Robust CNN architecture for classification of reach and grasp actions from neural correlates: an edge device perspective
MEASUREMENT SCIENCE AND TECHNOLOGY(2024)
摘要
Brain-computer interfaces (BCIs) systems traditionally use machine learning (ML) algorithms that require extensive signal processing and feature extraction. Deep learning (DL)-based convolutional neural networks (CNNs) recently achieved state-of-the-art electroencephalogram (EEG) signal classification accuracy. CNN models are complex and computationally intensive, making them difficult to port to edge devices for mobile and efficient BCI systems. For addressing the problem, a lightweight CNN architecture for efficient EEG signal classification is proposed. In the proposed model, a combination of a convolution layer for spatial feature extraction from the signal and a separable convolution layer to extract spatial features from each channel. For evaluation, the performance of the proposed model along with the other three models from the literature referred to as EEGNet, DeepConvNet, and EffNet on two different embedded devices, the Nvidia Jetson Xavier NX and Jetson Nano. The results of the Multivariant 2-way ANOVA (MANOVA) show a significant difference between the accuracies of ML and the proposed model. In a comparison of DL models, the proposed models, EEGNet, DeepConvNet, and EffNet, achieved 92.44 +/- 4.30, 90.76 +/- 4.06, 92.89 +/- 4.23, and 81.69 +/- 4.22 average accuracy with standard deviation, respectively. In terms of inference time, the proposed model performs better as compared to other models on both the Nvidia Jetson Xavier NX and Jetson Nano, achieving 1.9 sec and 16.1 sec, respectively. In the case of power consumption, the proposed model shows significant values on MANOVA (p < 0.05) on Jetson Nano and Xavier. Results show that the proposed model provides improved classification results with less power consumption and inference time on embedded platforms.
更多查看译文
关键词
brain computer interface,deep learning,convolutional neural networks,embedded edge devices
AI 理解论文
溯源树
样例
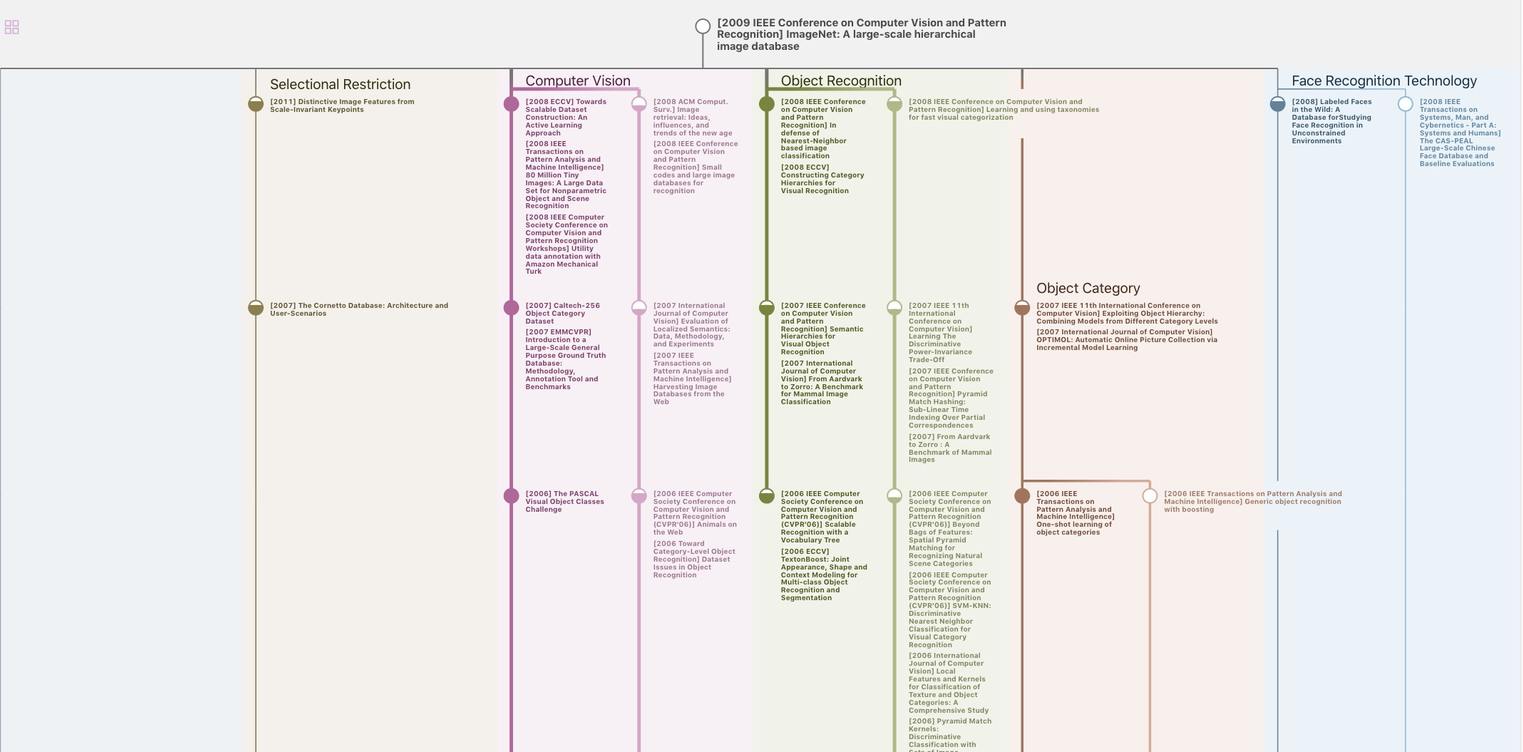
生成溯源树,研究论文发展脉络
Chat Paper
正在生成论文摘要