ScaleNet: Rethinking Feature Interaction from a Scale-Wise Perspective for Medical Image Segmentation
ADVANCES IN COMPUTER GRAPHICS, CGI 2023, PT IV(2024)
摘要
Recently, vision transformers have become outstanding segmentation structures for their remarkable global modeling capability. In current transformer-based models for medical image segmentation, convolutional layers are often replaced by transformers, or transformers are added to the deepest layer of the encoder to learn the global context. However, for the extracted multi-scale feature information, most existing methods tend to ignore the multi-scale dependencies, which leads to inadequate feature learning and fails to produce rich feature representations. In this paper, we propose ScaleNet from the perspective of feature interaction at different scales that can alleviate mentioned problems. Specifically, our approach consists of two multi-scale feature interaction modules: the spatial scale interaction (SSI) and the channel scale interaction (CSI). SSI uses a transformer to aggregate patches from different scale features to enhance the feature representations at the spatial scale. CSI uses a 1D convolutional layer and a fully connected layer to perform a global fusion of multi-level features at the channel scale. The combination of CSI and SSI enables ScaleNet to emphasize multi-scale dependencies and effectively resolve complex scale variations.
更多查看译文
关键词
medical image segmentation,transformer-based method,multi-scale feature interaction,multi-organ and skin lesion segmentation tasks
AI 理解论文
溯源树
样例
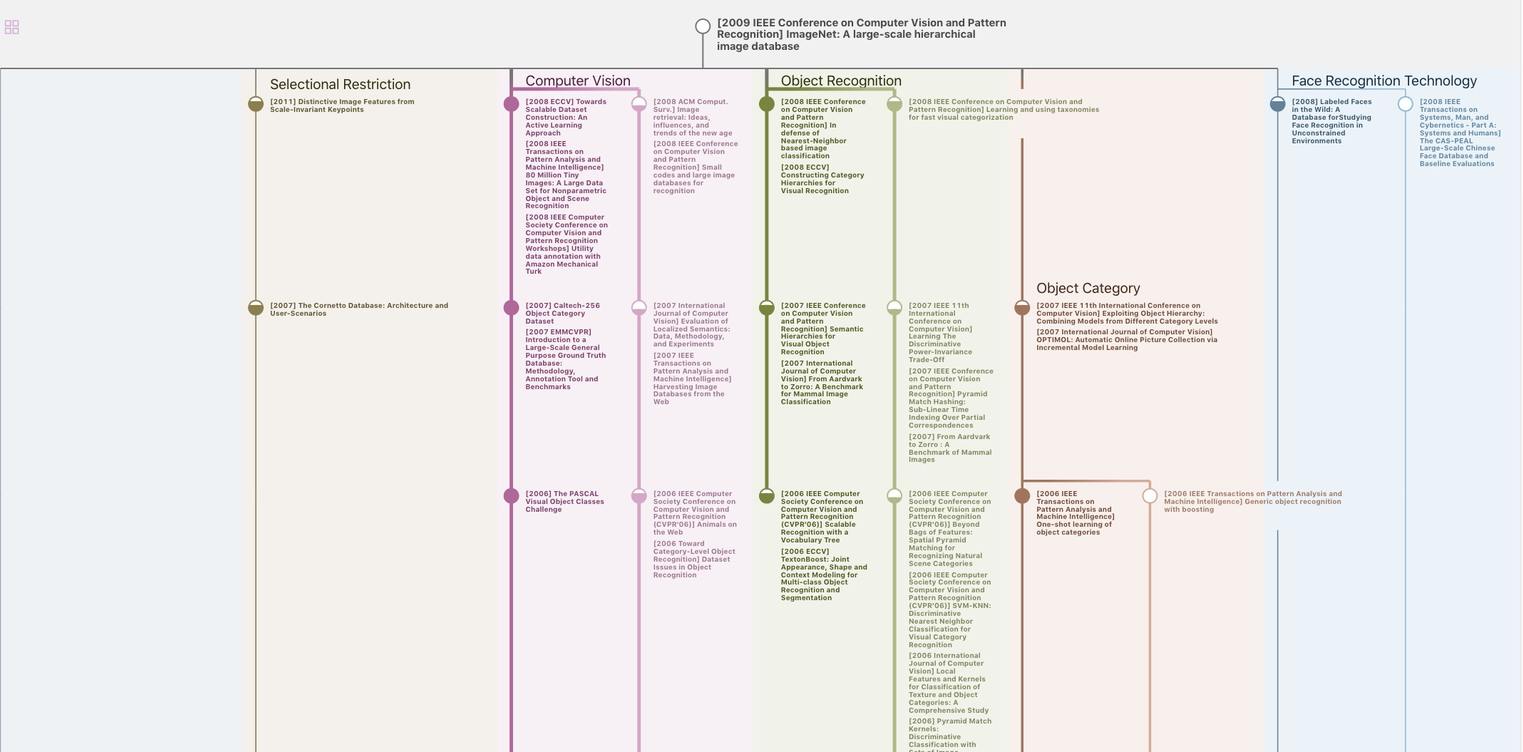
生成溯源树,研究论文发展脉络
Chat Paper
正在生成论文摘要