Sensor-Based Discovery of Search and Palpation Modes in the Clinical Breast Examination.
Academic medicine : journal of the Association of American Medical Colleges(2024)
摘要
PURPOSE:Successful implementation of precision education systems requires widespread adoption and seamless integration of new technologies with unique data streams that facilitate real-time performance feedback. This paper explores the use of sensor technology to quantify hands-on clinical skills. The goal is to shorten the learning curve through objective and actionable feedback.
METHOD:A sensor-enabled clinical breast examination (CBE) simulator was used to capture force and video data from practicing clinicians (N = 152). Force-by-time markers from the sensor data and a machine learning algorithm were used to parse physicians' CBE performance into periods of search and palpation and then these were used to investigate distinguishing characteristics of successful vs unsuccessful attempts to identify masses in CBEs.
RESULTS:Mastery performance from successful physicians showed stable levels of speed and force across the entire CBE and a 15% increase in force when in palpation mode compared to search mode. Unsuccessful physicians failed to search with sufficient force to detect deep masses F(5,146) = 4.24, P = .001. While similar proportions of male and female physicians reached the highest performance level, males used more force as noted by higher palpation to search force ratios t(63) = 2.52, P = .014.
CONCLUSIONS:Sensor technology can serve as a useful pathway to assess hands-on clinical skills and provide data-driven feedback. When using a sensor-enabled simulator, the authors found specific haptic approaches that were associated with successful CBE outcomes. Given this study's findings, continued exploration of sensor technology in support of precision education for hands-on clinical skills is warranted.
更多查看译文
AI 理解论文
溯源树
样例
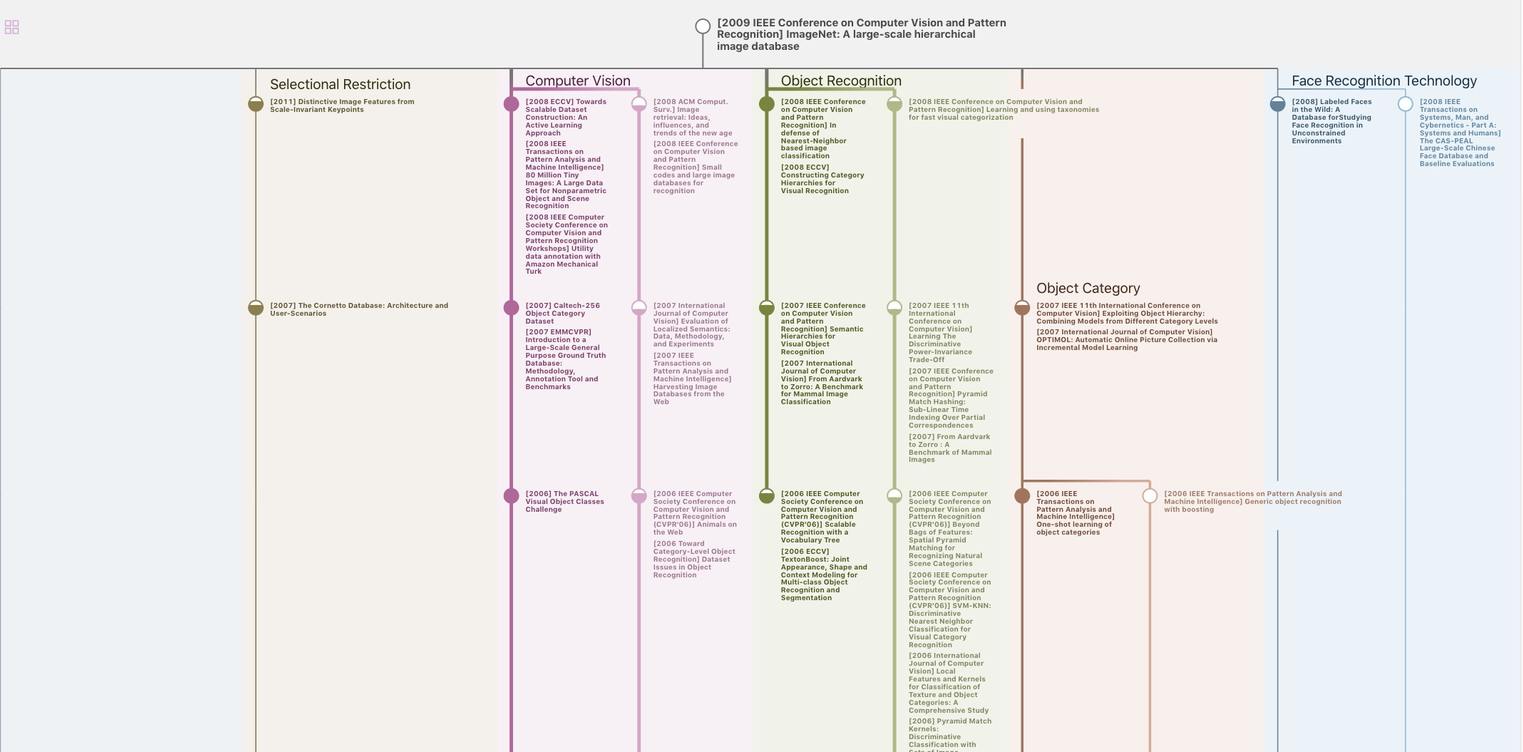
生成溯源树,研究论文发展脉络
Chat Paper
正在生成论文摘要