FiGCLIP: Fine-Grained CLIP Adaptation via Densely Annotated Videos
CoRR(2024)
摘要
While contrastive language image pretraining (CLIP) have exhibited impressive
performance by learning highly semantic and generalized representations, recent
works have exposed a fundamental drawback in its syntactic properties, that
includes interpreting fine-grained attributes, actions, spatial relations,
states, and details that require compositional reasoning. One reason for this
is that natural captions often do not capture all the visual details of a
scene. This leads to unaddressed visual concepts being misattributed to the
wrong words. And the pooled image and text features, ends up acting as a bag of
words, hence losing the syntactic information. In this work, we ask: Is it
possible to enhance CLIP's fine-grained and syntactic abilities without
compromising its semantic properties? We show that this is possible by adapting
CLIP efficiently on a high-quality, comprehensive, and relatively small
dataset. We demonstrate our adaptation strategy on VidSitu, a video situation
recognition dataset annotated with verbs and rich semantic role labels (SRL).
We use the SRL and verb information to create rule-based detailed captions,
making sure they capture most of the visual concepts. Combined with hard
negatives and hierarchical losses, these annotations allow us to learn a
powerful visual representation, dubbed Fine-Grained CLIP (FiGCLIP), that
preserves semantic understanding while being detail-oriented. We evaluate on
five diverse vision-language tasks in both fine-tuning and zero-shot settings,
achieving consistent improvements over the base CLIP model.
更多查看译文
AI 理解论文
溯源树
样例
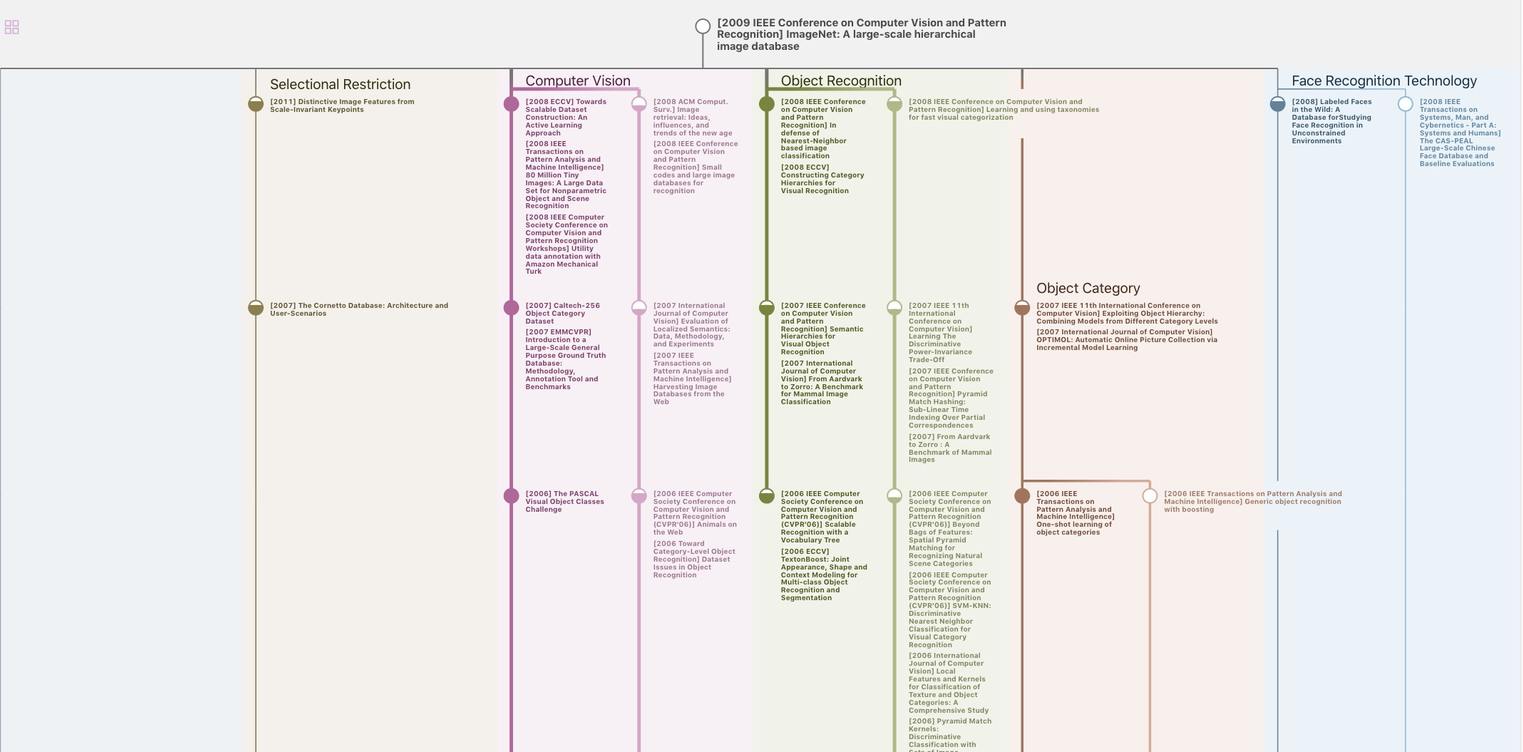
生成溯源树,研究论文发展脉络
Chat Paper
正在生成论文摘要