Graph Representation Learning for Parameter Transferability in Quantum Approximate Optimization Algorithm
arxiv(2024)
摘要
The quantum approximate optimization algorithm (QAOA) is one of the most promising candidates for achieving quantum advantage through quantum-enhanced combinatorial optimization. Optimal QAOA parameter concentration effects for special MaxCut problem instances have been observed, but a rigorous study of the subject is still lacking. Due to clustering of optimal QAOA parameters for MaxCut, successful parameter transferability between different MaxCut instances can be explained and predicted based on local properties of the graphs, including the type of subgraphs (lightcones) from which graphs are composed as well as the overall degree of nodes in the graph (parity). In this work, we apply five different graph embedding techniques to determine good donor candidates for parameter transferability, including parameter transferability between different classes of MaxCut instances. Using this technique, we effectively reduce the number of iterations required for parameter optimization, obtaining an approximate solution to the target problem with an order of magnitude speedup. This procedure also effectively removes the problem of encountering barren plateaus during the variational optimization of parameters. Additionally, our findings demonstrate that the transferred parameters maintain effectiveness when subjected to noise, supporting their use in real-world quantum applications. This work presents a framework for identifying classes of combinatorial optimization instances for which optimal donor candidates can be predicted such that QAOA can be substantially accelerated under both ideal and noisy conditions.
更多查看译文
AI 理解论文
溯源树
样例
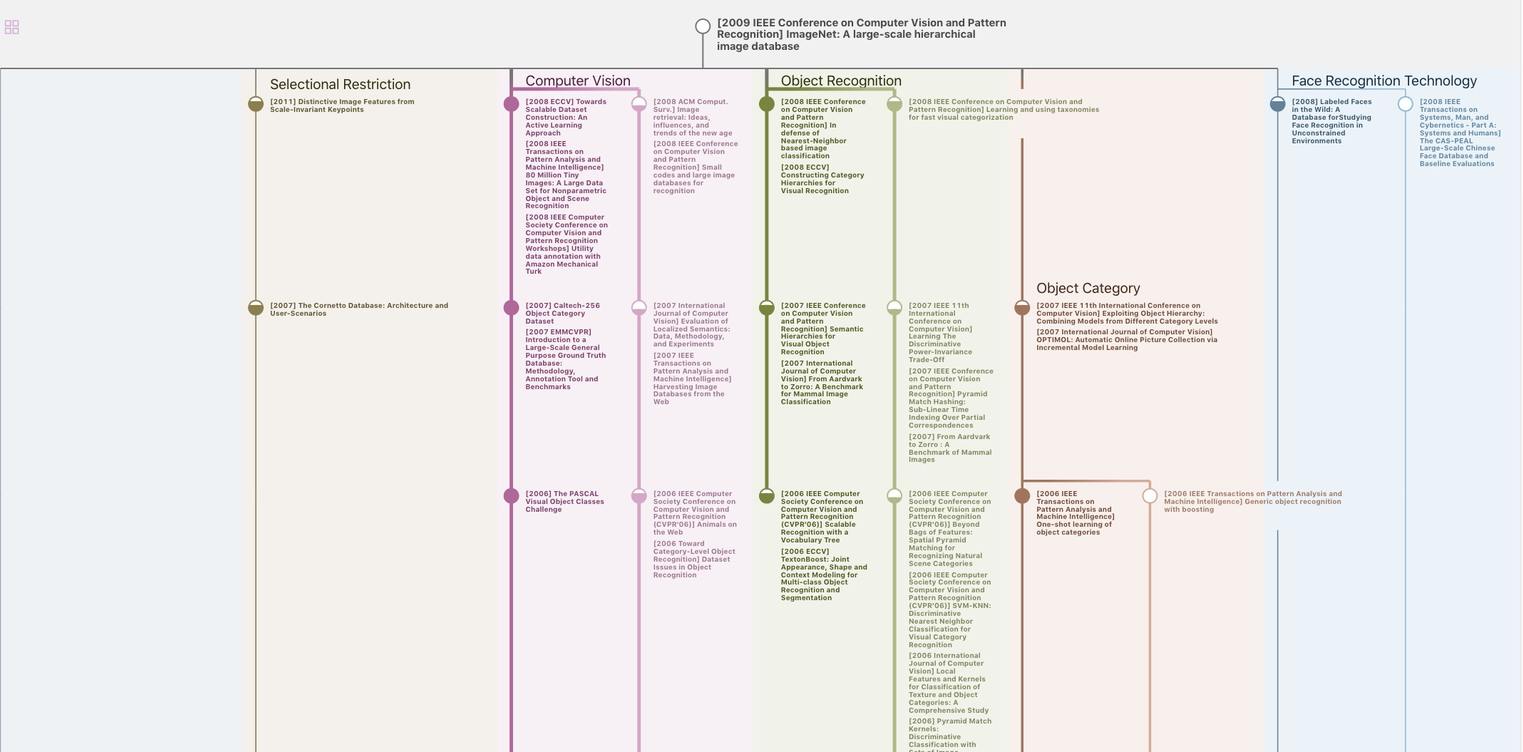
生成溯源树,研究论文发展脉络
Chat Paper
正在生成论文摘要