Identifying nonuniform distributions of rock properties and hydraulic fracture trajectories through deep learning in unconventional reservoirs
ENERGY(2024)
摘要
Predicting the trajectories of hydraulic fractures in unconventional reservoirs poses a significant challenge due to the heterogeneous nature of the rock matrix. Obtaining an accurate distribution of rock properties from limited measurements of rock samples near wellbores is difficult. In this study, we propose a deep learning framework that utilizes observations of hydraulic fracture patterns to identify nonuniform distributions of rock properties. Our approach utilizes the deep operator network (DeepONet) to map elastic properties to fracture geometries, and establishes an inverse problem to map fracture geometries back to elastic properties. Our results demonstrate a high level of accuracy in predicting nonplanar paths of single and multiple hydraulic fractures in heterogeneous formations. The well-trained model can rapidly evaluate numerous fracture patterns within seconds, surpassing the efficiency of finite element-based methods. Furthermore, fracture trajectories enable the identification of nonuniform distributions of rock properties. The maximum point-wise error of predicted Young's modulus compared to the ground truth is less than 20 %, with accurate identification of distribution patterns. Only a few regions exhibit relatively large errors, approaching 20 %. The presented approach provides valuable insights for the identification of reservoir properties in engineering applications.
更多查看译文
关键词
Deep learning,Hydraulic fracture,Unconventional reservoirs,Fracture trajectories,Heterogeneous formation
AI 理解论文
溯源树
样例
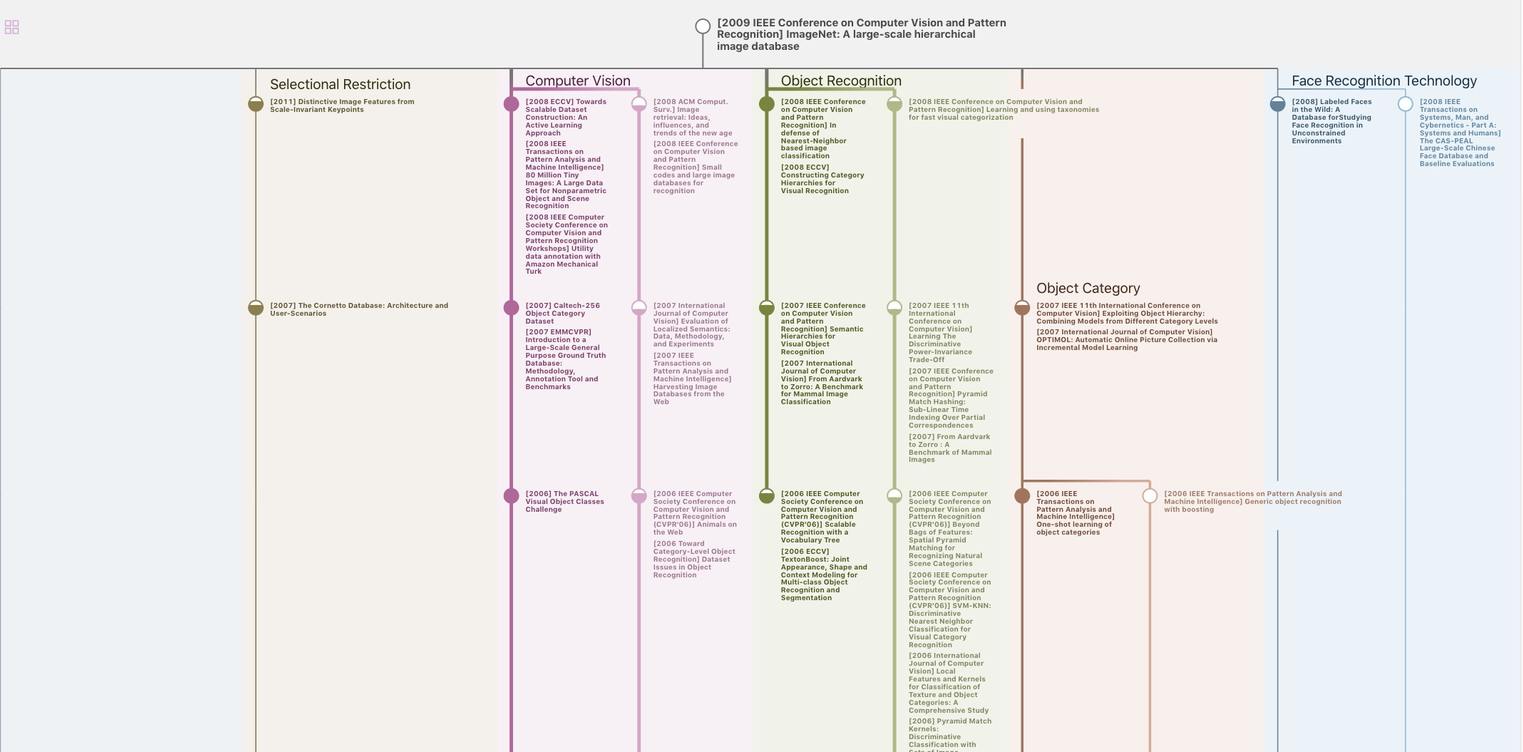
生成溯源树,研究论文发展脉络
Chat Paper
正在生成论文摘要