Central and Directional Multi-neck Knowledge Distillation
PATTERN RECOGNITION AND COMPUTER VISION, PRCV 2023, PT VII(2024)
摘要
There are already many mature methods for single-teacher knowledge distillation in object detection models, but the development of multi-teacher knowledge distillation methods has been slow due to the complexity of knowledge fusion among multiple teachers. In this paper, we point out that different teacher models have different detection capabilities for a certain category, and through experiments, we find that for individual target instances, there are differences in the main feature regions of the target, and the detection results also have randomness. Networks that are weak in overall detection performance for a certain category sometimes perform well, so it is not appropriate to simply select the best learning based on detection results. Therefore, we propose a novel multi-teacher distillation method that divides the central and boundary features of the instance target region through the detection of teacher models, and uses clustering to find the center of the response features of each category of teacher models as prior knowledge to guide the learning direction of the student model for the category as a whole. Since our method only needs to calculate the loss on the feature map, FGD can be applied to multiple teachers with the same components. We conducted experiments on various detectors with different backbones, and the results show that our student detector achieved excellent mAP improvement. Our distillation method achieved an mAP of 39.4% on COCO2017 based on the ResNet-50 backbone, which is higher than the single-teacher distillation learning method of the baseline model. Our code and training logs can be obtained at https://github.com/CCCCPRCV/Multi Neck.
更多查看译文
关键词
Knowledge distillation,Multi-teacher,Object detection
AI 理解论文
溯源树
样例
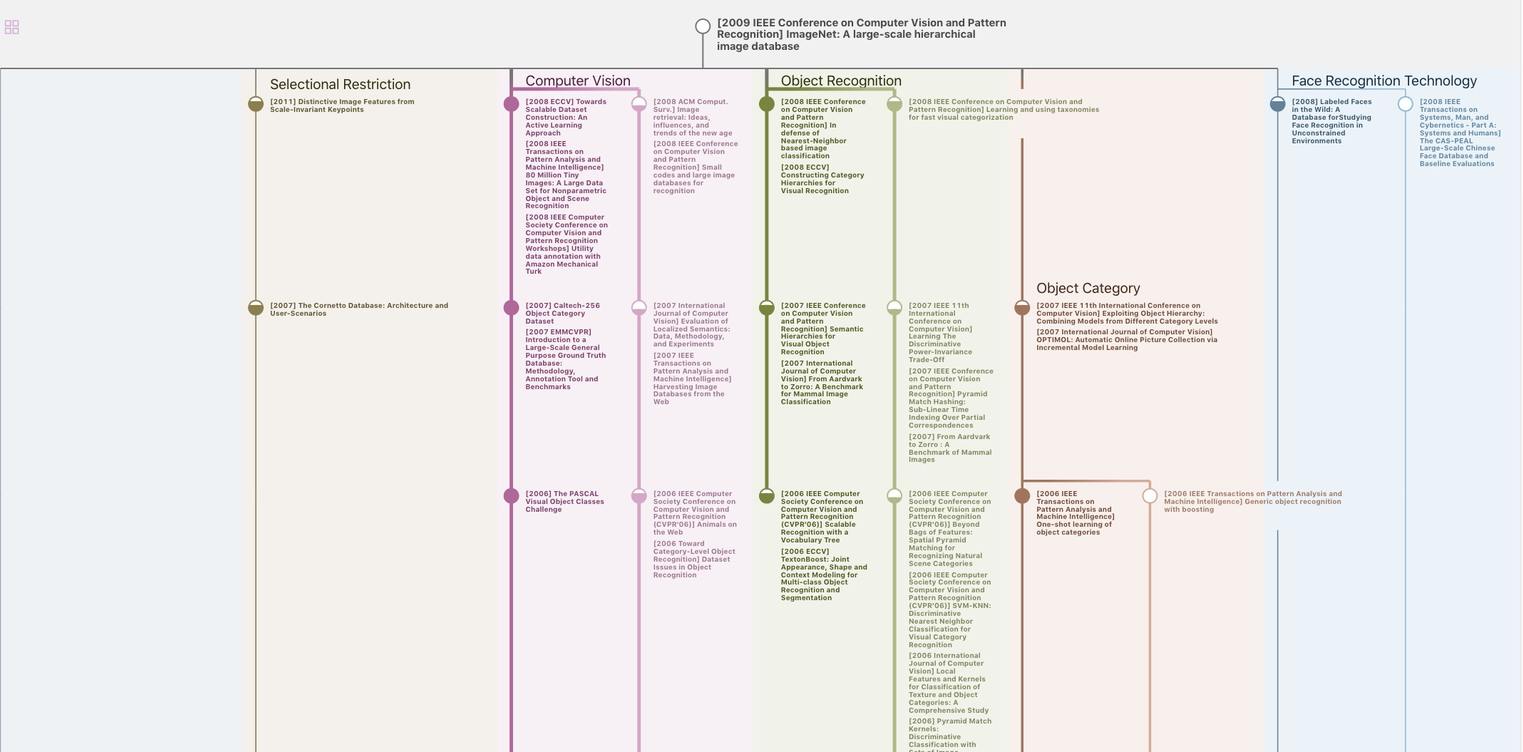
生成溯源树,研究论文发展脉络
Chat Paper
正在生成论文摘要