Exploring the potential of federated learning in mental health research: a systematic literature review
Applied Intelligence(2024)
摘要
The rapid advancement of technology has created new opportunities to improve the accuracy and efficiency of medical diagnoses, treatments, and overall patient care in several medical domains, including mental health. One promising novel approach is federated learning, a machine learning approach that allows multiple devices to train a shared model without exchanging raw data. Instead of centralizing the data in one location, each device or machine holds a portion of the data and collaborates with other devices to update the shared model. In this way, federated learning enables training on more extensive and diverse datasets than would be possible with centralized training while preserving the privacy and security of individual data. In the mental health domain, federated learning has the potential to improve mental disorders’ detection, diagnosis, and treatment. By pooling data from multiple sources while maintaining patient privacy by keeping data secure and ensuring that they are not used for unauthorized purposes. This literature survey reviews recent studies that have exploited federated learning in the psychiatric domain, covering multiple data resources and different machine-learning techniques. Furthermore, we formulate the gap in the current methodologies and propose new research directions.
更多查看译文
关键词
Federated learning,Mental illness,Psychiatry,Machine learning
AI 理解论文
溯源树
样例
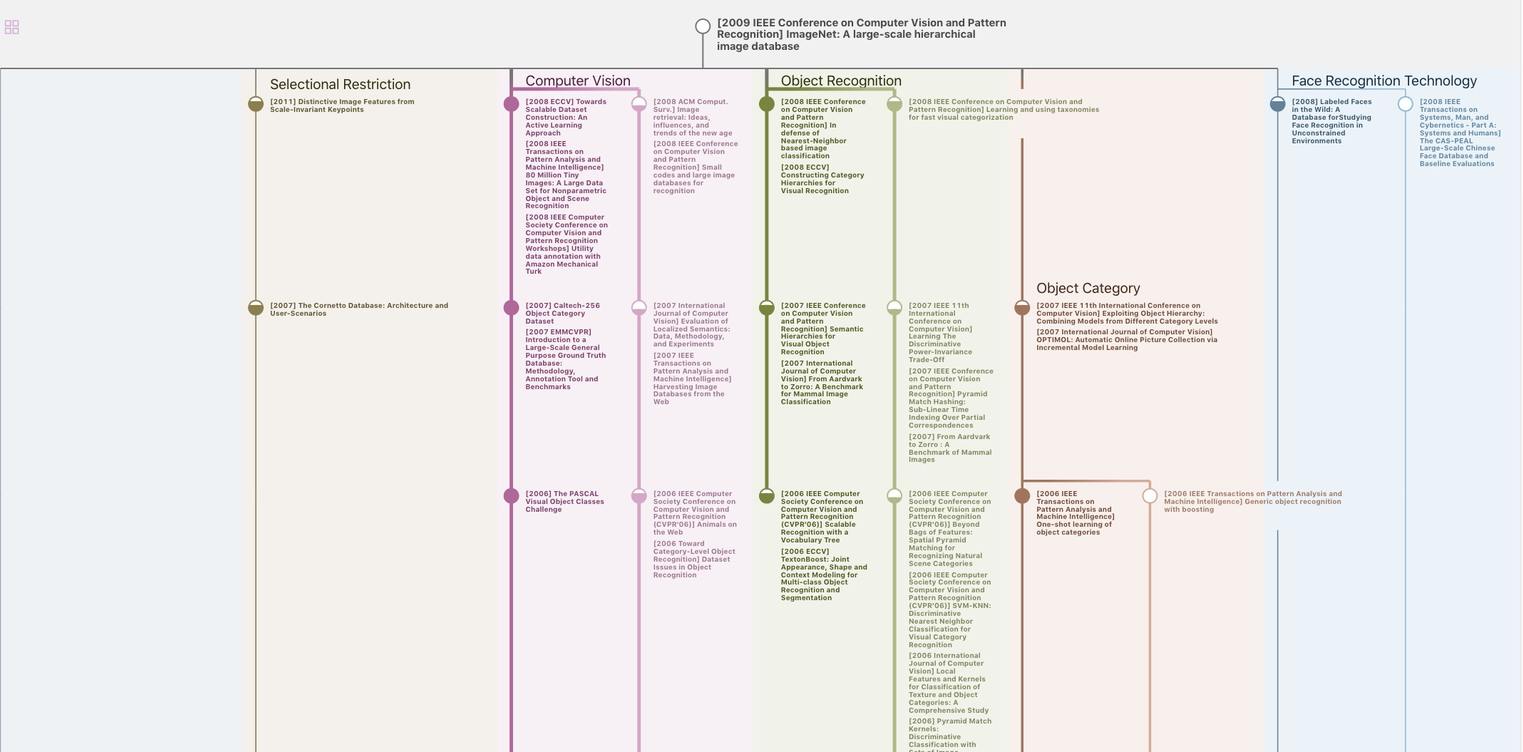
生成溯源树,研究论文发展脉络
Chat Paper
正在生成论文摘要