Vessel turnaround time prediction: A machine learning approach
OCEAN & COASTAL MANAGEMENT(2024)
摘要
Uncertainty in vessel turnaround time (VTT) is troublesome and would reduce the operational efficiency in port management, potentially causing economic losses. Despite vessels generally providing their estimated departure time (EDT), there is frequently a considerable difference between the EDT and the actual departure time (ADT) of vessels due to various factors such as unexpected port handling inefficiency. This variability complicates the coordination of efficient port operations. Our research aims to address this issue by employing an extreme gradient boosting (XGBoost) regression model to predict the VTT, using vessel arrival and departure data at the Hong Kong Port for the year 2022 and the first quarter of 2023. The proposed machine learning approach can provide more accurate predictions on VTT on average compared to the EDT data reported by vessels themselves, with a substantial reduction in both mean absolute error (MAE) and root mean square error (RMSE) of 23% (from 5.1 h to 3.9 h) and 24% (from 8.0 h to 6.1 h), respectively. These results present a significant leap forward in the predictive capabilities for the VTT and lay the foundation for further research into improving vessel scheduling efficiency, reducing port congestion and enhancing overall port performance.
更多查看译文
关键词
Maritime transport,Vessel turnaround time prediction,Machine learning in port management,Port efficiency improvement
AI 理解论文
溯源树
样例
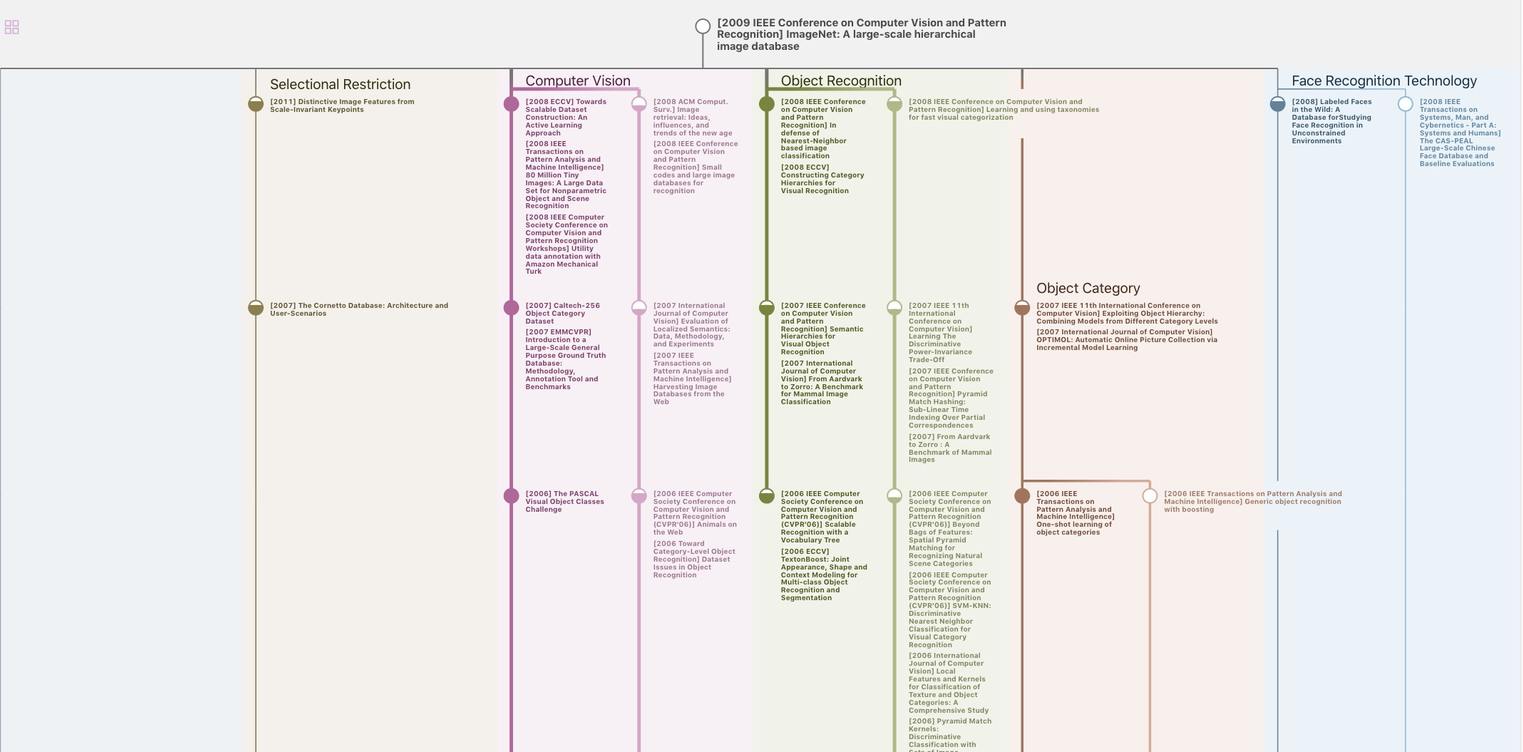
生成溯源树,研究论文发展脉络
Chat Paper
正在生成论文摘要