Nonconvex Tensor Hypergraph Learning for Multi-view Subspace Clustering
PATTERN RECOGNITION AND COMPUTER VISION, PRCV 2023, PT IV(2024)
摘要
Low-rank representation has been widely used in multi-view clustering. But the existing methods are matrix-based, which cannot well capture high-order low-rank correlation embedded in multiple views and fail to retain the local geometric structure of features resided in multiple nonlinear subspaces simultaneously. To handle this problem, we propose a nonconvex tensor hypergraph learning for multi-view subspace clustering. In this model, the hyper-Laplacian regularization is used to capture high-order global and local geometric information of all views. The nonconvex weighted tensor Schatten-p norm can better characterize the high-order correlations of multi-view data. In addition, we design an effective alternating direction algorithm to optimize this nonconvex model. Extensive experiments on five datasets prove the robustness and superiority of the proposed method.
更多查看译文
关键词
Hypergarph learning,Tensor Schatten-p norm,Low-rank representation
AI 理解论文
溯源树
样例
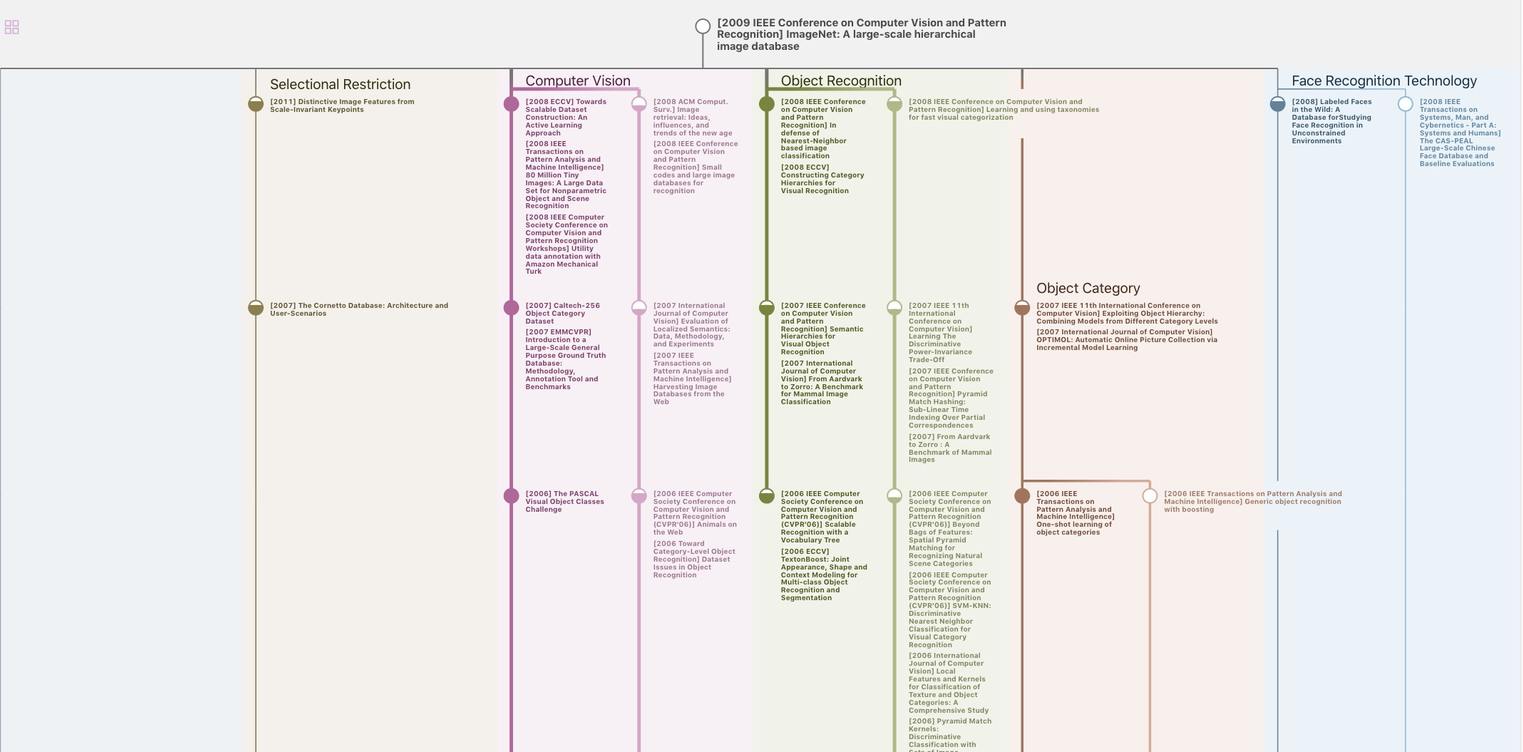
生成溯源树,研究论文发展脉络
Chat Paper
正在生成论文摘要