Machine Learning-Based Malicious Vehicle Detection for Security Threats and Attacks in Vehicle Ad-hoc Network (VANET) Communications
CoRR(2024)
摘要
With the rapid growth of Vehicle Ad-hoc Network (VANET) as a promising
technology for efficient and reliable communication among vehicles and
infrastructure, the security and integrity of VANET communications has become a
critical concern. One of the significant threats to VANET is the presence of
blackhole attacks, where malicious nodes disrupt the network's functionality
and compromise data confidentiality, integrity, and availability. In this
paper, we propose a machine learning-based approach for blackhole detection in
VANET. To achieve this task, we first create a comprehensive dataset comprising
normal and malicious traffic flows. Afterward, we study and define a promising
set of features to discriminate the blackhole attacks. Finally, we evaluate
various machine learning algorithms, including Gradient Boosting, Random
Forest, Support Vector Machines, k-Nearest Neighbors, Gaussian Naive Bayes, and
Logistic Regression. Experimental results demonstrate the effectiveness of
these algorithms in distinguishing between normal and malicious nodes. Our
findings also highlight the potential of machine learning based approach in
enhancing the security of VANET by detecting and mitigating blackhole attacks.
更多查看译文
关键词
Machine learning,VANET,malicious node
AI 理解论文
溯源树
样例
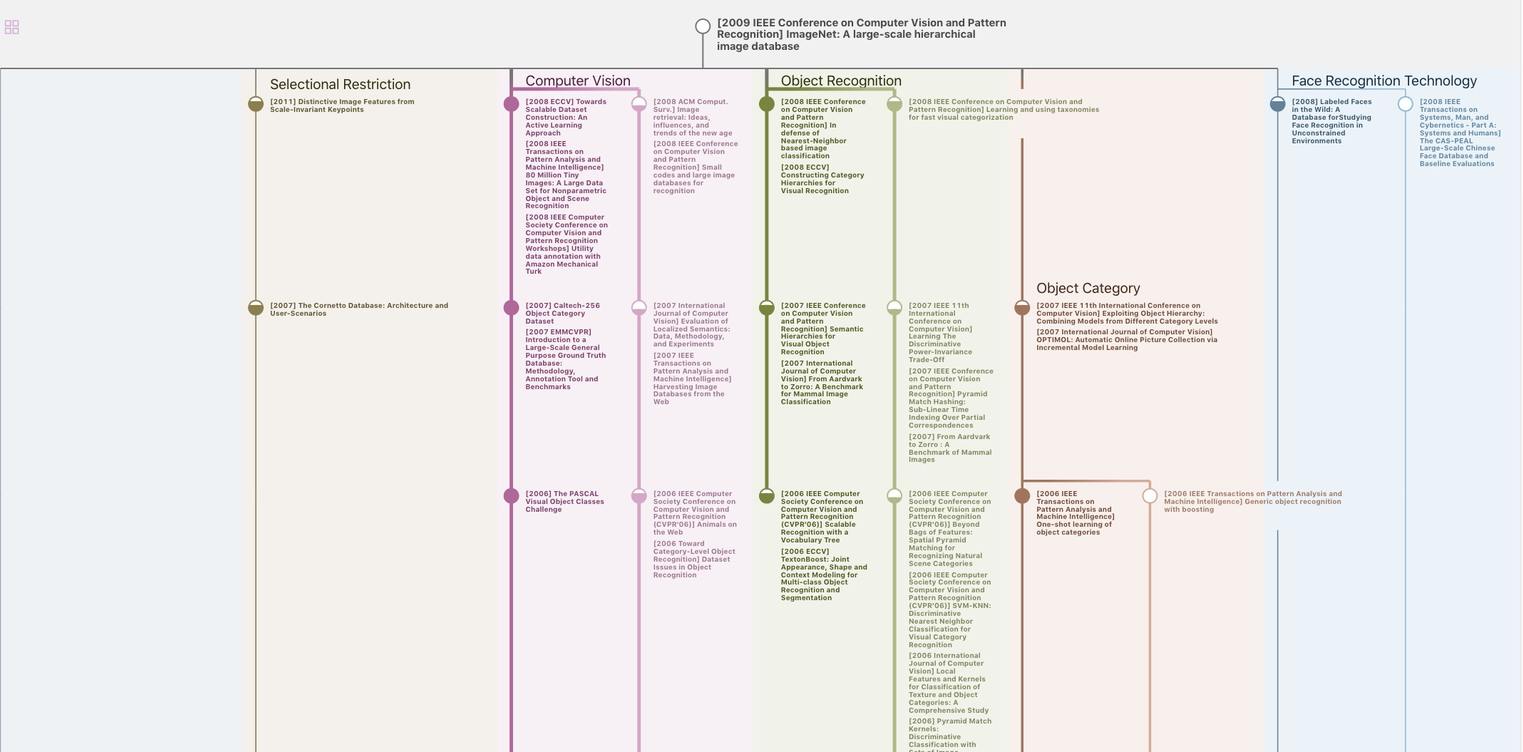
生成溯源树,研究论文发展脉络
Chat Paper
正在生成论文摘要