Online distortion simulation using generative machine learning models: A step toward digital twin of metallic additive manufacturing
JOURNAL OF INDUSTRIAL INFORMATION INTEGRATION(2024)
摘要
In the era of Industry 4.0 and smart manufacturing, Wire Arc Additive Manufacturing (WAAM) stands at the forefront, driving a paradigm shift towards automated, digitalized production. However, online simulation remains a technical barrier toward building a Digital Twin (DT) for metallic AM due to the prolonged computing time of numerical simulations and limitations in accuracy of current data-driven models. This study addresses these issues by introducing an adaptive online simulation model for predicting distortion fields, utilizing a diffusion model architecture for distortion process modelling with a Vector Quantized Variational AutoEncoder coupled with Generative Adversarial Network (VQVAE-GAN) backbone for spatial feature extraction, complemented by a Recurrent Neural Network (RNN) for time-scale result fusion. Pretrained offline with Finite Element Method (FEM) simulated distortion fields, the model successfully predicts distortion fields online using laser-scanned point clouds during the deposition process. Experimental validation on seven thin-wall structures demonstrated its superior performance, achieving a Root Mean Square Error (RMSE) below 0.9 m, outperforming FEM by 143 % and Artificial Neural Networks (ANN) based methods by 151 %, marking a significant stride towards realizing an AM-DT.
更多查看译文
关键词
WAAM,Physics-informed ML,FEM simulation,Digital twin,Metallic AM
AI 理解论文
溯源树
样例
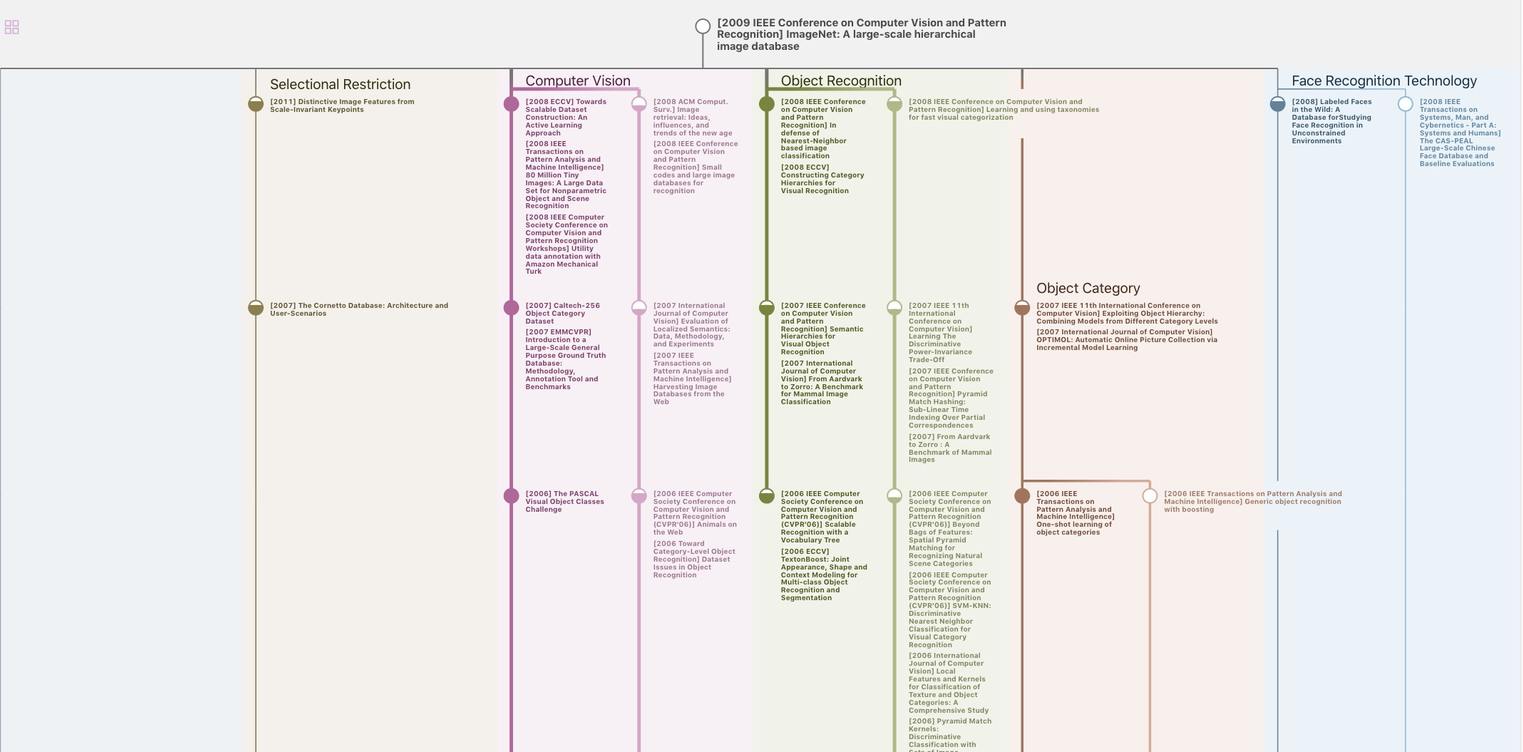
生成溯源树,研究论文发展脉络
Chat Paper
正在生成论文摘要