Always-Sparse Training by Growing Connections with Guided Stochastic Exploration
CoRR(2024)
摘要
The excessive computational requirements of modern artificial neural networks
(ANNs) are posing limitations on the machines that can run them. Sparsification
of ANNs is often motivated by time, memory and energy savings only during model
inference, yielding no benefits during training. A growing body of work is now
focusing on providing the benefits of model sparsification also during
training. While these methods greatly improve the training efficiency, the
training algorithms yielding the most accurate models still materialize the
dense weights, or compute dense gradients during training. We propose an
efficient, always-sparse training algorithm with excellent scaling to larger
and sparser models, supported by its linear time complexity with respect to the
model width during training and inference. Moreover, our guided stochastic
exploration algorithm improves over the accuracy of previous sparse training
methods. We evaluate our method on CIFAR-10/100 and ImageNet using ResNet, VGG,
and ViT models, and compare it against a range of sparsification methods.
更多查看译文
AI 理解论文
溯源树
样例
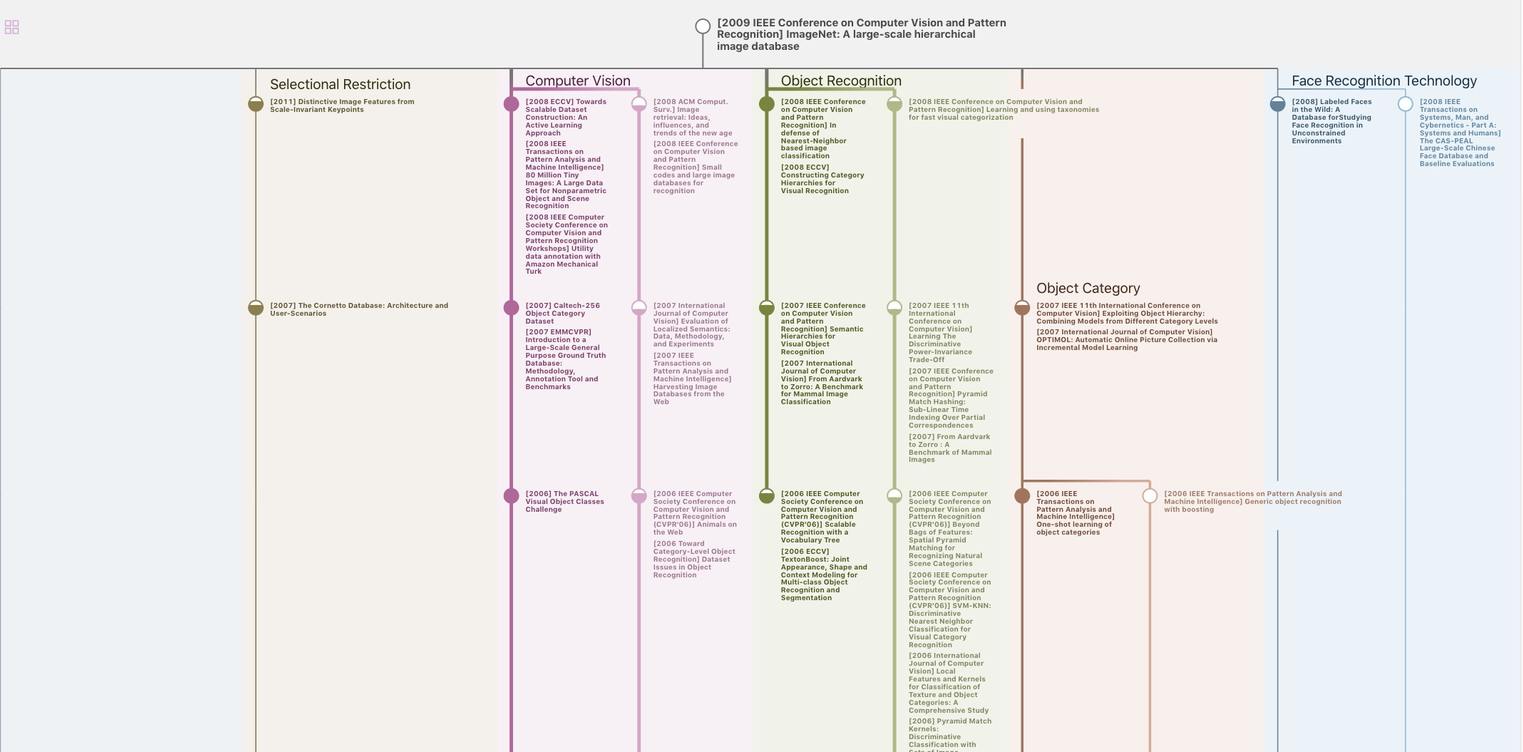
生成溯源树,研究论文发展脉络
Chat Paper
正在生成论文摘要