CaSE-NeRF: Camera Settings Editing of Neural Radiance Fields
ADVANCES IN COMPUTER GRAPHICS, CGI 2023, PT II(2024)
摘要
Neural Radiance Fields (NeRF) have shown excellent quality in three-dimensional (3D) reconstruction by synthesizing novel views from multi-view images. However, previous NeRF-based methods do not allow users to perform user-controlled camera setting editing in the scene. While existing works have proposed methods to modify the radiance field, these modifications are limited to camera settings within the training set. Hence, we present Camera Settings Editing of Neural Radiance Fields (CaSE-NeRF) to recover a radiance field from a set of views with different camera settings. In our approach, we allow users to perform controlled camera settings editing on the scene and synthesize the novel view images of the edited scene without re-training the network. The key to our method lies in modeling each camera parameter separately and rendering various 3D defocus effects based on thin lens imaging principles. By following the image processing of real cameras, we implicitly model it and learn gains that are continuous in the latent space and independent of the image. The control of color temperature and exposure is plug-and-play, and can be easily integrated into NeRF-based frameworks. As a result, our method allows for manual and free post-capture control of the viewpoint and camera settings of 3D scenes. Through our extensive experiments on two real-scene datasets, we have demonstrated the success of our approach in reconstructing a normal NeRF with consistent 3D geometry and appearance. Our related code and data is available at https://github.com/CPREgroup/CaSE- NeRF.
更多查看译文
关键词
neural radiance field,camera setting editing,novel view synthesis,image-based rendering
AI 理解论文
溯源树
样例
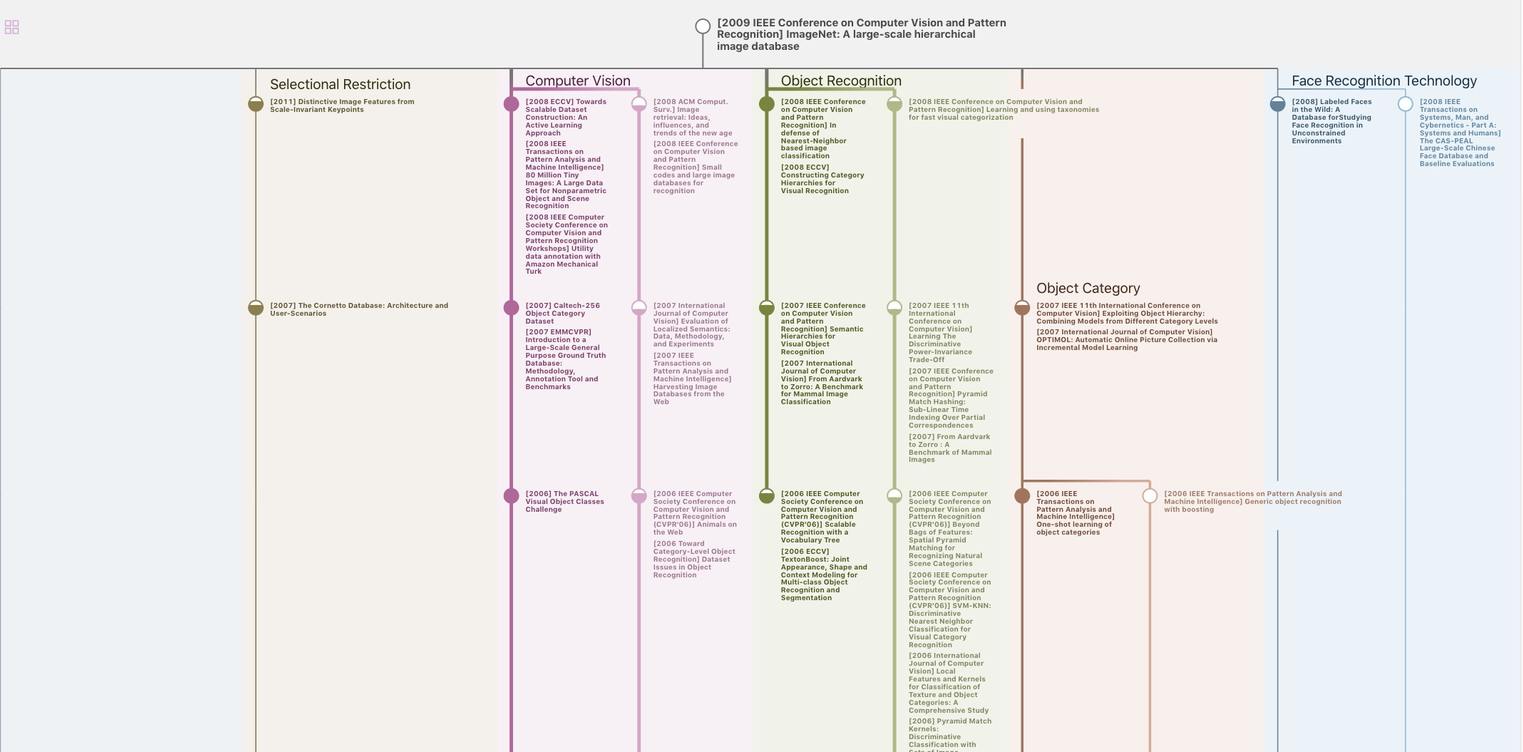
生成溯源树,研究论文发展脉络
Chat Paper
正在生成论文摘要