SIGMA leverages protein structural information to predict the pathogenicity of missense variants
CELL REPORTS METHODS(2024)
摘要
Leveraging protein structural information to evaluate pathogenicity has been hindered by the scarcity of experimentally determined 3D protein. With the aid of AlphaFold2 predictions, we developed the structure -informed genetic missense mutation assessor (SIGMA) to predict missense variant pathogenicity. In comparison with existing predictors across labeled variant datasets and experimental datasets, SIGMA demonstrates superior performance in predicting missense variant pathogenicity (AUC = 0.933). We found that the relative solvent accessibility of the mutated residue contributed greatly to the predictive ability of SIGMA. We further explored combining SIGMA with other top -tier predictors to create SIGMA+, proving highly effective for variant pathogenicity prediction (AUC = 0.966). To facilitate the application of SIGMA, we precomputed SIGMA scores for over 48 million possible missense variants across 3,454 disease -associated genes and developed an interactive online platform (https://www.sigma-pred.org/). Overall, by leveraging protein structure information, SIGMA offers an accurate structure -based approach to evaluating the pathogenicity of missense variants.
更多查看译文
AI 理解论文
溯源树
样例
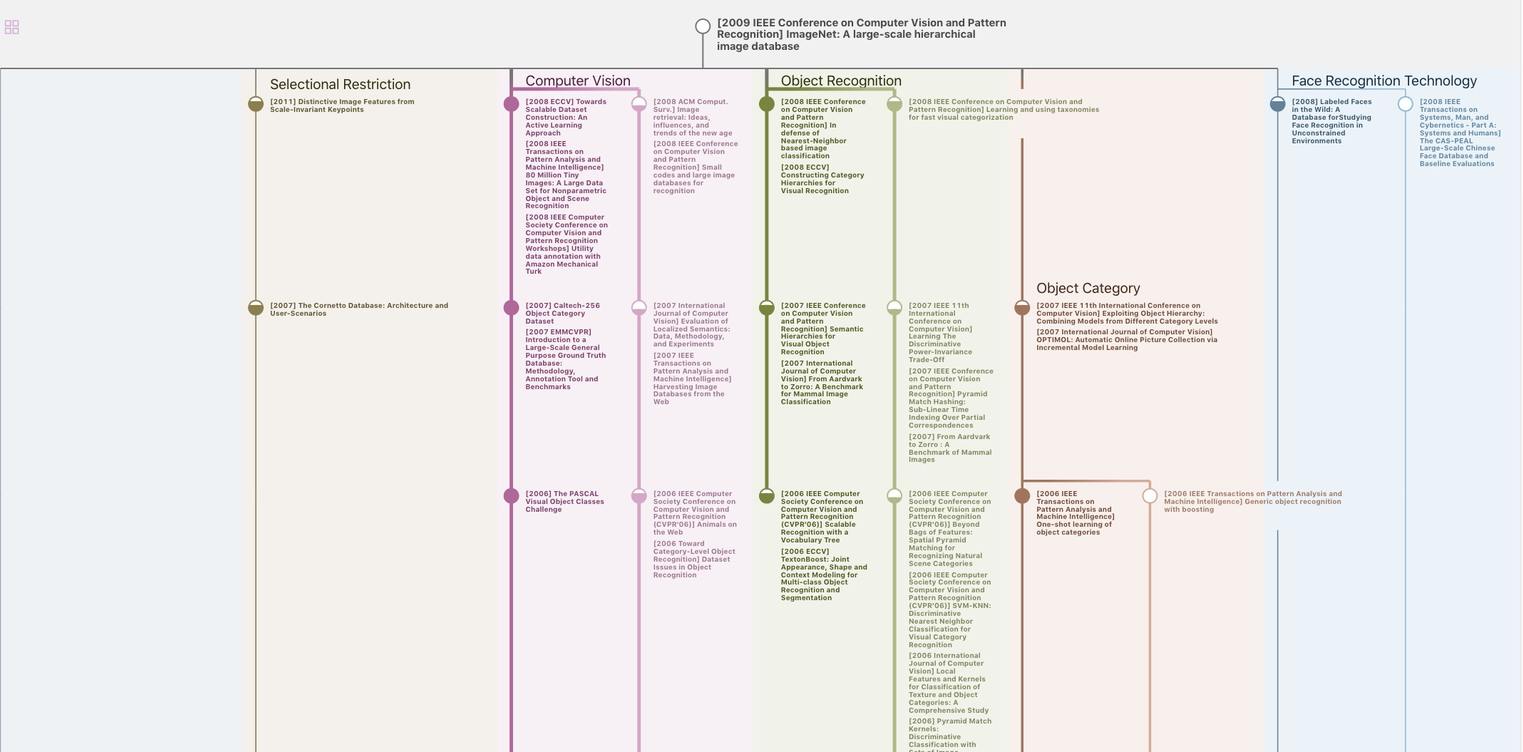
生成溯源树,研究论文发展脉络
Chat Paper
正在生成论文摘要