A novel time-frequency slice extraction method for target recognition and local enhancement of non-stationary signal features
ISA transactions(2024)
摘要
Blind deconvolution can remove the effects of complex paths and extraneous disturbances, thus recovering simple features of the original fault source, and is used extensively in the field of fault diagnosis. However, it can only identify and extract the statistical mean of the fault impact features in a single domain and is unable to simultaneously highlight the local features of the signal in the time-frequency domain. Therefore, the extraction effect of weak fault signals is generally not ideal. In this paper, a new time-frequency slice extraction method is proposed. The method first computes a high temporal resolution spectrum of the signal by short-time Fourier transform to obtain multiple frequency slices with distinct temporal waveforms. Subsequently, the constructed harmonic spectral feature index is used to quantify and target the intensity of feature information in each frequency slice and enhance their fault characteristics using maximum correlation kurtosis deconvolution. Enhancing the local features of selected frequency slice clusters can reduce noise interference and obtain signal components with more obvious fault signatures. Finally, the validity of the method was confirmed by a simulated signal and fault diagnosis of the rolling bearing outer and inner rings was accomplished sequentially. Compared with other common deconvolution methods, the proposed method obtains more accurate and effective results in identifying fault messages.
更多查看译文
关键词
Time-frequency slice extraction,Target diagnosis,Local feature enhancement,Deconvolution,Rolling bearing
AI 理解论文
溯源树
样例
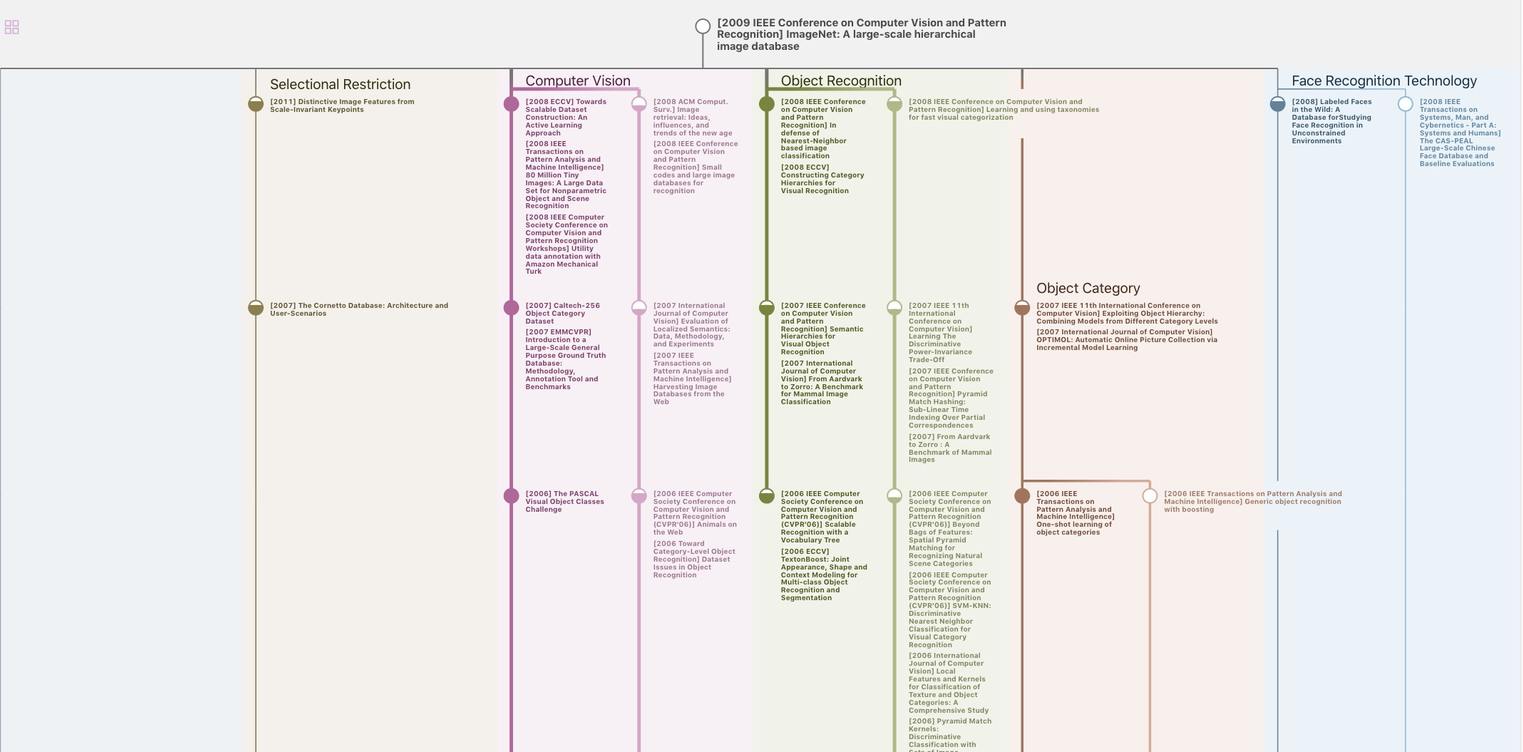
生成溯源树,研究论文发展脉络
Chat Paper
正在生成论文摘要