Machine Learning-based xApp for Dynamic Resource Allocation in O-RAN Networks
CoRR(2024)
摘要
The disaggregated, distributed and virtualised implementation of radio access
networks allows for dynamic resource allocation. These attributes can be
realised by virtue of the Open Radio Access Networks (O-RAN) architecture. In
this article, we tackle the issue of dynamic resource allocation using a
data-driven approach by employing Machine Learning (ML). We present an
xApp-based implementation for the proposed ML algorithm. The core aim of this
work is to optimise resource allocation and fulfil Service Level Specifications
(SLS). This is accomplished by dynamically adjusting the allocation of Physical
Resource Blocks (PRBs) based on traffic demand and Quality of Service (QoS)
requirements. The proposed ML model effectively selects the best allocation
policy for each base station and enhances the performance of scheduler
functionality in O-RAN - Distributed Unit (O-DU). We show that an xApp
implementing the Random Forest Classifier can yield high (85%) performance
accuracy for optimal policy selection. This can be attained using the O-RAN
instance state input parameters over a short training duration.
更多查看译文
AI 理解论文
溯源树
样例
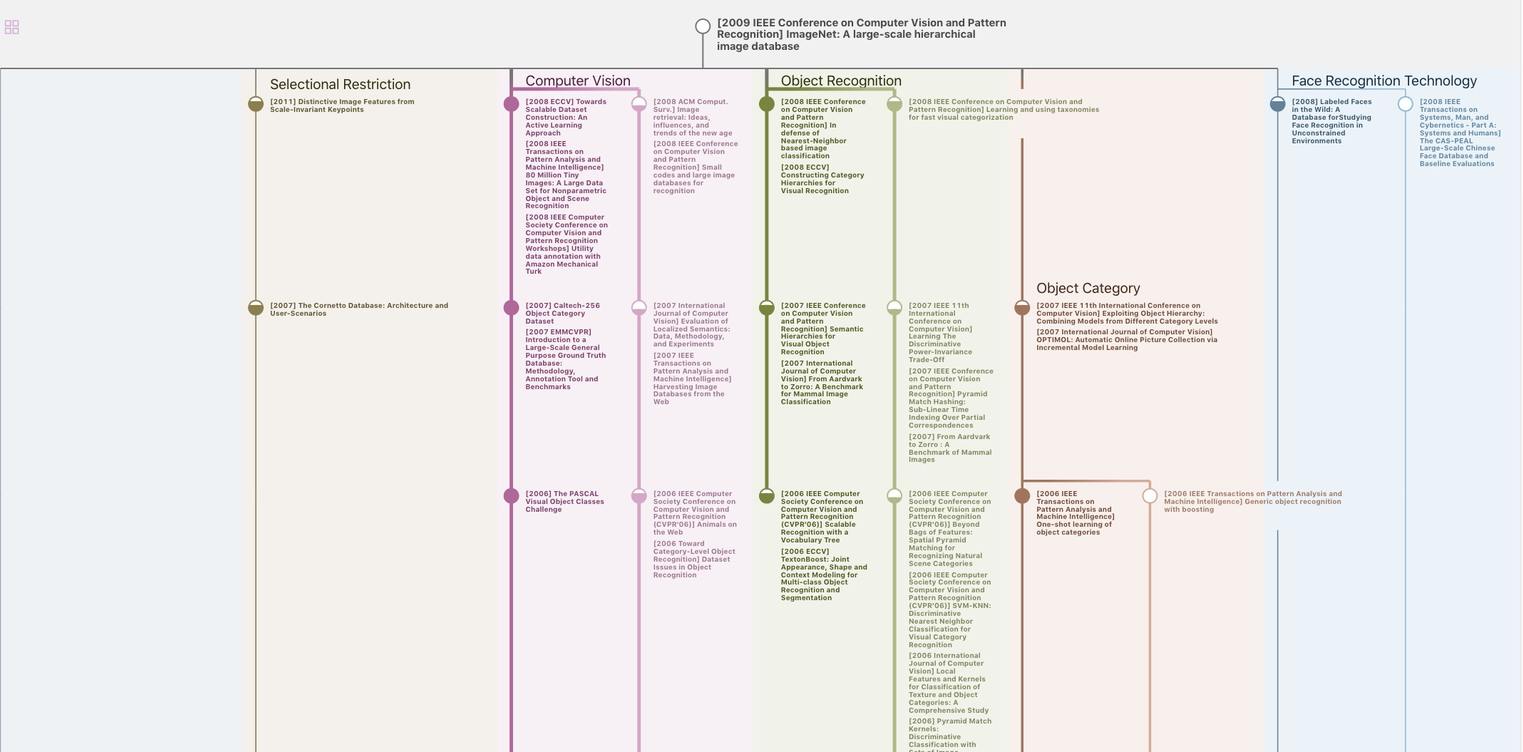
生成溯源树,研究论文发展脉络
Chat Paper
正在生成论文摘要