Correction: GAL: combining global and local contexts for interpersonal relation extraction toward document-level Chinese text
Neural Computing and Applications(2024)
摘要
Current interpersonal relation extraction toward Chinese text remains at the sentence-level, which narrows practical applications since most relational facts are implied through multiple sentences in the document. Moreover, considering huge differences between the Chinese and English languages (e.g., different ways of word segmentation and expression preferences), it is impractical to directly adopt models designed for English corpus to model Chinese implicit semantics. To address these challenges, we propose a novel model called GAL (global and local) to comprehend the Chinese context for interpersonal relation extraction at the document-level. Specifically, we devise the global and the local representation modules to encode the entity pair’s relation, perceiving related content from multi-perspectives. For the global representation module, we first design a heterogeneous graph comprised of the document node, entity nodes, and sentence nodes; then, we perform reasoning on it to obtain the global feature via R-GCN. For the local representation module, the robust hierarchical attention is conceived to capture the local semantic features from entity pair’s co-occurrence sentences. Finally, we concatenate the global and local representations for classification. Moreover, due to the lack of an open-source dataset, we employ the distant supervision method to construct the Chinese interpersonal relation extraction dataset at the document-level, DocIPRE. Various experimental results validate the better modeling ability of GAL as it achieves 54.55% on the F1 score, outperforming the state-of-the-art method by a significant margin (1.34% on F1). The source code and dataset will be available soon.
更多查看译文
关键词
Chinese interpersonal relation extraction,Distant supervision,Robust hierarchical attention,Heterogeneous graph,Graph convolutional network
AI 理解论文
溯源树
样例
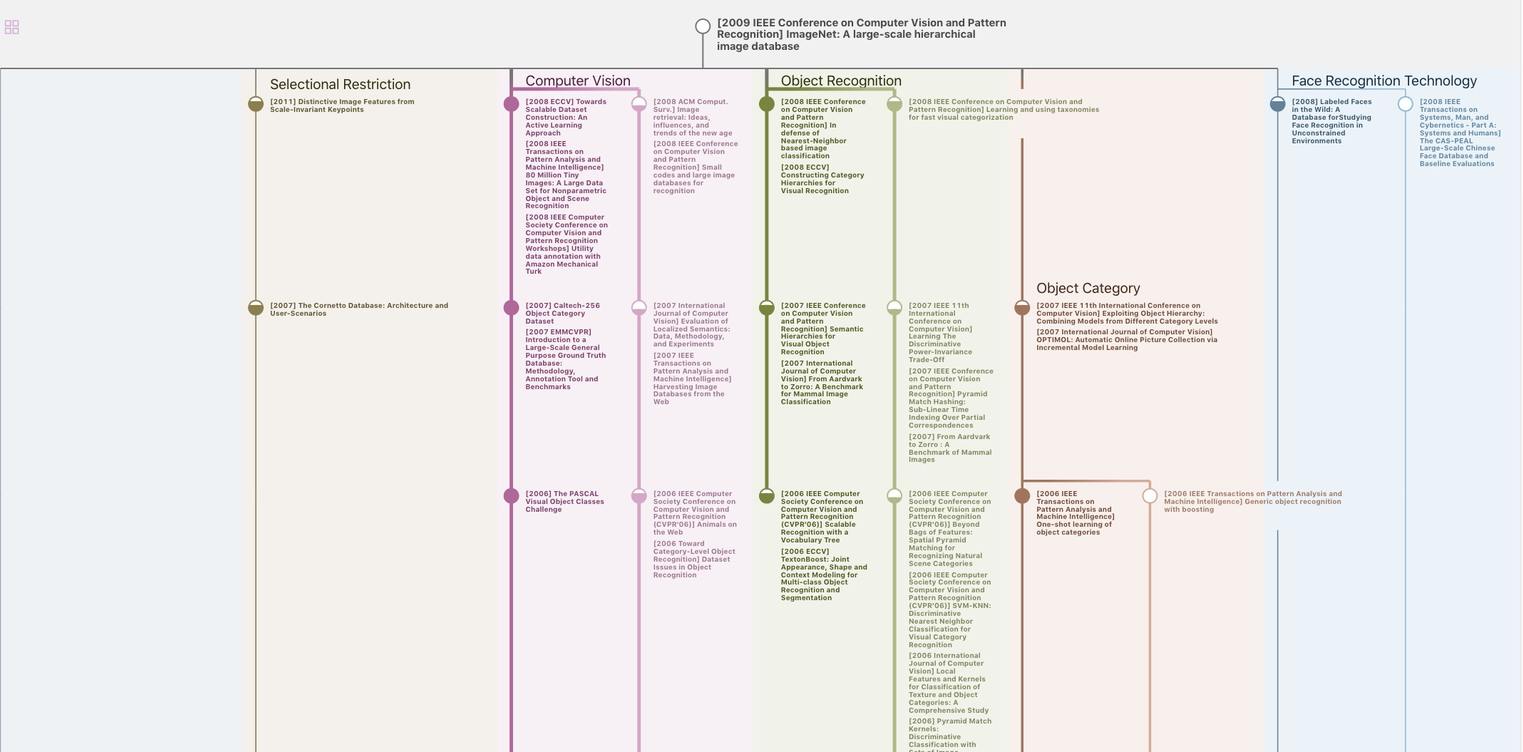
生成溯源树,研究论文发展脉络
Chat Paper
正在生成论文摘要