Digital Twin-Assisted Federated Learning Service Provisioning Over Mobile Edge Networks
IEEE TRANSACTIONS ON COMPUTERS(2024)
摘要
Federated Learning (FL) offers collaborative machine learning without data exposure, but challenges arise in the mobile edge network (MEC) environment due to limited resources and dynamic conditions. This paper presents a Digital Twin (DT)-assisted FL platform for MEC networks and introduces a novel multi-FL service framework to address resource dynamics and mobile users. We leverage DT models to optimize device scheduling and MEC resource allocation, aiming to maximize utility across FL services. Our work includes heuristic and constant approximation algorithms for offline multi-FL service scenarios and we also investigate an online setting of our solution with dynamic bandwidth and moving client conditions. To adapt to changing network conditions, we utilize historical bandwidth data in DTs and implement a deep reinforcement learning algorithm, Ra_DDPG, for automatic bandwidth allocation. Evaluation results demonstrate a significant 49.8% increase in system utility compared to a benchmark algorithm, showcasing the effectiveness of our approach.
更多查看译文
关键词
Digital twin (DT),digital twin-assisted mobile edge computing (DT-assisted MEC),multiple federated learning services,deep reinforcement learning algorithm
AI 理解论文
溯源树
样例
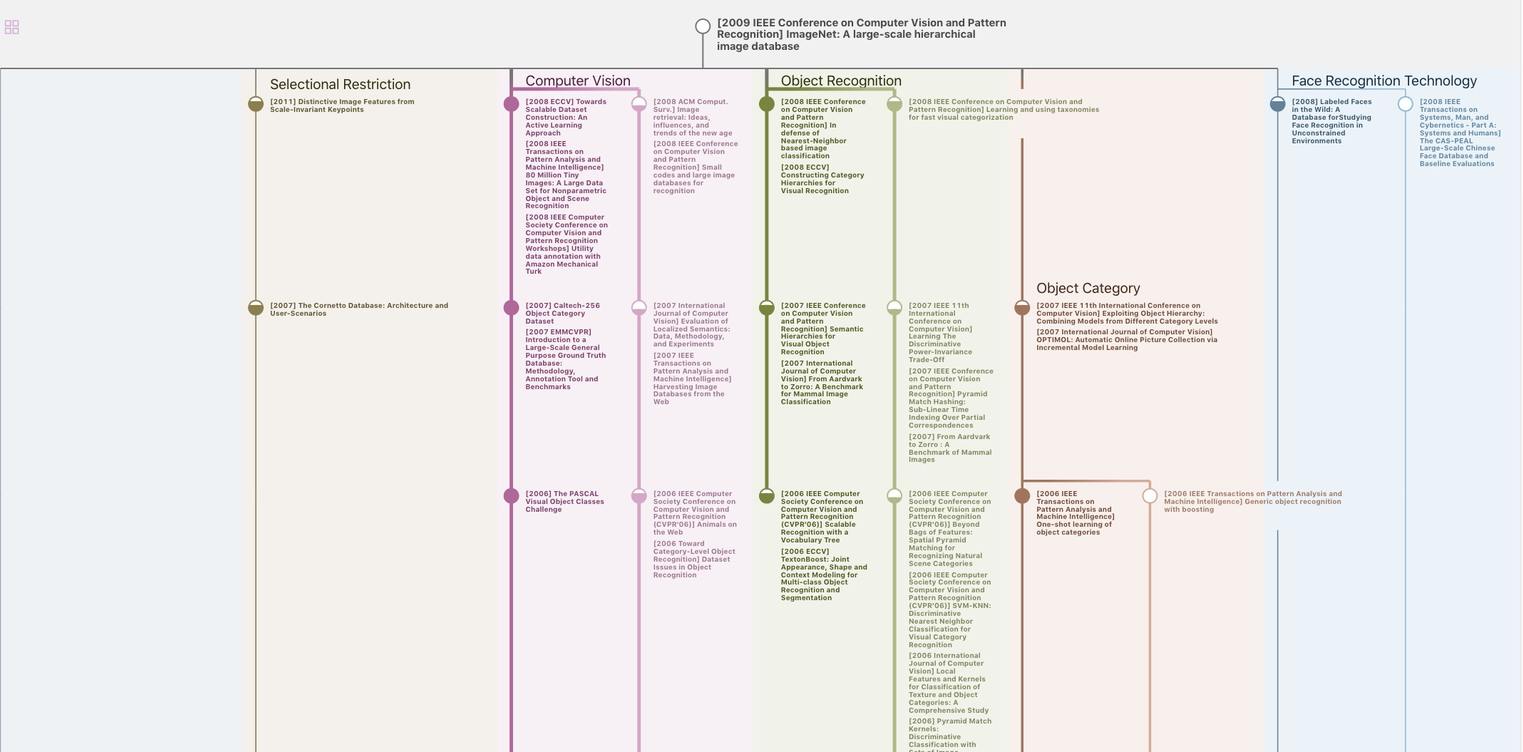
生成溯源树,研究论文发展脉络
Chat Paper
正在生成论文摘要