Modeling soil loss under rainfall events using machine learning algorithms
JOURNAL OF ENVIRONMENTAL MANAGEMENT(2024)
摘要
Soil loss is an environmental concern of global importance. Accurate simulation of soil loss in small watersheds is crucial for protecting the environment and implementing soil and water conservation measures. However, predicting soil loss while meeting the criteria of high precision, efficiency, and generalizability remains a challenge. Therefore, this study first used three machine learning (ML) algorithms, namely, random forest (RF), support vector machine (SVM), and artificial neural network (ANN) to develop soil loss models and predict soil loss rates (SLRs). These soil loss models were constructed using field observation data with an average SLR of 1756.48 t/km2 from rainfall events and small watersheds in the hilly-gully region of the Loess Plateau, China. During training, testing and generalizability stages, the average coefficients of determination from the RF, SVM, and ANN models were 0.903, 0.860, and 0.836, respectively. Similarly, the average Nash-Sutcliffe coefficients of efficiency from the RF, SVM and ANN models were 0.893, 0.791 and 0.814, respectively. These results indicated that MLs have superior predictive performance and generalizability, and broad prospects for predicting SLRs. This study also demonstrated that the RF model outperformed better than the SVM and ANN models. Therefore, the RF model was used to simulate the SLR of each small watershed in the Chabagou watershed. Our results showed the four-year (2017-2020) average annual SLR of the small watersheds ranged from 0.73 to 1.63 x 104 t/(km2 center dot a) in the Chabagou watershed. Additionally, the results also indicated the SLR of small watersheds under the rainstorm event with a 100-year recurrence interval was 4.4-51.3 times that of other rainfall events. Furthermore, this study confirmed that bare land was the predominant source of soil loss in the Chabagou watershed, followed by cropland land and grassland. This study helps to provide the theoretical basis for deploying soil and water conservation measures to realize the sustainable utilization of soil resources in the future.
更多查看译文
关键词
Soil loss model,Random forest,Support vector machine,Artificial neural network,Loess plateau
AI 理解论文
溯源树
样例
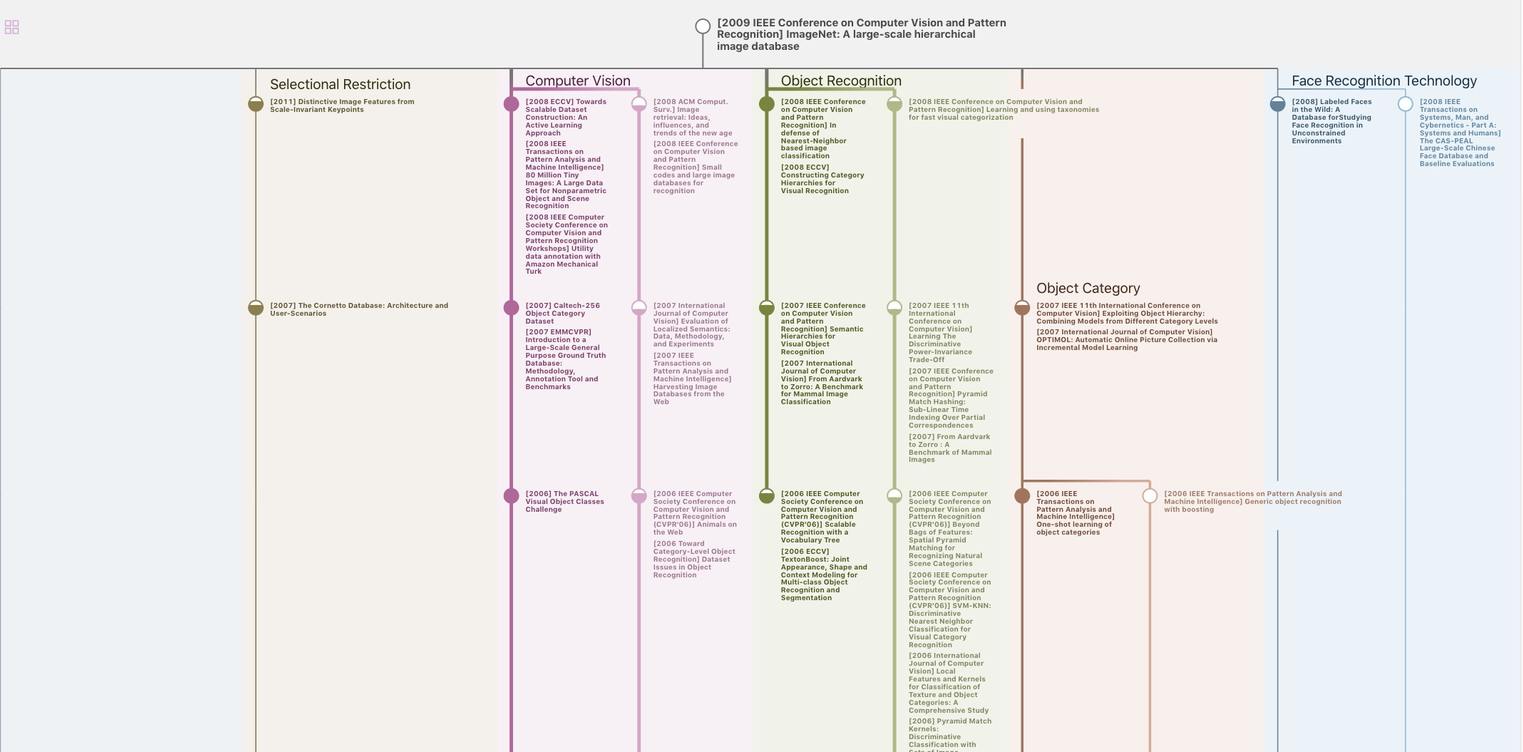
生成溯源树,研究论文发展脉络
Chat Paper
正在生成论文摘要