IPOCIM: Artificial Intelligent Architecture Design Space Exploration With Scalable Ping-Pong Computing-in-Memory Macro
IEEE TRANSACTIONS ON VERY LARGE SCALE INTEGRATION (VLSI) SYSTEMS(2024)
摘要
Computing-in-memory (CIM) architecture has become a possible solution to designing an energy-efficient artificial intelligent processor. Various CIM demonstrators indicated the computing efficiency of CIM macro and CIM-based processors. However, previous studies mainly focus on macro optimization and low CIM capacity without considering the weight update strategy of CIM architecture. The artificial intelligence (AI) processor with a CIM engine practically induces issues, including updating memory data and supporting different operators. For instance, AI-oriented applications usually contain various weight parameters. The weight stored in the CIM architecture should be reloaded for the considerable gap between the capacity of CIM and growing weight parameters. The computation efficiency of the CIM architecture is reduced by the weight updating and waiting. In addition, the natural parallelism of CIM leads to the mismatch of various convolution kernel sizes in different networks and layers, which reduces hardware utilization efficiency. In this work, we develop a CIM engine with a ping-pong computing strategy as an alternative to typical CIM macro and weight buffer, hiding the data update latency and improving the data reuse ratio. Based on the pingpong engine, we propose a flexible CIM architecture adapting to different sizes of neural networks, namely, intelligent pong computing-in memory (IPOCIM), with a fine-grained data flow mapping strategy. Based on the evaluation, IPOCIM can achieve a 1.27-6.27x performance and 2.34-5.30x energy efficiency improvement compared to the state-of-the-art works.
更多查看译文
关键词
Common Information Model (computing),Computer architecture,Artificial intelligence,Energy efficiency,Neural networks,Convolution,Memory management,Artificial intelligent processor,computing-in-memory (CIM),energy efficiency,neural networks,ping-pong computing
AI 理解论文
溯源树
样例
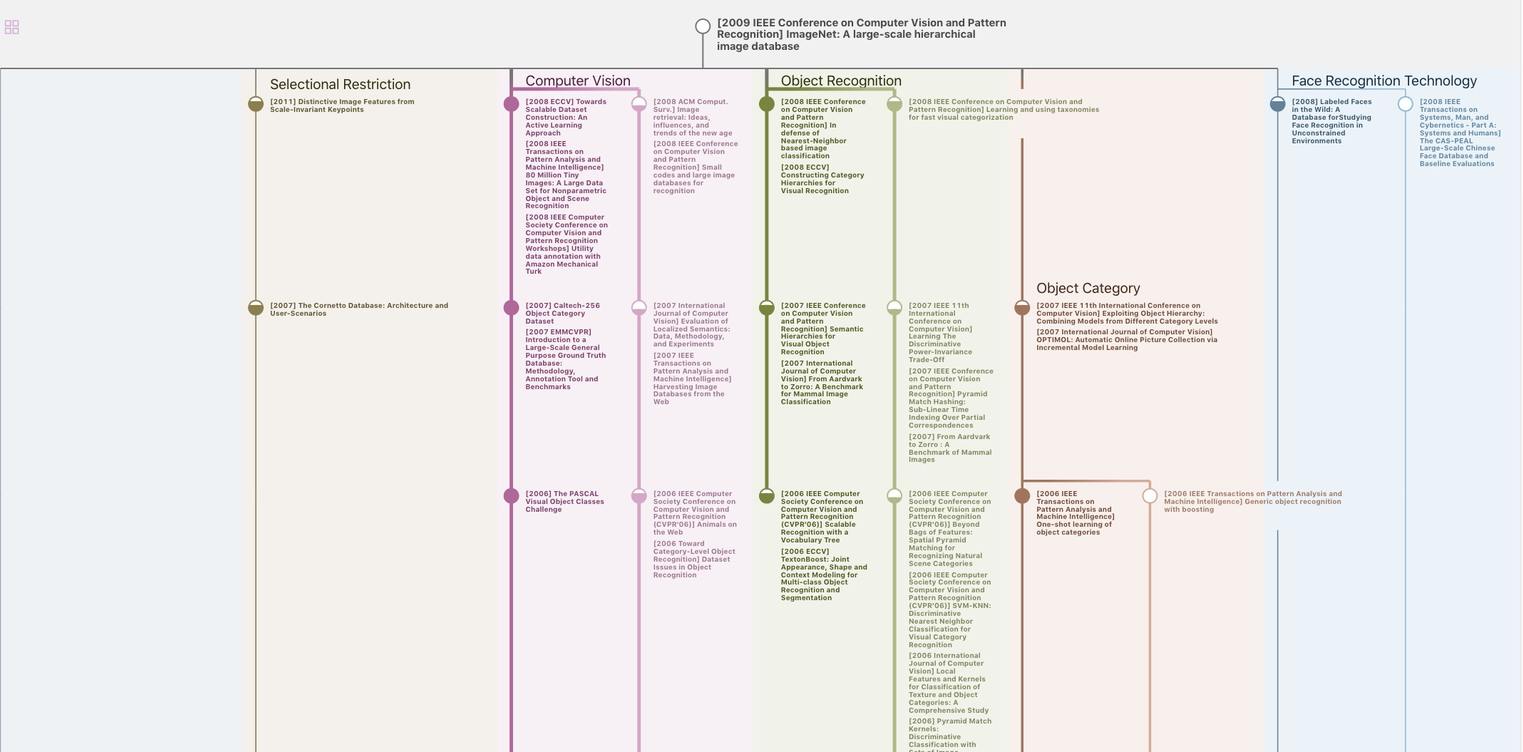
生成溯源树,研究论文发展脉络
Chat Paper
正在生成论文摘要