Multi-modal Graph and Sequence Fusion Learning for Recommendation
PATTERN RECOGNITION AND COMPUTER VISION, PRCV 2023, PT I(2024)
摘要
Multi-modal recommendation aims to leverage multi-modal information for mining users' latent preferences. Existing multi-modal recommendation approaches primarily exploit graph structures and multi-modal information to explore the graph information derived from user-item interactions, overlooking the underlying sequence information. Furthermore, by treating items solely as coarse-grained entities, the latent relationships of items within each modality are disregarded, impeding the effective extraction of latent user preferences. To address the limitations, we propose a novel approach called Multi-modal Graph and Sequence Fusion Learning Architecture for Recommendation (MMGCF). In MMGCF, we first construct dynamic item-item graphs to enhance item features and capture relationships within each modality. Subsequently, according to the influence between modalities, we design a self attention network to fuse multi-modal features. Finally, in addition to regular graph convolution, we also devise a sequence-aware learning layer to preserve and capture sequence information for model to learn user preferences from a sequential perspective. Extensive experiments conducted on three real-world datasets demonstrate the superiority of our method over various state-of-the-art baselines.
更多查看译文
关键词
Multi-modal Recommendation,Graph Neural Network,Recurrent Neural Network,Self Attention Network
AI 理解论文
溯源树
样例
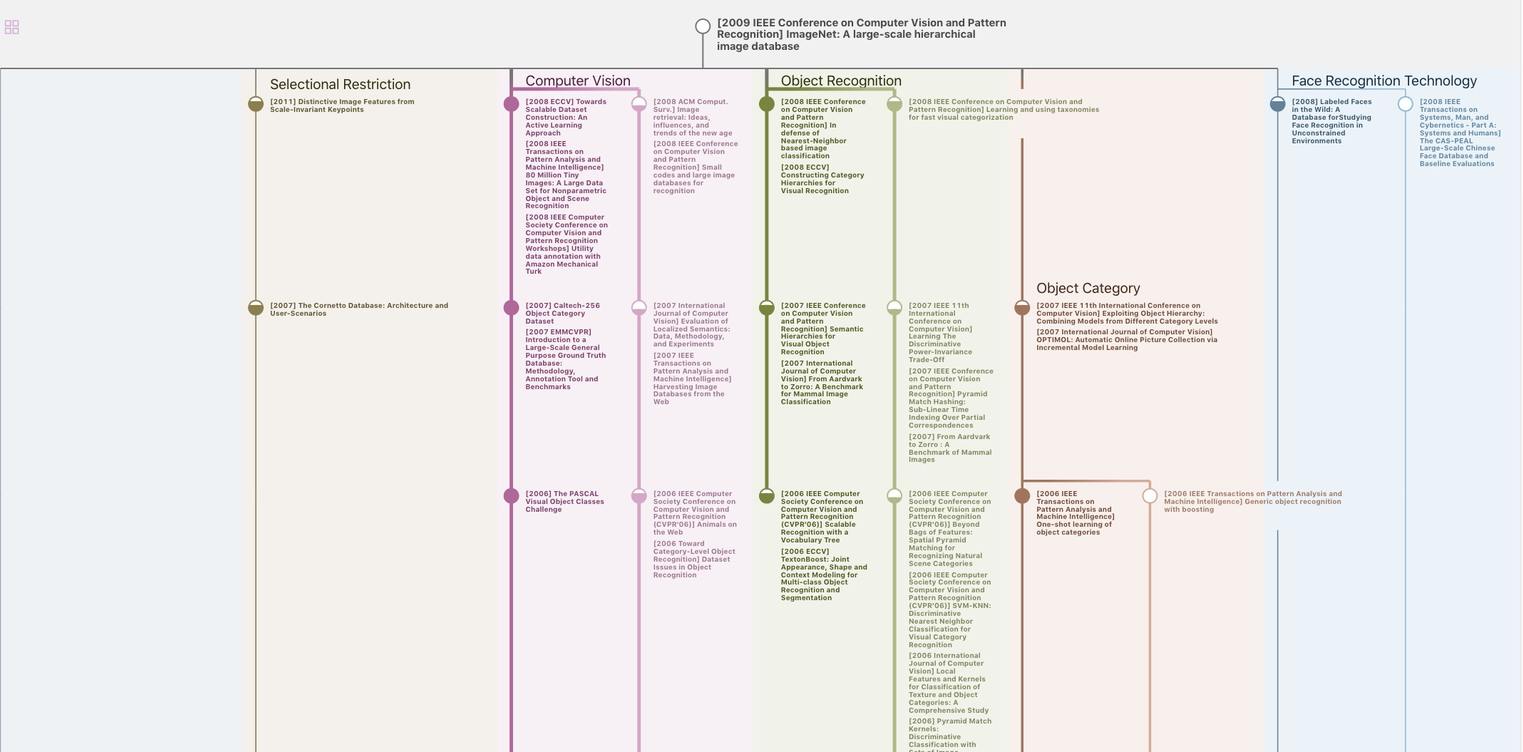
生成溯源树,研究论文发展脉络
Chat Paper
正在生成论文摘要