源树,研究论文发展脉络
Chat Paper
正在生成论文摘要