Global Consistency Enhancement Network for Weakly-Supervised Semantic Segmentation
PATTERN RECOGNITION AND COMPUTER VISION, PRCV 2023, PT IX(2024)
摘要
Generation methods for reliable class activation maps (CAMs) are essential for weakly-supervised semantic segmentation. These methods usually face the challenge of incomplete and inaccurate CAMs due to intra-class inconsistency of final features and inappropriate use of deep-level ones. To alleviate these issues, we propose the Global Consistency Enhancement Network (GCENet) that consists of Middle-level feature Auxiliary Module (MAM), Intra-class Consistency Enhancement Module (ICEM), and Critical Region Suppression Module (CRSM). Specifically, MAM uses middle-level features which carry clearer edges information and details to enhance output features. Then, for the problem of incomplete class activation maps caused by the high variance of local context of the image, ICEM is proposed to enhance the representation of features. It takes into account the intra-class global consistency and the local particularity. Furthermore, CRSM is proposed to solve the problem of excessive CAMs caused by the over-activation of features. It activates the low-discriminative regions appropriately, thus improving the quality of class activation maps. Through our comprehensive experiments, our method outperforms all other competitors and well demonstrates its effectiveness on the PASCAL VOC2012 dataset.
更多查看译文
关键词
Weakly-supervised semantic segmentation,Semantic segmentation,Intra-class consistency
AI 理解论文
溯源树
样例
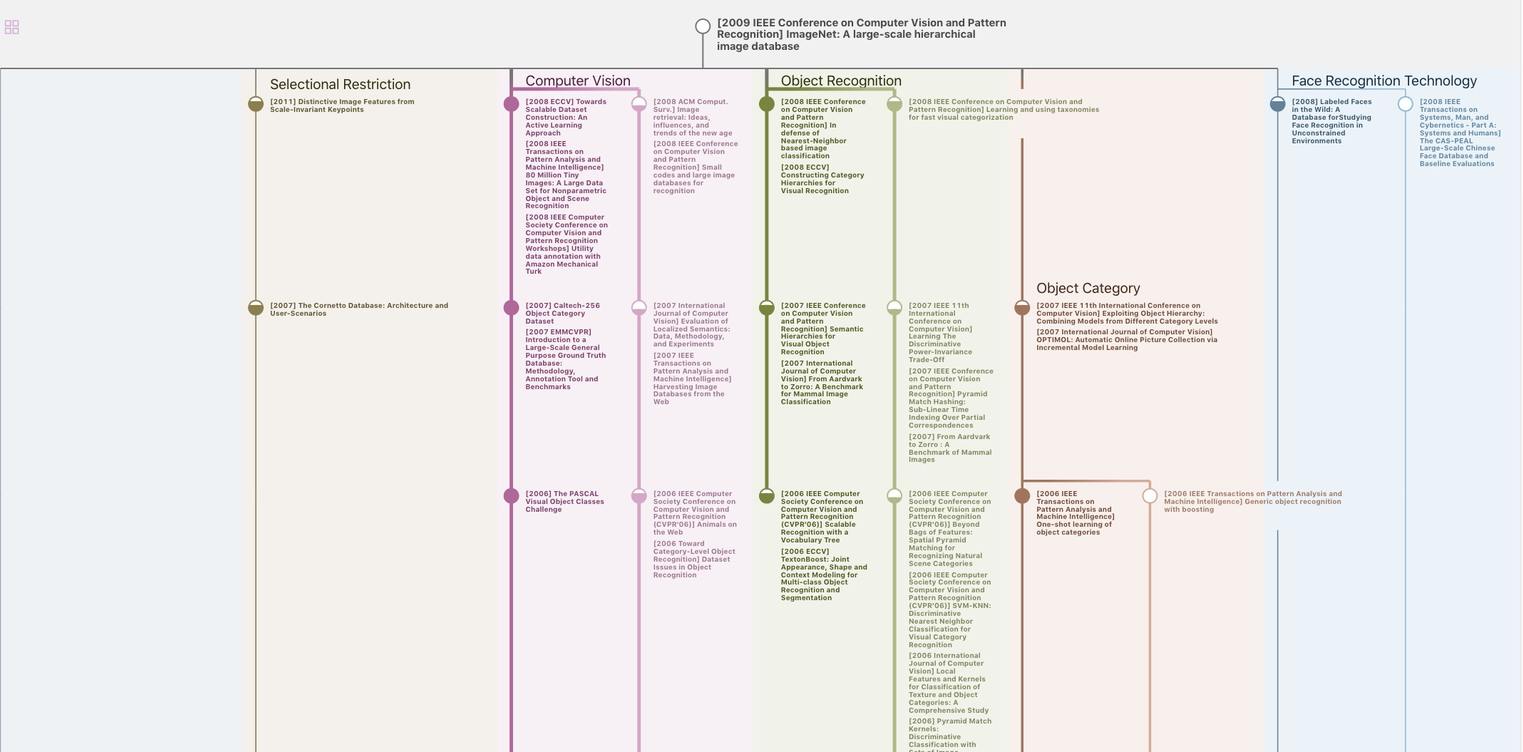
生成溯源树,研究论文发展脉络
Chat Paper
正在生成论文摘要