Improving Transferability of Adversarial Attacks with Gaussian Gradient Enhance Momentum
PATTERN RECOGNITION AND COMPUTER VISION, PRCV 2023, PT IX(2024)
摘要
Deep neural networks (DNNs) can be susceptible to subtle perturbations that may mislead the model. While adversarial attacks are successful in the white-box setting, they are less effective in the blackbox setting. To address this issue, we propose an attack method that simulates a smoothed loss function by sampling from a Gaussian distribution. We calculated the Gaussian gradient to enhance the momentum based on the smoothing loss function to improve the transferability of the attack. Moreover, We further improve transferability by changing the sampling range to make the Gaussian gradient prospective. Our method has been extensively tested through experiments, and the results show that it achieves higher transferability compared to state-of-the-art (SOTA) methods.
更多查看译文
关键词
Deep neural networks,adversarial examples,black-box attack,transferability,Gaussian gradient
AI 理解论文
溯源树
样例
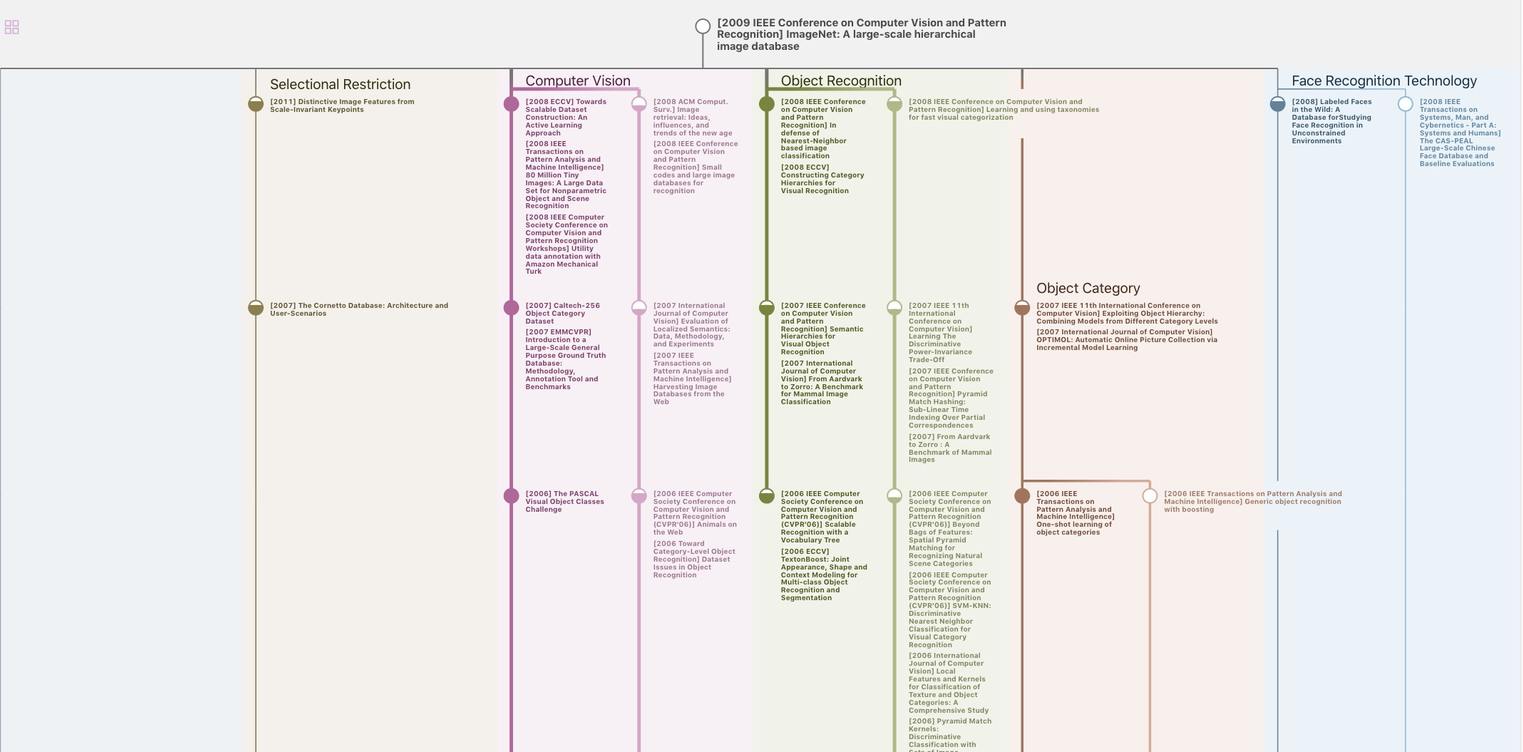
生成溯源树,研究论文发展脉络
Chat Paper
正在生成论文摘要