Confidence-Guided Open-World Semi-supervised Learning
PATTERN RECOGNITION AND COMPUTER VISION, PRCV 2023, PT IV(2024)
摘要
Open-world semi-supervised learning differs from traditional semi-supervised learning by allowing labeled and unlabeled data from different data distributions, i.e., unlabeled data may contain novel classes. The goal is to identify samples of known classes while simultaneously detecting and clustering samples of novel classes found in the unlabeled data. To address this challenging task, we propose a novel open-world semi-supervised learning model, which integrates two new confidence-guided techniques to better leverage unlabeled data, especially those from novel classes. Specifically, we first approximate the confidence of model on unlabeled samples by inspecting the prediction consistency through the training process, which guides the temperature scaling for cross-entropy loss. Then, we introduce an adaptive class-specific reweighting contrastive learning method, which further synchronizes the learning speed of known and unknown classes, while reducing the influence of noisy labels. Extensive empirical results show our approach achieves significant performance improvement in both seen and unseen classes compared with previous studies, especially in challenging scenarios with limited labeled data.
更多查看译文
关键词
open-world semi-supervised learning,confidence estimation,contrastive learning
AI 理解论文
溯源树
样例
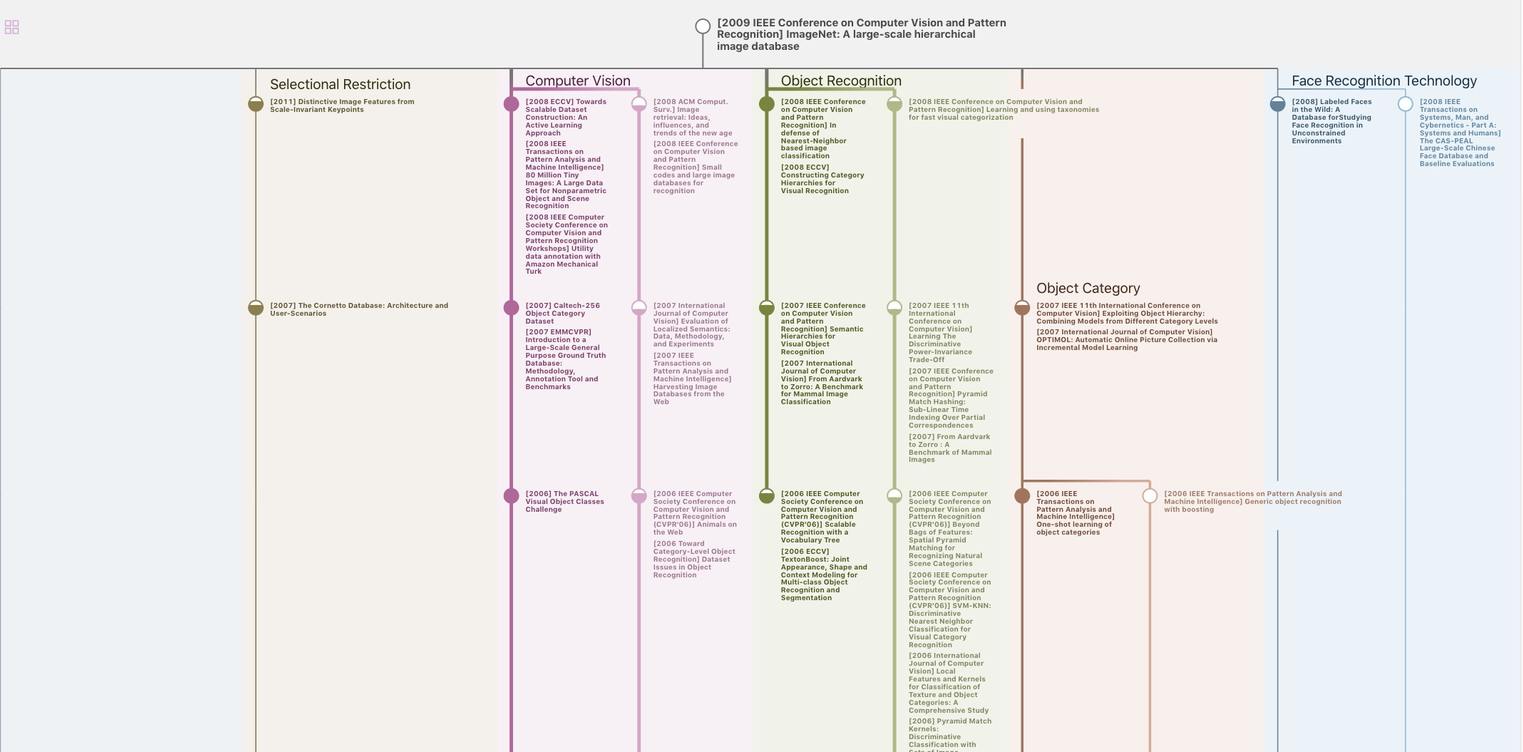
生成溯源树,研究论文发展脉络
Chat Paper
正在生成论文摘要