Reconstructing Disease Histories in Huge Discrete State Spaces
KI - Künstliche Intelligenz(2024)
摘要
Many progressive diseases develop unnoticed and insidiously at the beginning. This leads to an observational gap, since the first data on the disease can only be obtained after diagnosis. Mutual Hazard Networks address this gap by reconstructing latent disease dynamics. They model the disease as a Markov chain on the space of all possible combinations of progression events. This space can be huge: Given a set of n≥ 266 events, its size exceeds the number of atoms in the universe. Mutual Hazard Networks combine time-to-event modeling with generalized probabilistic graphical models, regularization, and modern numerical tensor formats to enable efficient calculations in large state spaces using compressed data formats. Here we review Mutual Hazard Networks and put them in the context of machine learning theory. We describe how the Mutual Hazard assumption leads to a compact parameterization of the models and show how modern tensor formats allow for efficient computations in large state spaces. Finally, we show how Mutual Hazard Networks reconstruct the most likely history of glioblastomas.
更多查看译文
关键词
Cancer genetics,Cancer progression model,Continuous-time Markov chains,Glioblastoma,Huge combinatorial state spaces,Low-rank tensor formats,Probabilistic graphical models,Proportional hazards,Reconstruction of latent processes
AI 理解论文
溯源树
样例
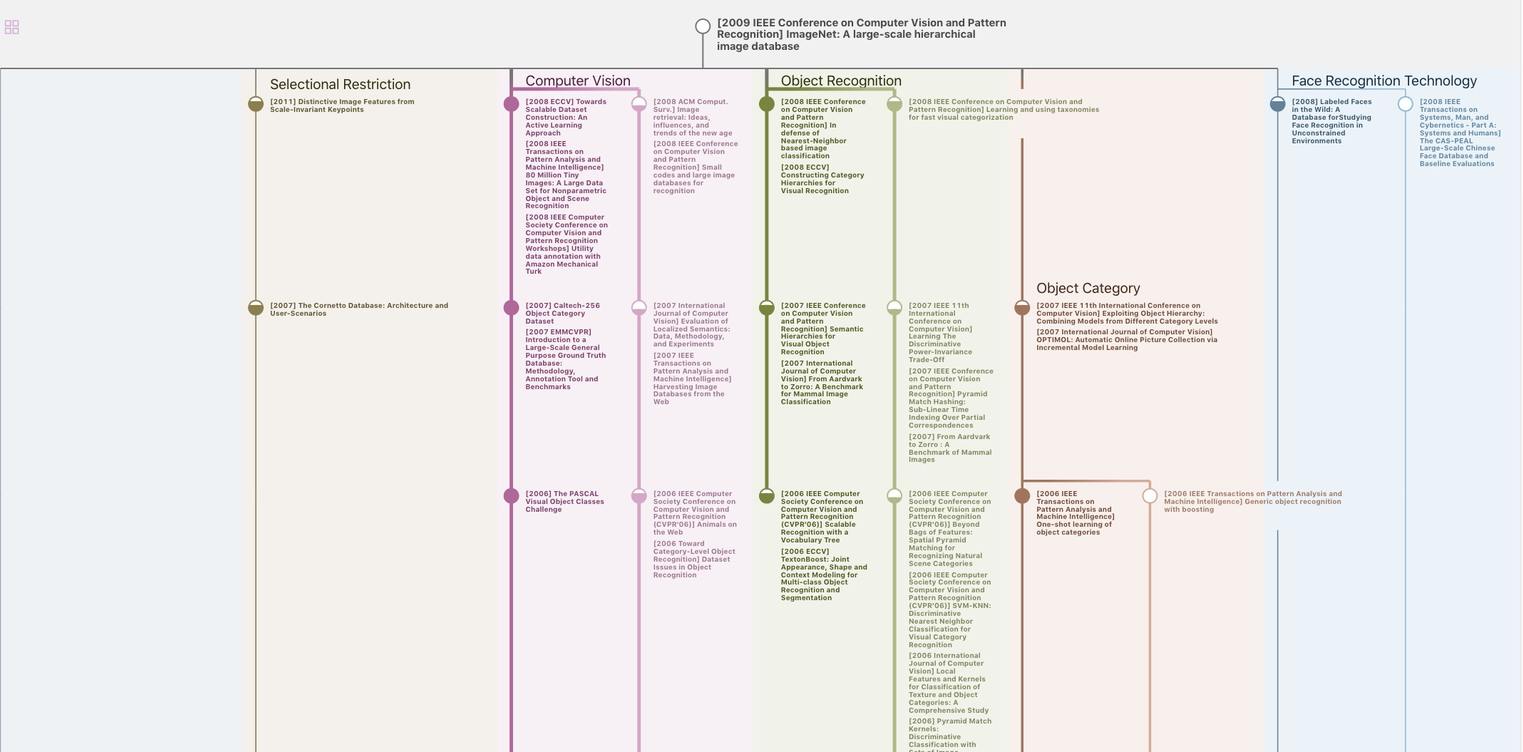
生成溯源树,研究论文发展脉络
Chat Paper
正在生成论文摘要