Iterative Residual Optimization Network for Limited-Angle Tomographic Reconstruction
IEEE TRANSACTIONS ON IMAGE PROCESSING(2024)
摘要
Limited-angle tomographic reconstruction is one of the typical ill-posed inverse problems, leading to edge divergence with degraded image quality. Recently, deep learning has been introduced into image reconstruction and achieved great results. However, existing deep reconstruction methods have not fully explored data consistency, resulting in poor performance. In addition, deep reconstruction methods are still mathematically inexplicable and unstable. In this work, we propose an iterative residual optimization network (IRON) for limited-angle tomographic reconstruction. First, a new optimization objective function is established to overcome false negative and positive artifacts induced by limited-angle measurements. We integrate neural network priors as a regularizer to explore deep features within residual data. Furthermore, the block-coordinate descent is employed to achieve a novel iterative framework. Second, a convolution assisted transformer is carefully elaborated to capture both local and long-range pixel interactions simultaneously. Regarding the visual transformer, the multi-head attention is further redesigned to reduce computational costs and protect reconstructed image features. Third, based on the relative error convergence property of the convolution assisted transformer, a mathematical convergence analysis is also provided for our IRON. Both numerically simulated and clinically collected real cardiac datasets are employed to validate the effectiveness and advantages of the proposed IRON. The results show that IRON outperforms other state-of-the-art methods.
更多查看译文
关键词
Convolution,Reconstruction algorithms,Transformers,Iron,Iterative methods,Image reconstruction,Optimization,Limited-angle CT,image reconstruction,model optimization,transformer,convergence
AI 理解论文
溯源树
样例
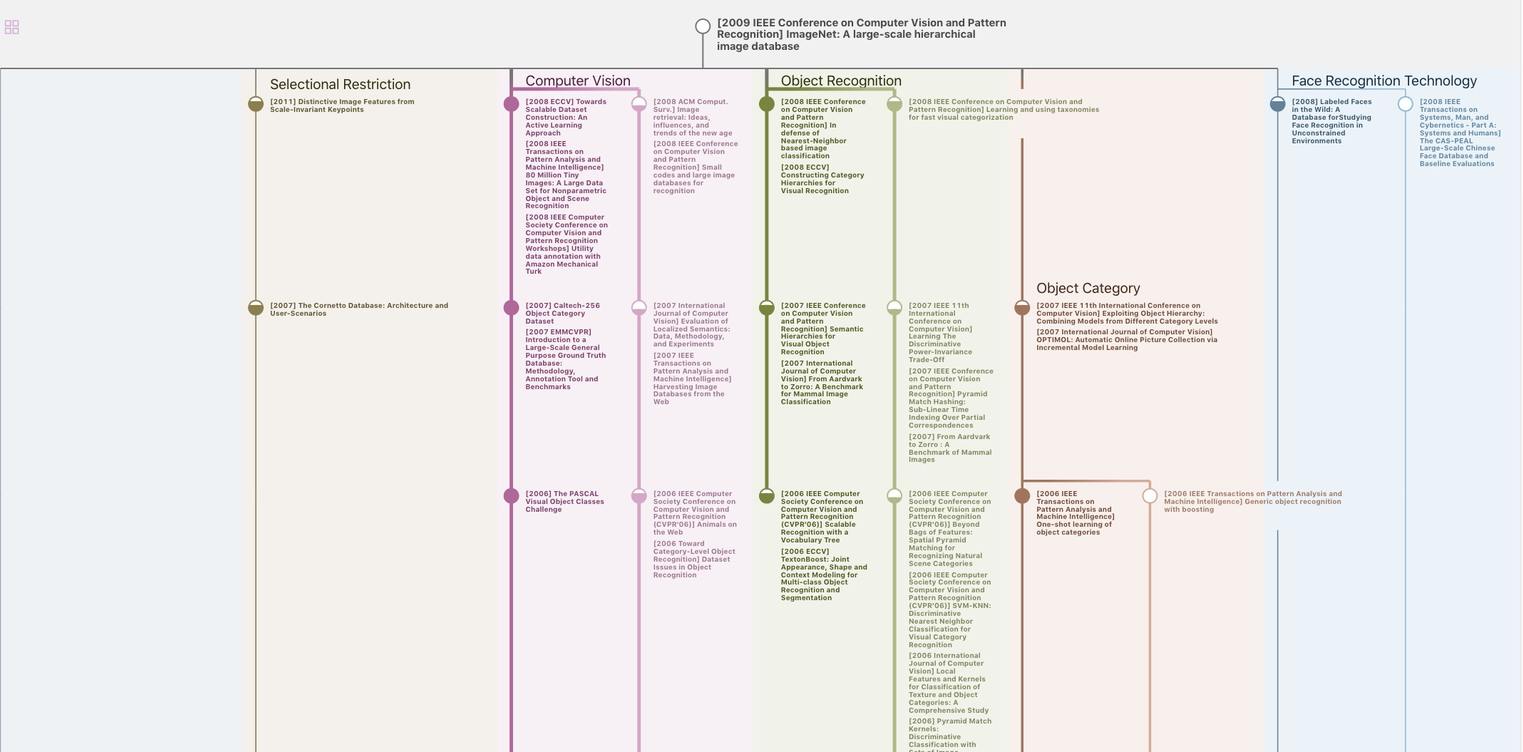
生成溯源树,研究论文发展脉络
Chat Paper
正在生成论文摘要