Multi-fidelity physics constrained neural networks for dynamical systems
COMPUTER METHODS IN APPLIED MECHANICS AND ENGINEERING(2024)
摘要
Physics -constrained neural networks are commonly employed to enhance prediction robustness compared to purely data -driven models, achieved through the inclusion of physical constraint losses during the model training process. However, one of the major challenges of physics -constrained neural networks consists of the training complexity especially for highdimensional systems. In fact, conventional physics -constrained models rely on singular -fidelity data necessitating the assessment of physical constraints within high -dimensional fields, which introduces computational difficulties. Furthermore, due to the fixed input size of the neural networks, employing multi -fidelity training data can also be cumbersome. In this paper, we propose the Multi -Scale Physics -Constrained Neural Network (MSPCNN), which offers a novel methodology for incorporating data with different levels of fidelity into a unified latent space through a customised multi-fidelity autoencoder. Additionally, multiple decoders are concurrently trained to map latent representations of inputs into various fidelity physical spaces. As a result, during the training of predictive models, physical constraints can be evaluated within low-fidelity spaces, yielding a trade-off between training efficiency and accuracy. In addition, unlike conventional methods, MSPCNN also manages to employ multi-fidelity data to train the predictive model. We assess the performance of MSPCN N in two fluid dynamics problems, namely a two-dimensional Burgers' system and a shallow water system. Numerical results clearly demonstrate the enhancement of prediction accuracy and noise robustness when introducing physical constraints in low-fidelity fields. On the other hand, as expected, the training complexity can be significantly reduced by computing physical constraint loss in the low-fidelity field rather than the high-fidelity one.
更多查看译文
关键词
Reduced-order modelling,Multiple fidelity,Physical constraints,LSTM networks,Dynamical systems,Long-time prediction
AI 理解论文
溯源树
样例
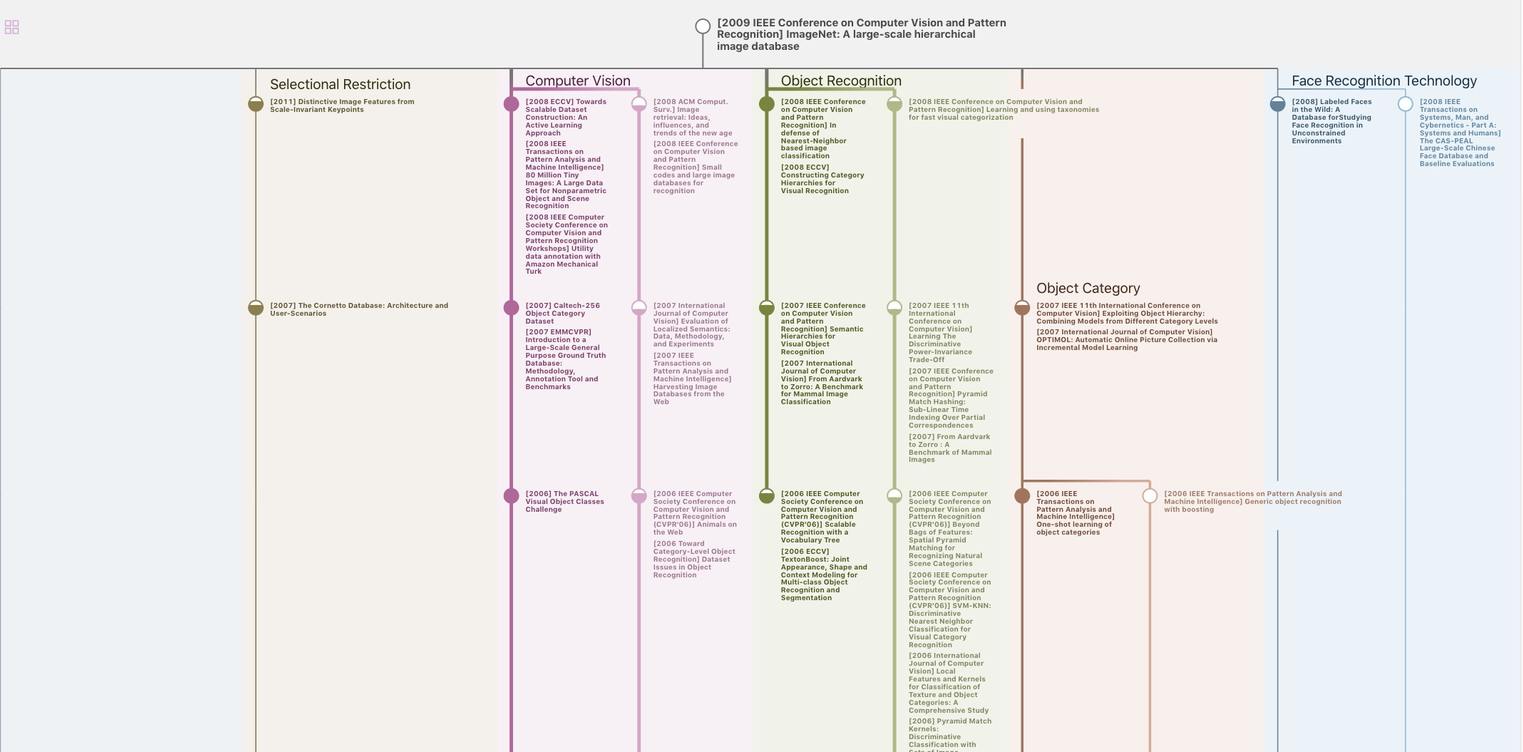
生成溯源树,研究论文发展脉络
Chat Paper
正在生成论文摘要