BoNuS: Boundary Mining for Nuclei Segmentation with Partial Point Labels.
IEEE transactions on medical imaging(2024)
摘要
Nuclei segmentation is a fundamental prerequisite in the digital pathology workflow. The development of automated methods for nuclei segmentation enables quantitative analysis of the wide existence and large variances in nuclei morphometry in histopathology images. However, manual annotation of tens of thousands of nuclei is tedious and time-consuming, which requires significant amount of human effort and domain-specific expertise. To alleviate this problem, in this paper, we propose a weakly-supervised nuclei segmentation method that only requires partial point labels of nuclei. Specifically, we propose a novel boundary mining framework for nuclei segmentation, named BoNuS, which simultaneously learns nuclei interior and boundary information from the point labels. To achieve this goal, we propose a novel boundary mining loss, which guides the model to learn the boundary information by exploring the pairwise pixel affinity in a multiple-instance learning manner. Then, we consider a more challenging problem, i.e., partial point label, where we propose a nuclei detection module with curriculum learning to detect the missing nuclei with prior morphological knowledge. The proposed method is validated on three public datasets, MoNuSeg, CPM, and CoNIC datasets. Experimental results demonstrate the superior performance of our method to the state-of-the-art weakly-supervised nuclei segmentation methods. Code: https://github.com/hust-linyi/bonus.
更多查看译文
关键词
Weakly-supervised segmentation,nuclei image,partial point label,boundary mining,pixel affinity
AI 理解论文
溯源树
样例
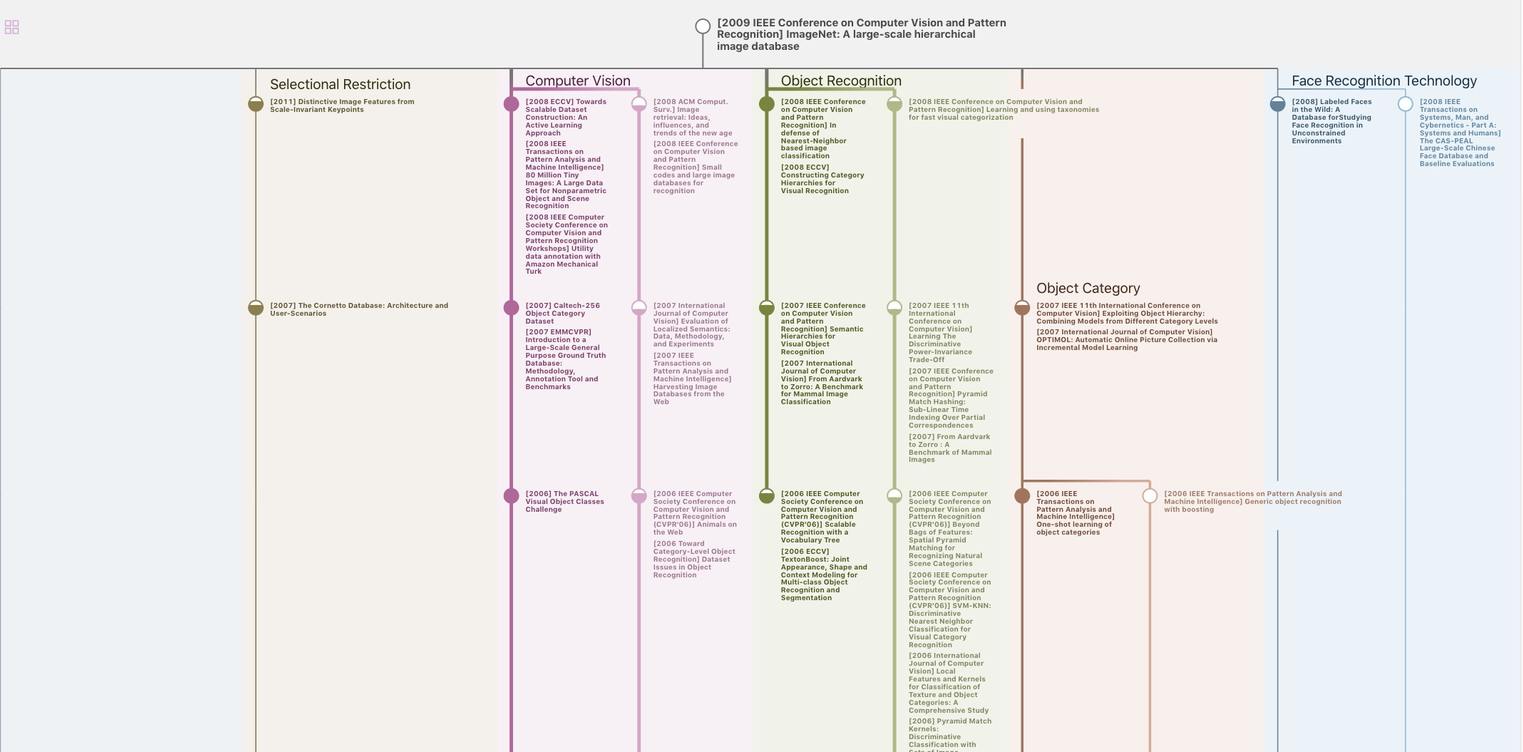
生成溯源树,研究论文发展脉络
Chat Paper
正在生成论文摘要